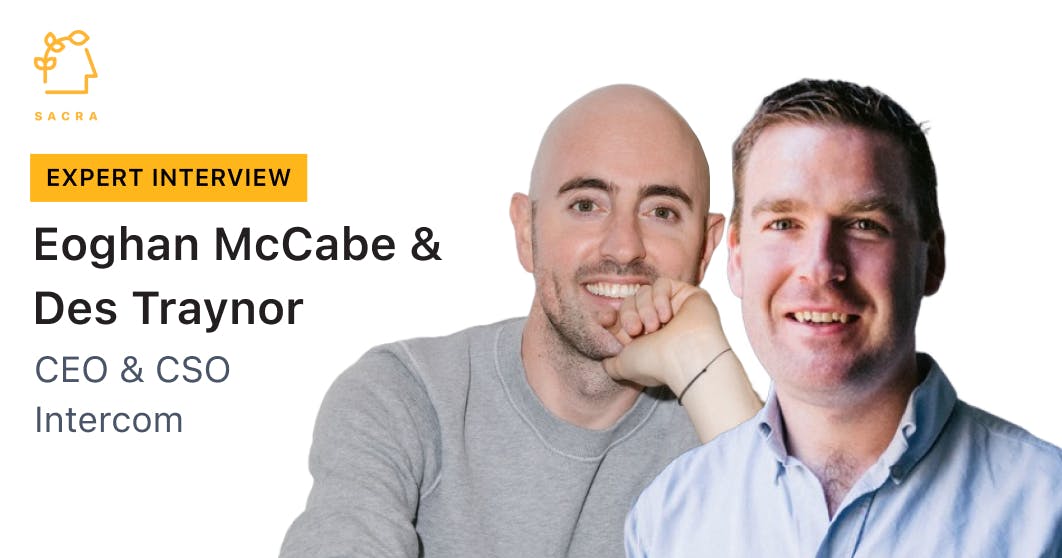
Background
Eoghan McCabe and Des Traynor are co-founders and today, the CEO and CSO of Intercom. We talked to Eoghan and Des about the three generations of customer service chatbots, how AI is transforming customer service, and what the future of the customer support/success function looks like in a world of support-trained LLMs.
Questions
- Intercom has been positioning pretty hard on AI, and it's interesting to see that high-level conviction around it. How important is it that Intercom became founder-led again to make this big push around AI? Eoghan, we'd love to hear about your return to the CEO role over the last 8 months and how the AI focus came about.
- Let’s dive into Fin, your AI bot for customer service. Can you go deep into what the core problem is for customers and how AI is the solution to that?
- Do you intend to really, really focus on automated responses that improve the speed of response, or do you intend to also offer an option with a human in the loop? And how do you win the trust of customers so that they feel confident having Fin responding automatically on the site directly to customers?
- Fin was built on GPT-4. Can you tell us about the process of building Fin—key learnings around hallucinations and the shortcomings of GPT-3.5?
- Per what you said, are data, feedback loops, and SaaS the three main components of building an AI application? Do any of these take real major precedent? Is the data component by far the most important? How do you think about that?
- Can you talk about the go-to-market strategy around Fin—keeping it in beta, in waitlist, to build anticipation and at the same time be able to fine-tune it behind the scenes?
- Is percent resolution the main metric you use to evaluate product market fit? How do you decide that you're basically good to go?
- Philosophically, we've only been talking about OpenAI, GPT-3 and 3.5 and 4, ChatGPT. Did you look at Anthropic, Bard, or others? Do you have an eventual trajectory to give yourself optionality around the different LLMs versus being an OpenAI shop or an OpenAI partner? How have you thought about that?
- Can you talk about the previous era of chatbots and what worked, what didn't work, and how does that set us up for the current moment that we're in?
- How does customer service change in a world where 80% of queries are automatically answered by AI? What does the team construction look like? How are you imagining that?
- One of the things that's mixed into this question is Intercom's business model—pricing per seat SaaS—and how if customer service teams shrink, that’s something where you're disrupting your own model. Do you anticipate having usage-based models based on tokens? Is that something that’s going to be part of every AI-powered SaaS company's business model? How do you think about the pricing part?
- Can you talk about interoperability versus vertical integration? Are there benefits for having your help docs and your chatbot both powered by Intercom?
- How do you think about how AI enables Intercom to go up against an incumbent like Zendesk on customer service? What can Intercom do that Zendesk can't? How do you think about the positioning against an Intercom?
- Is building a customer data platform (CDP) important when we talk about bringing more user data in so that the AI can be powered off of and personalized based on user data?
- Is there a plan for integrating into the customer’s actual database to extract that information? How do you think that that information makes its way into the AI bot?
- How are you thinking about marketing automation today? Is it essentially some form of proactive customer support? Have you played around with AI applications, in those use cases, and are there any promising results?
- What are the core differences between the support use case with LLMs vs. LLMs with “marketing automation”?
- How has Intercom’s pricing evolved in the last eight months since Eoghan's return? What are the plans for pricing, and how do you use that competitively to avoid the bottom-up disruption from many folks who are building for AI customers?
- If everything goes right for Intercom over the next five years, what does it become and how has the world changed?
Interview
Intercom has been positioning pretty hard on AI, and it's interesting to see that high-level conviction around it. How important is it that Intercom became founder-led again to make this big push around AI? Eoghan, we'd love to hear about your return to the CEO role over the last 8 months and how the AI focus came about.
Eoghan McCabe: I don't want to oversell my own individual importance beyond what’s appropriate.
The reality is that Intercom has had an ML team for six years and we have some really outstanding leaders in our R&D organization, and what we’re doing in AI started from the bottom-up. The way Fin went down specifically was that Des excitedly texted me on the weekend to say, “Hey, the team have built this AI bot that’s actually amazing.”
That said, between the amount of capital we're putting towards this bet and the trade-offs we're making, it’s a very big decision, and it’s been a founder-oriented decision.
We have spent a lot of time and attention trying to tease apart the strategic implications of AI for customer service, which led us to believe that not only was betting on it like this a giant opportunity, but not betting on it was a serious risk.
So, to answer your actual question, it takes risk-taking energy. What is the thing that propels the founder? Is it their excitement to do new, cool, sexy stuff, or is it their willingness to take a risk that typical operators might not? Those things actually are beautifully joined and that's what creates great technology companies as far as I'm concerned.
Des Traynor: You have to move with the uncertainty. AI is just evolving too fast.
There's no opportunity to wait and see. There's no opportunity to run tests, to play it slow, to be cautious. You need to be willing to move on intuition. You need to be willing to make, as Eoghan said, a large bet based on belief, based on backing yourself, based on backing your intuition. That, to me, is what I experience from Eoghan.
Let’s dive into Fin, your AI bot for customer service. Can you go deep into what the core problem is for customers and how AI is the solution to that?
Eoghan: Fundamentally here's our perception of the customer service market, which is 100% our focus.
The standards for customer service online—and all businesses are going online, so let's just say the standard for customer service—is terrible.
Imagine if you were in a coffee shop or a car dealership and you asked a question and they said, “We're going to get back to you on Wednesday.” That’s the standard for customer service online today, and we're all so used to it that we don't question it.
Similarly, there were very few people in 2011 saying, “I should be able to see when the taxi is coming on my phone, order the taxi without ever talking to anyone, and be able to choose the quality level of my car,” No one was making these complaints—before Uber arrived.
That’s our belief about the market today and the problem that we're solving. Intercom set out 12 years ago to “make internet business personal”.
We certainly made a dent vis-a-vis our introduction of messengers and more personal ways to chat, which made it more personal, but we never really delivered great personal customer service because heretofore it has been not quite possible. You're limited by the physics of time and human energy and by the economics involved in being able to afford enough people to respond to everyone immediately.
The problem that great AI solves for customer service is that it can actually give great instant answers. It can actually dramatically reduce these down times. It can actually give the customer the thing they want. What we're going to see is consumers actually wanting to talk to AI bots.
We’re going to see people go from, “Goddammit, just get me to a human,” to “Give me your chatbot so I can ask all my little questions whenever I want, I don't want to have to do any niceties with a human.”
It’s hilarious to see people writing messages of thanks to Fin, but that is the basic problem it solves, and it's a total game changer for businesses, because long response times create huge drop-offs in funnels—people just don't come back.
Des: I would say the single biggest contribution I expect AI-generated customer support to make here is to the physics of support. Today, you take your number of inbound conversations times the average handling time on each one divided by your number of reps, and that equals roughly how quickly you can get back to people.
That's a formula that it's hard to get away from, and it means that when your customers are not really high LTV, it becomes hard to staff a massive support team.
One part of the problem is that there's so much repetitive and transactional undifferentiated heavy lifting that happens in the world of customer support. That weighs down the effectiveness and the value add of the customer support team quite a bit.
If you can imagine some sort of Maslow's hierarchy of great support, the bare minimum is to get back to your customers. A lot of businesses struggle to do that.
After that, it's to get back to your customers fast. Then it's to get back to them with the right answer fast. Somewhere up at the top it’s about getting ahead of the problems and being proactive.
What I believe Fin will do is let people climb that hierarchy pretty fast. People will get fast, accurate, tailored answers, and that'll be like with zero human involvement. That's going to be a game changer.
Do you intend to really, really focus on automated responses that improve the speed of response, or do you intend to also offer an option with a human in the loop? And how do you win the trust of customers so that they feel confident having Fin responding automatically on the site directly to customers?
Des: We are going to do both. One of the things we do is called agent assist, which is where Fin will suggest an answer to the agent that's basically good to go. The agent can tailor it, or if it's wrong, they can retrain Fin to better understand what should have been said.
I think this will speed up the productivity of agents. We had a release in January of an inbox GPT feature—summarization of conversations or expansion of replies, and that kind of thing. That's the beginning of a new type of AI-powered help desk built on agent assist, augmenting the agent, co-pilot for support, and all of that sort of stuff—so it's definitely coming.
There are two other things I'd say.
One is that we have a product in the market which lets you do customized answers. The product is called Resolution Bot. If there are specific answers you deeply care about, you can splice questions matching that out and say, “Don't use generative AI for questions like this—here’s the right answer.”
The last point I'd make is that if you think about this formula of support—the number of inbound volume times the average handling time and so on—you can speed up the agent, which I consider to be tending to the beast, or you can remove the question entirely, and I consider that to be taming the beast.
It’s a much bigger prize when you can get Intercom to actually remove the conversation from the inbox entirely. Even if you can speed up the agent by 10x, you're still locked into the proportion of inbound volume. If you can remove the conversation entirely, it takes it from 10 seconds per answer to zero. That is a step change in terms of support team productivity.
Fin was built on GPT-4. Can you tell us about the process of building Fin—key learnings around hallucinations and the shortcomings of GPT-3.5?
Des: We've had a great relationship and been partners with OpenAI for quite a while. In fact, we've been building against these large language models for a while.
We built a chatbot with GPT-3, and we observed it, and what we saw was that it had hallucinations. The danger of a hallucination in customer support is that the bot spits out a reply, the reply is wrong, but the user doesn't know the reply is wrong—and the business never even knows the conversation happened. It’s dark matter, and it’s dangerous, and that’s why we care so much about it from a trust, safety and accuracy perspective.
With GPT-3.5 Turbo, the Chat GPT release, we saw a step change. We crossed a perceptual cliff where it was like, “Hey, it looks like we can really contain this,” but there were still leaks. You could still get it to speak to things that it didn't actually have expertise in. We have, obviously, a torture test of queries where we can try to force a model to hallucinate or go off script—because even outside of hallucination there are things you don't want your support bot expressing, like political opinions or even just talking about the weather.
Controlling chatbots, how they engage and when they choose to not engage all became a lot more possible, and ultimately crossed a threshold where we know we can do it.
We can build a chatbot that will give you a straight answer if it knows the right answer with confidence. If it thinks it knows, but it's not totally sure, it'll say, “I think the answer is blah blah blah, and I read this article to get that, what do you think?” It can suggest its own uncertainty, which is really valuable because it encourages the reader to not just take the answer at face value.
Lastly and most importantly, one of the more difficult challenges is where if a chatbot doesn’t know, it can honestly say so and then hand the conversation over to a human. One of our primary beliefs about the future of customer support is that it is AI plus humans, and as a result, we spend a lot of time designing the ability to do that hand over.
We’ve gone through a period where AI was clearly not good enough. Then, we saw it improve to the point that there was definitely something there. Today, with GPT-4, we're believe that we can definitely go with this—and we think we can well clear the expectations of our customers who have high thresholds for the quality of our product.
Eoghan: When I think about the last two months and the roadmap going forward is, my thought is this: everyone can build a wrapper around GPT, but we're learning—and we knew, but now because we've got it in-market with customers we're really learning—that done well, this will be a very big product.
There's functionality around, like Des said, for your written answers, for very important questions. There's certain actions that you might want the bot to take. There's a lot of software to build there. There's all the orchestration—which customers does the bot talk to and when. There's segmentation around certain topics for certain customers. There's rules around it accessing private data that it can't actually share sources to, versus accessing a public knowledge base where it can link back. There's mechanics around custom user attributes, where if a user asks a business about the status of their order, it needs to look at user information, not just a knowledge base. There's all of the deep reporting we've been building and we will build. It goes on and on and on and on.
As this market evolves, you’re going to see a divide between companies that build an AI bot platform and then companies that build a GPT wrapper.
Per what you said, are data, feedback loops, and SaaS the three main components of building an AI application? Do any of these take real major precedent? Is the data component by far the most important? How do you think about that?
Eoghan: I think our big play, and our big bet, is that the best AI bots are going to be connected to your entire customer service platform. They're going to have all your customer information, and they're going to have all the conversational information, and they can create seamless experiences for the end user and the humans using this system, too.
We're not going to replace humans. It's going to be big teams of customer support agents teaching bots, answering questions that the bots can't answer, and actually providing customer connections that the bots can't do either.
The big thing for us, rather than just bucketing it in something like data, is actually interplay and interconnection with this end-to-end platform. There'll be a bunch of people that build AI bots, then you got someone like Zendesk who has the help desk. There's no one else out there that has an AI bot and human customer service platform.
Des: I'd offer that in terms of software, it's a long road map. There’s tone of voice customization, there's customization based on a user’s subscription tier, and others. There's a long tail of stuff, including reporting—I would say there's a classic SaaS product to be built around this whole thing that we're hard at work at. We've got a lot of it built, and there’s a lot more to come.
Can you talk about the go-to-market strategy around Fin—keeping it in beta, in waitlist, to build anticipation and at the same time be able to fine-tune it behind the scenes?
Eoghan: The marketing benefits of the waitlist were actually the least important component of the waitlist.
When a late-stage company with a lot of attention and tens of thousands of paying customers is developing a new technology, particularly one in a space that's moving as fast as AI, you have to deliberately do a bunch of things that startups just have no choice but to experience.
When you're a tiny startup with none of this kind of captive audience, you don't need a waitlist because no one knows about you. You have to beg, borrow and steal one person to try your product out. In doing so, you get the time and space to slowly develop the product with the market and with the customer, get feedback, figure out what's working, and make adjustments.
We didn't have the capacity with OpenAI, but also we just hadn't built up a full product or figured out how much we wanted to sell it. We would not have been ready to just unleash it to the market on day one (Ed: Fin is live as of 6/13). So it was actually an artificial constraint we added to give us space to develop this. But, of course, these constraints create other types of benefits and the waitlist thing allowed us to give it to certain special customers and do a little bit of the GTM stuff. But it was not actually the biggest part.
Des: There’s a bit of “nail it before we scale it” as well. We know what we're trying to build and we know who it should work for, and we’re working on getting it humming for folks who have existing docs—like 30%, 40%, 50%, 60%, up to 75-78% resolutions.
Then it’s like, “Okay, well, how can we expand?” We built things to help people bring their own help center, where they could import their docs from any public URL—Notion, Zendesk, whatever. That opened the floodgates a little bit more.
Then we've also been adding features that the customers have been requesting along the way. It's really just classic startup work—you find your ICP, make it work great for them, expand your ICP, make it work great again, expand your ICP, and so on, and that's what we're doing.
Is percent resolution the main metric you use to evaluate product market fit? How do you decide that you're basically good to go?
Eoghan: The main metrics we use for product-market fit are more around customer purchase and adoption—”Can we get people to try and use and buy this?”
Resolutions are like a north star metric. Resolving peoples’ inbound support questions with Fin is a way to measure how much value we're delivering. In terms of how well that fits into the market, that’s determined by how well the market buys it. That's more than just tech—it’s also pricing, for example.
Philosophically, we've only been talking about OpenAI, GPT-3 and 3.5 and 4, ChatGPT. Did you look at Anthropic, Bard, or others? Do you have an eventual trajectory to give yourself optionality around the different LLMs versus being an OpenAI shop or an OpenAI partner? How have you thought about that?
Des: Right now, OpenAI seems to be leading the way. We’re partnered with them and we're delivering a great experience for customers based on their tech. I’m sure there are other fantastic LLMs, and we do look at them—as you mentioned, Anthropic, Bard, Cohere, and open source ones. We fully expect that there will be a lot more to come and this space will expand and grow.
Right now, we're still trying to bring a product to market, so it feels like a premature optimization to try and nail the perfect LLM. The important thing, for us, or for anyone in this space, is to not be so heavily coupled that you can't ever try out anything else. We believe OpenAI is the best partner for us today, but we have to obviously be aware of the market evolving.
Eoghan: There will probably be different models for different use cases. This is going to change and evolve so much. There may end up hundreds of models—we might end up running one of our own.
Des: Even with OpenAI, we don't just use GPT-4. We use GPT-3 and GPT-3.5 as well. And there's an interesting question to be had even there, where not all businesses prioritize accuracy to the same degree. Some prioritize other traits, whether it's price or whether it's speed or other things. You have to be willing to listen to and learn from the market, and it might well be the case that we have to actually serve a spectrum of people. Some people want just whatever the cheapest they can get is. Some people want, no, it has to be world-class, best possible. And there could be data points along there. Again, we just need to be able to not make decisions today that restrict us to a very specific segment of the market when we believe that we actually built the tech that can serve the full breadth of spectrum.
Eoghan: We've built demo versions of Fin with multiple models, and we'll continue to experiment.
Can you talk about the previous era of chatbots and what worked, what didn't work, and how does that set us up for the current moment that we're in?
Eoghan: In my view, there's been three generations of chatbots.
The first generation was tools like Drift, which function by a bunch of conditions—”Did you want to talk to sales? Did you want to talk to support? Did you want to book a call? Did you want a demo?” It's like a phone tree in a messenger.
The second generation was our previous AI product, Resolution Bot, which used fuzzy matching and machine learning approaches to match questions to pre-written, curated answers.
The third generation is Fin and other GPT-powered bots that use LLMs to interpret the meaning behind questions and find the answers in big pieces of text.
The dirty secret is that bots, to this point, have been crappy. We actually saw nice resolution rates with our Resolution Bot product—sometimes up to 50%—but they came when people invested a lot of time and energy into programming it. For most people, the economics just didn’t make sense, or there was too much convincing that needed to be done for people to get there.
Bots, before today, made sense in thin use cases, but they were more of a feature, not a product. We, of course, have the phone-tree style bots at Intercom.
It makes sense in certain use cases, but they got their best traction in consumer instances where the actual surface area of the product was extremely tight and the volume of problems or support requests was really high. I'm thinking DoorDash or Uber Eats, where they know that roughly 87% of support requests relate to about 16 different problems, or three simple categories. For them, it's well worth them investing a phenomenal amount into building out that phone tree or first generation chatbot. None of them used generation two chatbots.
Beyond that, for the masses of SaaS companies and everyone else, bots made no sense. That's why I think these third generation chatbots like Fin really change things, because there's no setup required, and it's just practically more helpful because it doesn't require the user to know exactly the category of the question. They can interact with the bot in a human way. There's no learning for humans and there's no setup for the company.
How does customer service change in a world where 80% of queries are automatically answered by AI? What does the team construction look like? How are you imagining that?
Des: First of all, internally we have this manifesto about the future of customer support.
We believe support is about AI/automation together with humans, not versus or either/or. It’s both proactive and reactive, so support can also get ahead of problems instead of just waiting for problems to happen. Lastly, it is both omnichannel and conversational.
As to your point about 80% of queries being answered by AI, there's a few things going on there. One is that I see the support team moving towards being this first and last team. They answer questions the first time, because the bot hasn't seen it before. Once they answer it, it's the last time they see it—because the bot now learns from their answer.
We're seeing this happen already a bit, but I think it will evolve a bit from where we are today. Humans will tackle the harder, thornier issues that are either extremely complicated—as in proper backend bugs or logistical inquiries—or specifics like “Your package is stuck in Germany because you haven't paid the taxes,” or something that's not a one-liner.
Humans will deal with issues of high emotion and high urgency, where somebody's really angry and they just need to talk to someone. Most businesses historically could not have afforded to offer a “call me” option, but in a world where 80% or so of the undifferentiated stuff is gone, they can.
The piece I'm really excited to see evolve is the proactive aspect of support. So many businesses still wait for shit to go wrong before they jump in. You'd never run a real business that way. A restaurant wouldn't watch a guest sit at a table for hours and be like, “Oh, wonder what's going on over there?”
I think getting support teams onto their front foot and into a proactive state of mind will be incredibly ROI-positive. It lets support move up the value chain in a sense to actually be a driver of business growth. We don't know exactly where that will go, and I'm sure you've seen this with all your AI research and interviews, all of this shit could be out of date in five weeks time depending on what happens. But I do believe that this idea of support going proactive is an essential next step to getting really good at customer service.
One of the things that's mixed into this question is Intercom's business model—pricing per seat SaaS—and how if customer service teams shrink, that’s something where you're disrupting your own model. Do you anticipate having usage-based models based on tokens? Is that something that’s going to be part of every AI-powered SaaS company's business model? How do you think about the pricing part?
Eoghan: For as long as these LLM services are so expensive, it's going to be really hard for people to sell in a way that's divorced from any kind of usage metric. For them to be able to guarantee the unit economics, they'll need to be tied to costs in some way.
We're pretty much selling at cost, and we're trying to sell it for as cheap as possible, because we think that this is a great entry into the market. If we were trying to actually make a margin on top of the cost, it'd be really expensive.
We do think the costs are going to come way down, and you can imagine that when they do, just like any AWS service, they might then start to sell in some sort of flatter way.
A typical help desk might sell seats irrespective of how often someone logs into the app, and that's because the per unit cost of a login on the servers is just so damn cheap. That's how I think it's going to go over time.
You bring up an interesting point, though, about the tension between seats and these AI bots, and that's a consideration in our minds for pricing also. We know that we may need to cannibalize a little bit of our seat business, but our belief also is that a very small number of companies, perhaps just one, hopefully Intercom, will be able to build a really deep, rich AI bot product, and all the software that comes after the LLM service is where you'll earn your margin. I think we will earn a margin on this over time, and I don't think it's going to actually severely threaten our seats-based business.
Pricing is one of the most interesting aspects of this stuff, because customers don't really have a frame of reference. We started with $1.90 per resolution. That's when we believe that an answer from Fin has fixed the problem with the customer and it didn't need to go to the support team. And some people are like, “$1.90? Amazing. I pay $10 to $15 per resolution”.
Other people are like, “$1.90? Are you freaking kidding me? It's just software. You want me to give you $2 every time your software does nothing for me?” It's a totally divergent set of expectations, and we're so early in it right now. (Ed: pricing is now at $0.99 per resolution)
I think the human time concept—charging based on how long these agents are running for—whether or not that's what people call it, will be the winner, because that's the comparison in the real world.
For whatever it's worth, I don't know if this is interesting to you or not, but the nuances are actually more interesting than that.
When these bots get really good—like with Fin, where it does 50% resolution for some customers—people end up asking more questions. You'll actually have a lot more inbound and you might need about the same size-ish support team.
Can you talk about interoperability versus vertical integration? Are there benefits for having your help docs and your chatbot both powered by Intercom?
Des: There are definitely benefits to having everything on the same platform.
For example, if a bot sees a question, doesn’t know the answer, and sends it to a human, it creates a pretty magical experience if the human can answer the question, or train the bot, and update the documentation all in one fell swoop. That’s a magical experience that maximizes human productivity and efficiency and ultimately gets you this compound interest from doing customer support that you can’t otherwise get.
Somebody will point out it's possible to do this by daisy-chaining 11 APIs together, the experiences doing that are never seamless—once you start pasting in five different API keys, you're into the world of jank, and it's not going to happen.
Ultimately, of course, we will read from any help center to get you up and running. It's not a great pitch if we’re like, “Hey, we can solve all your support woes, but the first thing you need to do is rewrite your entire docs over in this new tool.”
Obviously, we'll get there with importers—we've already built some of this today, as it stands. We believe the final state here is you're going to want your bot and your help desk dialed together. You don't want a disparate bag of technologies trying to talk to each other. It's not going to work right. But to let people get started, we'll absolutely work wherever their docs are.
How do you think about how AI enables Intercom to go up against an incumbent like Zendesk on customer service? What can Intercom do that Zendesk can't? How do you think about the positioning against an Intercom?
Eoghan: Zendesk is an incredible company. They achieved giant things. They owned a whole market and they are synonymous with help desk. That's just so hard to achieve, and they did it in kind of a low-key way. They were just the best and most complete solution for a long period of time, even if they lost some steam over the years. I think they were excellent at go-to-market, which has not been our forte. We're outstanding at product innovation and software development. They were excellent at go-to-market and they were slow to build things. It's not really in their DNA to go and move fast on this stuff. I don't believe they even have an ML team.
Of course, they were just acquired by private equity in a contentious, difficult deal, and their founders are out. I expect that Zendesk's focus going forward—and this appears to be the case given the price increases we’ve just seen from them—will be the PE playbook, which is “All right, we bet on this business, but we believe that there's a lot of inefficiencies in it. We're going to increase prices, reduce costs, etc, and we're going to win at our current game and sell the company in two to three years.” That’s a little disingenuous to some PE, but most of it is like that.
Because of that, they're not likely to move fast at all on AI. I mean, we moved theoretically too fast—such that, if it didn't play out, it could have been a big miss for us. Of course, we're not out of the woods yet, but they were never going to do that, and it’s still TBD on whether they try to acquire someone to do this kind of AI in house.
If you look at some of the most interesting disruptive companies right now, like Rippling or Stripe, they build really broad tech for a lot of different problems as a platform. People have realized that you can achieve more at large if you've got everything in one joined up system.
Our positioning against Zendesk is that we are an AI plus human customer service platform, all-in-one, and they're not about to have AI and they're not all-in-one.
Is building a customer data platform (CDP) important when we talk about bringing more user data in so that the AI can be powered off of and personalized based on user data?
Eoghan: Whether or not Intercom is the central source of truth—and it is in a lot of companies—I just think it's important that there's a lot of data there that the AI stuff can build upon, or other types of automation.
Des: This is more of a market observation, but a lot of companies obsess over how much information they store for their customers. They all end up with this problem where they store data, but can't actually point to what they use the data for.
The usage of data is fundamentally more important than its hypothetical future value. In times like these, when companies are charging customers a lot of money for storing shit that they might one day want optionality on using, it's the first thing to go off the bill. People kill all that data that they had been storing.
I care more about information that's going to be useful—like whether you’re a premium customer, an account, or you’re in a marketplace, you're a buyer or you're a seller—because that actually guides the type of support experience you get. That's the stuff we care about: anything that informs proactive support like, “Hey, we're a project management tool and you haven't set up any projects yet. We should proactively support you in doing that.”
Is there a plan for integrating into the customer’s actual database to extract that information? How do you think that that information makes its way into the AI bot?
Des: A couple of things on this.
When you install Intercom, generally—and it has been this way since 2012 or 2013—you choose what information you send over to us from your database. If you're Spotify, say, you can send over the number of playlists people follow, their premium account status, how many friends they have in the network, whatever.
Our advice is send over anything that's going to help you deliver a better customer experience, but don't send over everything, because you're going to end up with just a big junky install. On top of that, you can log events inside the product. That's generally how the data gets over and gets attached to user records.
On the AI piece, the question is what should Fin consume before attempting to answer your query. The world we want to create is one where our AI chatbots have three things.
They have the problem that the user has said to them, which is like, “My shipment hasn't arrived” or “I can’t download this song”.
Then they have an understanding of the user from the CDP that tells them, “Oh, this isn’t a premium customer, so that's why they can't download the song.”
Then they go to the knowledge base and say, “Ah, it appears downloading is attached to premium.”
What we want Fin to do is basically say, “Given this problem for this type of user, here's our policy,” and write the answer.
The challenge for us is to make sure that Fin is drinking in the right context about the user, the right context about the business—i.e., the docs or whatever humans have said over 10 years of history through the help desk or whatever—and then coming up with the best answer. If you're sure about the answer, tell the user. If you're not sure, draft a reply for the support agent and let them take it from there.
That's where we need to get to, and it's going to be a pretty magical experience. Obviously, I'm talking ahead of where Intercom is today. We have this experience live for our own support team today, but it’s coming soon for the rest.
The thing we haven't looked at yet is giving Fin read-only SQL access to our customer's databases for it to go sniffing for what might be useful. I suspect that would be contentious, difficult, confusing, etc. But ultimately, businesses are actually simpler than that most of the time. There's not that many foreign concepts. Again, we're just trying to replicate what humans do, and humans can do this pretty well.
How are you thinking about marketing automation today? Is it essentially some form of proactive customer support? Have you played around with AI applications, in those use cases, and are there any promising results?
Des: We're not in the business of the more lead nurturing aspect of marketing automation—like, “Hey, all these email addresses fell off the back of a truck and now I'm going to augment them through some ZoomInfo type thing, and then I'm going to just try and nurture them into setting up a meeting.”
You can use Intercom to do that, but it's not what we designed it for. We're about our vision of customer service, which includes proactive service, but that’s distinct from marketing. Proactive means someone is trying to use or using your product, and you can help them, and I think we can use AI to help with that by inferring the important steps that a customer hasn’t taken in your app.
There are other little obvious sub-use cases of that like, “Hey, people in a state like yours usually talk to us about one of these things.” Even today, if you open our messenger home screen, it's AI that guesses what you're about to ask. It guesses that based on who you are, the screen you're in for the product, the state of your account, etc.
We haven't dipped into the generative marketing piece yet. You can imagine some version of the future where you say, “Hey, tell customers to do the right stuff.” Click and see what happens. I don't think we're going to get there. I don't think that'll be a polished customer experience. You'll get a load of marketing-happy, lorem ipsum-style text that gives generic descriptions to generic outcomes. And I think customers will be like, “What the hell is this?”
In general, as much as I'm a believer in AI, I'm also a believer in being clear and speaking well and cleanly to your users. As I said, most products, it's not like you have 900 use cases you need to nail. If it's project management, they need to invite people, start posting messages, uploading files. If you haven't done one of those things, you're not using their product. If you've done them all, you're probably using their product. If you stopped using their product, it's probably because their product wasn't good enough.
When I think about proactive support and AI, it's to work out the state of the user, and work out what the likely next best thing for them to do is, and then proactively offer that to them. To be clear, we don't have anything to say on that right now. We're working hard on the support use case. We think that, going back to that Maslow's hierarchy of support or whatever, proactive is the next stage once you're doing a great job of reactive. But we do want to get there. That's how we think about that space.
What are the core differences between the support use case with LLMs vs. LLMs with “marketing automation”?
Des: I haven't thought about it in terms of core differences, but there are definitely defined expectations around customer support. Customers want a reply, and there's a precarious situation in that if the customer doesn't get what they want, they might quit. It's a closed-world environment in that regard.
With proactive support, it does go a step earlier where people aren't expecting it. No one logs in to be like, “Oh, why haven't you already offered me blah, blah, blah.” We're not at that point yet. Now to be clear, there are businesses where that is expected. If you walk into a five-star hotel, you expect proactive customer support. If you walk into a Mercedes dealership, you expect proactive support. But we're not there on the internet yet and that's okay.
When you don't have expectations, it means that there's two things that happen.
One is that there's less of a definition of what “complete” looks like. Separately, you run the risk of pissing somebody off—like they didn't want to be nagged and they got nagged, and you're trying to force them to do something that you better be right about what you're doing. There's a subtle difference there.
Then, if you go one step earlier in the funnel, which is the pre-customer piece, you can imagine a generative AI paragraph that’s like “Hey, I know you haven't even heard about our product yet, but here’s some writing tailored to you because I think you work in fintech” or whatever.
When you get into that world, you run an extra big risk because your customer wasn’t asking for that email, probably didn’t even want this email, and then it wasn’t particularly well written.
The danger of that world is that you get people who say they sent 4 million emails and got 4 customers, so measure their success by the 4 and not the 3,999,996. Holistically, however, we just think about the overall customer experience. We want to be giving great service to our customers. That’s what drives everything that we build and everything about how we think about the products we make.
How has Intercom’s pricing evolved in the last eight months since Eoghan's return? What are the plans for pricing, and how do you use that competitively to avoid the bottom-up disruption from many folks who are building for AI customers?
Des: To sort of set the scene a bit, the reason Intercom's pricing has been such a pain point has been the breadth of service that we offer.
When we started Intercom, we were building a platform that turned out to be used in just a general, broad set of use cases. It was used in this sort of Drift-y, live chat/talk to you with visitors on your website. It was used to onboard your customers, do proactive support, and it was used in customer support. In a sense, we had three different tools all working off the same record.
We had very, very big customers like Amazon, and we had very, very small customers, like every YC startup. We were also B2B and B2C, and there's a big difference there in that B2B tends to see small customer counts and high value per customer, while B2C tends to see massive customer counts and low value per customer.
If anyone has one set of metrics and price points that can solve for that riddle, I'll take it—but it doesn't work. That's the background.
Going upmarket piece also fundamentally changed our commercialization. The whole idea of “Talk to us” on the pricing page comes from the fact that if you're a large business, you want to use us in certain ways but not in other ways. It's definitely easier to just talk to a salesperson. But, I think ultimately, we overplayed that. We've been progressively rolling it back, and you’ll see things like cardless free trials and stuff like that coming out soon.
In terms of what will change, we are firmly a customer service platform. That simplifies things a bit. We've launched our early-stage program, which is $65 for at least a year, and that gets you all of Intercom.
We are looking top-to-bottom at our pricing for our new strategy, and for customer service in a post-AI world, knowing that we really want to do business at both ends of the market.
One interesting thing we’ve seen is that even if we are going upmarket, we still have to care about what's downmarket.
Often, even the supposedly small companies often grow up to be big. Notion started using us when they were tiny, for example.
Sometimes companies acquire other businesses and they inherit Intercom that way.
Sometimes it's just two engineers working on a new, cool project inside a big company, and they say, “Let's put Intercom on it”, and their usage grows in that way.
The lesson is that we still have to really care about every new internet business, and the changes we’re going to make to our commercialization are going to reflect that we want every new internet business to use Intercom.
By the way, as a side note: we want simple, clear, transparent, and predictable pricing. Because of some units we charge on—like your number of your users—your Intercom bill can 10x if you have a great day. There's a spikiness to it that people don’t expect. We want to have simple, clear, transparent, predictable pricing, and that's what's coming in.
If everything goes right for Intercom over the next five years, what does it become and how has the world changed?
Des: We become the number one dominant and default customer service platform for internet businesses. We'll be the default choice because we think that our vision for the future—with AI plus humans, reactive plus proactive, and conversational plus omnichannel is the right way.
How will the world be better? We will raise the standards of customer service on the internet substantially—such that you'll either get instant replies to common queries or you'll get really well-considered, thoughtful, empathetic, accurate replies to messier ones. In either case, you're going to be treated really well online.
We've been saying for 10-12 years that our mission is to make internet business personal, and that hasn't changed. That's what we're here to do. We believe we'll just substantially raise the standards of customer service on the internet, and we'll do that from a position of strength within the market.
Disclaimers
This transcript is for information purposes only and does not constitute advice of any type or trade recommendation and should not form the basis of any investment decision. Sacra accepts no liability for the transcript or for any errors, omissions or inaccuracies in respect of it. The views of the experts expressed in the transcript are those of the experts and they are not endorsed by, nor do they represent the opinion of Sacra. Sacra reserves all copyright, intellectual property rights in the transcript. Any modification, copying, displaying, distributing, transmitting, publishing, licensing, creating derivative works from, or selling any transcript is strictly prohibited.