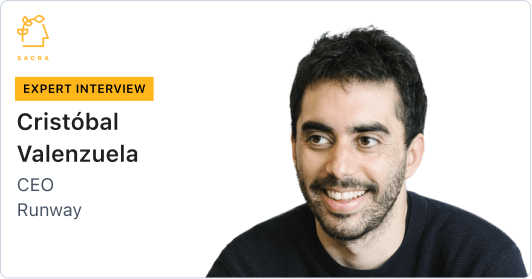
Background
Cristóbal Valenzuela is the CEO and co-founder of Runway. We talked to Cristóbal about the state of generative AI in video, foundational models for text vs. videos, and unit economics of generative AI for video.
Questions
- A lot has happened in generative AI in 2022 with DALL-E 2, Stable Diffusion, Midjourney, and ChatGPT coming out. Can you walk us through how you see the story of the development of generative AI over the last year?
- Have you seen your core customer profile evolve in the last one year, with the new developments in the generative AI space? Have you seen any new customer segments that have started using Runway?
- Can you compare at a high-level generating new videos via generative AI vs. generating new text and images? How are they different and how are they similar?
- In the world of text-based generative AI, people have this understanding that there’s a GPT-3 model, and then individual startups are adding a layer of fine-tuned models on top of it. Can you talk a bit about how Runway works?
- At ~$0.05 per query with roughly 2M users doing 100 queries per month, ChatGPT’s monthly costs would be at around $10M. How does that level of per-query cost and usage roughly match up to video?
- LLM/GPT latency would apparently have to come down and costs would have to come down about 10x before it would be feasible to integrate AI-generated results into search. How much of a bottleneck is pricing and latency for video generation today and how do you see it trending?
- Video post-production from a veteran editor costs roughly $250 per hour. At 20 hours per month, that’s about $5K—similar usage of Runway costs $12. Does this roughly track to how you think about Runway’s value and cost-savings potential? How do you see Runway changing how video editing/post-production teams work?
- Let’s take another creator tool — Figma — not only has it made creating vector graphics and UX UI cheaper, but it has also changed the way designers work by giving them something to collaborate on. Five years from now, how do you see Runway changing how post-production teams and video teams work together?
- With the costs of training models going down, what do you think prevents more startups from training their own foundational models, and owning their full stack?
- Andrew Ng observed that 80% of an AI developer's time is spent on data preparation and labeling and data is the big bottleneck to building AI. How do you see self-supervised learning from large text and image models changing the role of data preparation in building AI models/applications?
- Today, TikTok has a strong position in the consumer video market via their in-built distribution and wide selection of video filters that allow people to quickly make videos that would have taken hours to edit together years ago. How do you think about competing with tools like TikTok, or do you see them as more complementary to Runway’s more B2B focus? Is there a world where Runway tools would show up in TikTok’s list of video filters?
- What’s your take on talent availability for AI, given that it's a relatively new field and training the models, or even owning the full stack, in your case, requires certain specialized skill sets? What trends do you see emerging from this scarcity of AI in the market?
- Is there anything else you’d like to share with us about Runway or generative AI for videos we haven’t discussed?
Interview
A lot has happened in generative AI in 2022 with DALL-E 2, Stable Diffusion, Midjourney, and ChatGPT coming out. Can you walk us through how you see the story of the development of generative AI over the last year?
Yes, a lot has happened over the last year in the generative AI space. Not only have we seen an explosion and inflection point in the quality, scale, and controllability of large language and generative image models, but we've also seen an explosion in the practical applications of these systems in real-world scenarios. Having more practical applications of these models, with more people building on top of them, creates positive feedback loops to continue learning how these models can be adapted and improved to better align with what users want.
That inflection point might look like an overnight success, but the reality is that it has been brewing for years. Progress in the field has been steady over the last decade. I think it all started around 2012, around the time AlexNet was published. And since then, we’ve seen regular and continuous advancements in an almost exponential fashion. The critical difference driving the changes we’ve seen in the last year has been the quality of the outputs. Models are getting good at generating content, which has helped cross a chasm from being considered toys to being taken more seriously.
For companies building on this space, conviction matters. Because research takes time. We've been building Runway for four years now. The earlier versions of our generative models and generative products took more work for people to understand. Both in terms of usability and practicalities. Given that quality has improved, with models like our Latent Diffusion work, the usefulness realization has become obvious.
Human mental models must change to grasp the possibilities of new radical technologies fully. And changing a mental model takes time. In the case of Generative AI, there’s been a massive collective mental model adjustment in the last months that has finally helped us understand how useful this wave of AI is going to be. No more thought experiments are needed; everyone can experience it themselves with something like ChatGPT or Runway. But we're still early. We are still scratching the surface of what's gonna come.
Have you seen your core customer profile evolve in the last one year, with the new developments in the generative AI space? Have you seen any new customer segments that have started using Runway?
We remain focused on storytellers. Storytelling can take different shapes and forms. It can happen inside production and professional environments, like post-production companies and ad agencies that are already using Runway. It can also happen inside marketing teams and small teams — a handful of people working in a company or a small business. For Runway, Generative AI is driven by finding ways of embedding this technology to augment people’s ability to tell stories.
On the research front, we're focusing on multimodal AI systems to enable new types of creativity tools. Which will also translate into new market opportunities. At the core, this wave of AI research is enabling new ways of interacting with software and synthesizing media that will fundamentally transform existing customers' workflows.
A second-order effect of this new wave of improved models is the democratization of productivity and creative tasks that used to be very hard to do without highly specialized training. As models continue to automate what used to take multiple hours of work and translate that into seconds, we will continue to see leveling of capabilities that only a handful of people had access to. Think about creating professional ads, product photography, and editing a large volume of content. Anyone can use “Erase and Replace” — a tool we have in Runway that allows you to professionally swap a background and generate any sort of original background, hundreds if you want in seconds — opens the door for non-technical users to drive creative work without specialized tools.
Can you compare at a high-level generating new videos via generative AI vs. generating new text and images? How are they different and how are they similar?
Working with language models is slightly different from working with generative models for images and videos. Video is notably harder, given the temporal consistency component that needs to be considered. Making sure that you maintain the relationship between frames of objects moving in that frame.
The human eye has been trained to detect the slightest imperfection in a video frame. If you're generating a video from scratch or editing a video with the help of an automated system, the final result needs to work really well to retain the magical illusion of movements in frames. Those subtleties are one of the biggest challenges when working with video models.
There is also the iteration speed factor at which video models can get transferred into products. Video has this added complexity for decoding, encoding, streaming, and a multitude of small optimizations that have to happen. In addition, there are unit economics that also need to make sense since it’s traditionally a more expensive medium than working with text tokens.
Natural language has seen faster and more rapid improvements, but now, images and video are catching up. I expect video to be pretty much the center of research in the next couple of years when it comes to generative models.
In the world of text-based generative AI, people have this understanding that there’s a GPT-3 model, and then individual startups are adding a layer of fine-tuned models on top of it. Can you talk a bit about how Runway works?
We have a special approach to building products. Runway is a full-stack applied AI research company. We do the research, develop, and train generative models from scratch. We also develop the necessary infrastructure to safely deploy those models into production-ready environments and continuously improve them. That entails, for example, building any necessary systems to ingest video streams, dataset preparation, etc. And ultimately, we build real-world applications on top of those models and that infrastructure.
Owning the entire stack has the advantage of having full visibility and control over how the product gets deployed and how our users interact with it. This gets translated into the lowest research level if needed and helps direct product roadmaps and prioritization more effectively.
Our full-stack approach is visible in our Latent Diffusion work (High-Resolution Image Synthesis with Latent Diffusion Models is the full name of the paper), which we first released and published as open-source in late 2021. Latent Diffusion was the collaboration between two organizations — Runway and LMU Munich. Latent Diffusion has had a few open-source training iterations. Since then, we’ve consistently improved our original Latent Diffusion model, inference speed, the infrastructure and built multiple production-ready tools on top of it. And since the model was open-source, it’s been incredible to see the amount of innovation that has been built by the community. We do have some very exciting releases coming up that will go further than what LatentDiffusion currently allows from a technological perspective.
At ~$0.05 per query with roughly 2M users doing 100 queries per month, ChatGPT’s monthly costs would be at around $10M. How does that level of per-query cost and usage roughly match up to video?
It depends. Not all models are created equal. Not all models are used in the same way. In particular, video has a slightly more complex inference process than per-token queries from language models. And more importantly, you need to factor in what type of video task the user is requesting. Video editing and video generation are more than just generating a series of frames. Especially if you want to optimize for users having expression and controllability. In contrast, large language models, like the one behind ChatGPT, are taking advantage of zero-shot or few-shot techniques, which can generate good results with little to no new data. These models can more easily generalize to a wide spectrum of downstream tasks. No additional training required. Potentially, having one model to solve multiple problems like copywriting, code generation, chat applications, etc. The per-query costs of all those tasks will always be in the same ballpark, with inference optimizations being, for the most part, applicable to all tasks since it’s the same model. But in the case of video, just given the nature of the medium, the universe of transformations that can be made to all or parts of a video frame is a way more complex problem.
For Runway, the bet early on was to build a full-stack pipeline and uncover optimal cost-effective ways to deploy those models for creative use cases. And that also translates into finding optimal unit economics to deploy these kinds of models to millions of users. Considering all the quirks and nuances of how creatives work. It's a long-term investment. One that has not been necessarily easy.
There are short-term products and long-term products. We're focused on building long-term products. We’re a company building a product that will not offer marginal innovations or incremental improvements to an existing system. We're interested in inventing. Leap-frogging the current stack. That also needs to translate into our cost structure, making it cost-effective to use these models in production, something we spent a lot of time doing.
LLM/GPT latency would apparently have to come down and costs would have to come down about 10x before it would be feasible to integrate AI-generated results into search. How much of a bottleneck is pricing and latency for video generation today and how do you see it trending?
Overall, costs of computing across the stack will continue to decrease. Storage, GPU compute, database cost, all of it. Some will happen faster than others. Storage is pretty negligible today. That’s just the nature of sustained technological innovations, markets, and competition. We are still early, but we will continue to see the cost of training and running models decreasing over time.
Text generation and video generation are different products. From a cost and pricing perspective, they have different structures. Of course, you always want the cost of running a model to be as low as possible, which will directly impact the price. Pricing, cost, and latency are different dials that require constant fine-tuning to make a good product in this space. We have a robust video streaming pipeline with a low-latency network that makes editing and video playback fast. For generation, we run constant optimization experiments, like distillation on the diffusion process, to make inference as fast as possible. If you are building a real-time creative application, latency is key. You can’t creatively work with a tool that takes too long to respond to inputs. There’s still a lot to do on latency, but we’ll continue to make latency as optimal as possible.
Video post-production from a veteran editor costs roughly $250 per hour. At 20 hours per month, that’s about $5K—similar usage of Runway costs $12. Does this roughly track to how you think about Runway’s value and cost-savings potential? How do you see Runway changing how video editing/post-production teams work?
The two main impacts of disruptive technologies are cost and accessibility. They make things cheaper, simpler, and more accessible to more people. Video has historically been an expensive medium. From triple-A post-production companies making blockbuster long-form movies to small creators publishing on TikTok. Video tends to be expensive to work with and complex. Editing multimedia content is frequently a sophisticated and time-consuming process. Creating a video nowadays involves using a software stack and a set of primitives that were invented almost 35 years ago. The biggest impact of Generative AI on the video industry is that it will drive the cost of content to zero. We will continue to see a large decrease in the cost of creating professional content across different disciplines, making them more convenient to use.
Today, a professional artist working in the film industry can save hours, even days of work, using Runway. This was the case, for example, for the VFX team behind the film "Everything Everywhere All at Once", directed by the Daniels. A beautiful movie that most probably wins dozens of awards. “Everything Everywhere All at Once” is a film with abundant and very impressive visual effects that make the story very unique. Although the most impressive feat is that only five people ended up doing the majority of the visual effects shots. What used to take dozens of teams working around the clock for months or even years is now feasible for a small creative team that can leverage new technologies, like Runway’s Green Green, very fast. Automating the tedious and time-consuming aspects of the editing process.
I believe that the way “Everything Everywhere All at Once" was made will set the tone for what’s to come. It’s a primal example of the true power of disruptive technologies and the impact they have on storytelling.
Let’s take another creator tool — Figma — not only has it made creating vector graphics and UX UI cheaper, but it has also changed the way designers work by giving them something to collaborate on. Five years from now, how do you see Runway changing how post-production teams and video teams work together?
I think collaboration is at the heart of every creative endeavor. Great things are created in a collaborative environment. What Figma did exceptionally well was putting collaboration at the heart of the product value. Today, collaboration is not a good-to-have feature, it is table stakes. Is not a moat, it is a basic workflow you need to build. Is how modern teams get things done.
For Runway, collaboration is at the center of the product. It is a must. What we're offering is a set of automation tools and creation tools that use AI in radically new ways. But we also offer teams the chance to iterate fast, to collaborate more conveniently.
Runway is allowing professional teams to work together in ways that, beyond the AI components, were very hard to do a couple of years ago. That's the real promise of a new set of creative tools; they are accessible, fast, web-first, and collaborative.
With the costs of training models going down, what do you think prevents more startups from training their own foundational models, and owning their full stack?
Most of the things that need to be invented and built in the AI space are still ahead of us. There are large opportunities at a research level and all the way up to products that can leverage commodity models and API. And so, it is essential to understand where to focus as a company and what strategies to deploy. Every company is different. Every company needs to find a focus. For Runway, our core belief since the day we started was to prioritize having full autonomy and freedom of exploration at every level of the AI stack. We aim to build radical new tools and solutions that we can’t even imagine today. But to enable that to happen, you need to build a strong organization with those cultural elements at the core. Those values drive product innovation and allow you to learn quickly, dropping preconceived ideas behind to follow opportunities down to the bare fundamentals. Is first principles thinking applied to company building. It took us years to iterate on how to build the knowledge and best practices to know how to drive research forward, train models from scratch like we did with our Latent Diffusion work, and then translate those findings into usable systems.
A research paper is not a product. Training a model is not a product. A product is a way of solving a problem. A model can help you do it in some way. But there’s a lot more that comes down to product building. For us, owning the stack is a long route that takes tenacity, patience, and a learning mentality. Training foundational models is just as important as knowing how to take them to valuable solutions.
We are now working on better versions of generative models that are far improved from the original Latent Diffusion work. So, if you put that into perspective, the current state of diffusion models is a milestone but expect many more models over the next years.
By the way, part of the exciting aspect of open-sourcing Latent Diffusion was to see the explosion of creativity that emerged. Those are the most rewarding aspects of open source. But open sourcing and building an open source company are very different from building a product company, and we're 100% a product company driven by applied research.
Andrew Ng observed that 80% of an AI developer's time is spent on data preparation and labeling and data is the big bottleneck to building AI. How do you see self-supervised learning from large text and image models changing the role of data preparation in building AI models/applications?
Anything that can make the data preparation process for building AI models and applications more efficient will be welcomed. Particularly if you can maintain quality at scale. The less time and resources that need to be spent on collecting and labeling data and more on fine-tuning, new architectures, etc., the better. Additionally, if models can learn more useful representations from unlabeled data that later can be used for a wide range of tasks, it will further reduce the need for task-specific data preparation. However, the main factor is still quality. Especially in image generation models where the aesthetic components matter significantly. I think we might be heading towards a future where most of the data preparation will become more the responsibility of end-users that can fine-tune models to downstream tasks and special domains. Currently, models are great at general tasks but can have a hard time in domain-specific areas. That’s why I believe fine-tuning models with particular aesthetic qualities will be so important.
Today, TikTok has a strong position in the consumer video market via their in-built distribution and wide selection of video filters that allow people to quickly make videos that would have taken hours to edit together years ago. How do you think about competing with tools like TikTok, or do you see them as more complementary to Runway’s more B2B focus? Is there a world where Runway tools would show up in TikTok’s list of video filters?
Distribution platforms are evolving, and content is being consumed differently. If you're a professional team and creating a video, you might have to explore and create different versions of that video for different audiences. TikTok is only one of them. Consumers, prosumers, and professionals alike will tend to converge more and more similar tools. Mostly because the tools themselves are becoming more accessible, powerful, and convenient to use across different domains. So, it doesn't really matter if you're just a casual creator or a professional; you can use some aspects of the same tool and process.
As content generation driven by AI becomes more accessible new changes to distribution will emerge. Generation and distribution will be extremely close, happening almost simultaneously. We are close to having generated social media apps. Entirely generated YouTube videos. Generated Netflix shows and so on. All in real-time. The tools and primitives you’ll use to generate those stories, to craft those ideas, will be far distanced from whatever we are using today. It’s a new medium that has new affordances and new possibilities.
What’s your take on talent availability for AI, given that it's a relatively new field and training the models, or even owning the full stack, in your case, requires certain specialized skill sets? What trends do you see emerging from this scarcity of AI in the market?
The world is full of scarcity. And in the world of AI, there is a scarcity of a few things, but GPUs and talent are probably the most important ones.
For talent, there’s been an overwhelming amount of interest in the space, and more people are flocking in to become researchers or AI engineers. Which is phenomenal. Although, my belief is that the best teams building in the space will not be formed by only domain experts. The unexplored emerging qualities of generative models will most likely be uncovered by multidisciplinary teams of artists, hackers, and researchers. It requires a unique cross-domain experience and non-traditional backgrounds. AI values divergent thinking and exploring uncharted territories with an original mindset. I’m excited for talent to come from diverse backgrounds and disciplines. That’s when you really start to move things forward. Bringing in new sensibilities and understandings of the world. The perfect talent to cultivate combines an artistic, aesthetic sensibility and good technical understanding of how the technology works.
The other trend will be GPU availability. Most AI models leverage GPU computing either for training, inference, or both. GPUs are scarce, and having a constant supply becomes fundamental. I’m intrigued to see what will happen as the demand for GPU continues to grow, probably in an exponential manner.
Is there anything else you’d like to share with us about Runway or generative AI for videos we haven’t discussed?
I think 2022 was an inflection point for Generative AI. As a company building in that space for the last four years, it's clear that now the field has gotten the attention it deserves. But 2023 will be even more critical. Probably will be marked by the largest and biggest advancements in the space. Natural language and text applications are moving incredibly fast, but now, generative video will start to move at the same lighting speed. We are already working on a few models and a few products that are going to be announced and released earlier in the year. Perhaps this is the most exciting time to be building in this space because most of the things that we need to build are still ahead of us.
Disclaimers
This transcript is for information purposes only and does not constitute advice of any type or trade recommendation and should not form the basis of any investment decision. Sacra accepts no liability for the transcript or for any errors, omissions or inaccuracies in respect of it. The views of the experts expressed in the transcript are those of the experts and they are not endorsed by, nor do they represent the opinion of Sacra. Sacra reserves all copyright, intellectual property rights in the transcript. Any modification, copying, displaying, distributing, transmitting, publishing, licensing, creating derivative works from, or selling any transcript is strictly prohibited.