- Valuation Model
- Expert Interviews
- Founders, funding
How does self-supervised learning reduce the need for data preparation and labeling for AI developers?
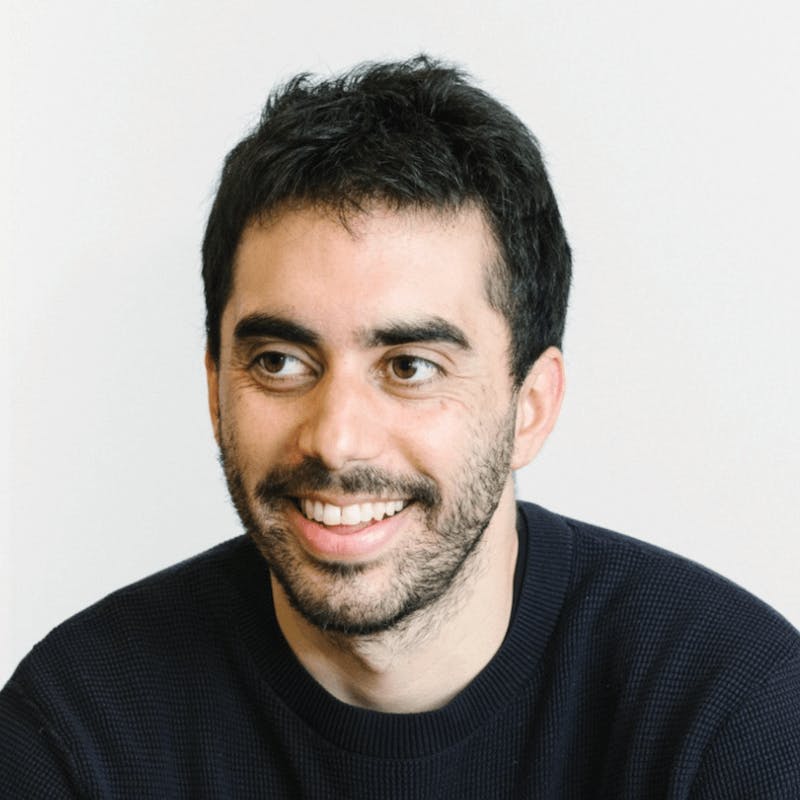
Cristóbal Valenzuela
Co-founder & CEO at Runway
Anything that can make the data preparation process for building AI models and applications more efficient will be welcomed. Particularly if you can maintain quality at scale. The less time and resources that need to be spent on collecting and labeling data and more on fine-tuning, new architectures, etc., the better. Additionally, if models can learn more useful representations from unlabeled data that later can be used for a wide range of tasks, it will further reduce the need for task-specific data preparation. However, the main factor is still quality. Especially in image generation models where the aesthetic components matter significantly. I think we might be heading towards a future where most of the data preparation will become more the responsibility of end-users that can fine-tune models to downstream tasks and special domains. Currently, models are great at general tasks but can have a hard time in domain-specific areas. That’s why I believe fine-tuning models with particular aesthetic qualities will be so important.