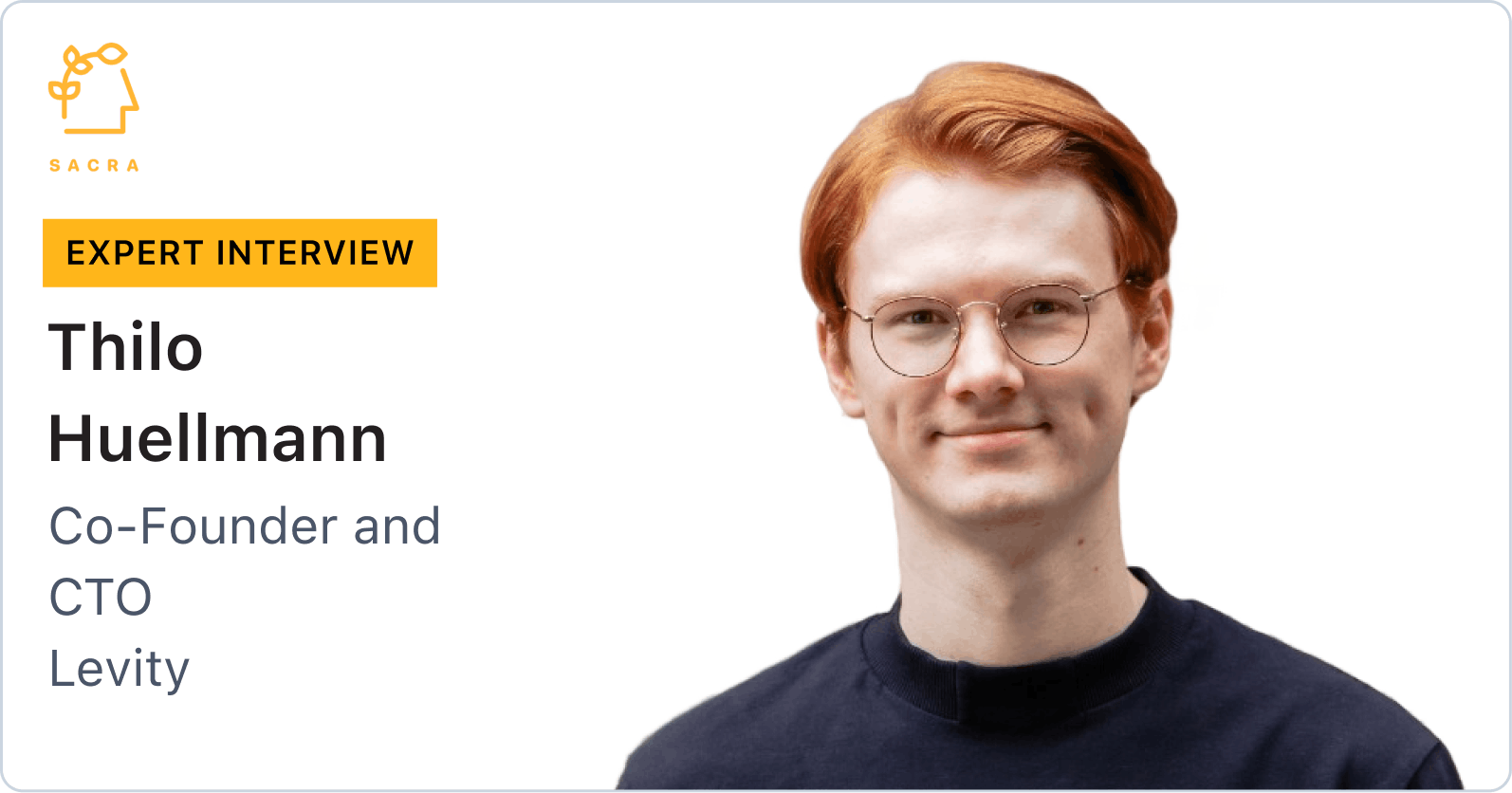
Background
Thilo is the co-founder and CTO of Levity. We spoke to Thilo about how businesses can use AI to automate workflows, the hard part about building an AI tool in the cloud, and competitive dynamics in the workflow automation market.
Questions
- Can you tell us a little about Levity? What is the problem that Levity is trying to solve?
- Can you talk about your core customer profiles and some of your key use cases?
- What are your thoughts on the workflow automation space and how it’s evolved? What does the future look like?
- Where do AI-based tools like Levity and others fit in in this market? Do you see them as replacing existing tools or as complementing and extending the value chain?
- Do you see breakpoints where customers duct-tape together their own solutions with existing automation tools and eventually graduate to something which is automated or AI-based like Levity?
- How does Levity actually work? What are the various components that come together from an AI and data model point of view?
- Can you double-click on how your customers are currently importing their data? You mentioned import integrations. Is it self-serve or do they reach out to you?
- What is the real hard thing about what you are building right now that many people who are not trained to understand AI will not really appreciate?
- How do you optimize infrastructure and manage operational costs? As you scale, how different will your infrastructure look—will this grow by 10X or 100X?
- With regards to the modeling and the data needed to train it, what happens if the data is not sanitized? What are the steps you share with your customers or take on their behalf to make it more consumable by the AI engine? Have there been issues with the quality of data used?
- Are there any trade-offs between using a no-code tool versus developing something like this in-house? Are there situations where one fares better than the other on cost or performance parameters?
- From a customer retention point of view, what are some of the things which make Levity more sticky?
- The no-code market is full of solutions that deep dive into specific vertical use cases—revenue operations, fraud detection, revenue forecasting, and so on. What went into that decision to build Levity as a horizontal solution?
- Many SaaS or cloud-based companies offer a freemium tier or free trials. Levity starts with a paid plan as its lowest tier. What was the thinking there?
- Levity 1.0 essentially is about data categorization, tagging, and labeling. What's your vision for Levity 2.0 and beyond?
- In terms of your tech, vision, and product, what will Levity look like five years from now?
Interview
Levity gives non-technical people in small- and medium-sized organizations all the tools to automate workflows that they couldn't automate before, because they needed machine learning and developer expertise, which is always scarce.
On the meta level, we solve input problems around unstructured data coming from third parties and we cover the whole value chain end-to-end, from raw historical data to fully-integrated solution without any developer involvement.
For example, some of our customers reach out to their customers with regular offers via email and SMS. People respond to those with either "Yes, I'm interested” or “No, I'm not interested.” or even “Delete my contact information". In the past, our customers would process these responses manually because people would write all sorts of things. With Levity, they just ingest all processed responses from the past and train a custom text classifier on that. From there on their classifier can do this categorization task on its own.
Can you talk about your core customer profiles and some of your key use cases?
We focus on the non-technical people that want to (or have to) solve these kinds of problems by themselves. The types of organizations we're targeting have between 100 and 1,000 full-time employees and are fairly digitized. These aren’t companies that still work with pen and paper, but ones that have already automated most processes that can be automated in a rule-based way.
Many of them are very familiar with tools like Zapier already, but they're stuck when there's unstructured data. Often times they never thought about automating it because they thought it was not possible for them.
The type of person we look at probably leads a small team and considers themselves to be a “process owner” in that team. They’re constantly looking for ways to optimize their processes and operations to make their team more efficient. It could be someone like a Head of Marketing or Operations, for example.
What are your thoughts on the workflow automation space and how it’s evolved? What does the future look like?
It's a vast space with many different tools that do many different things. All of them have a right to exist in my opinion and I don’t believe there will be a single player or a few players that can just overtake the market. Each of them has a different angle and solves similar but different problems. One major segment is of course the RPA space which is mostly relevant for large enterprises like the Fortune 500. In my opinion RPA is often just a band-aid for broken workflows in legacy systems such as SAP and the technology is very flaky because it relies on screen scraping and click bots. And if you miss one edge case you have a problem. I don't really see how that technology is the future, because the up-and-coming companies that will be relevant in ten to twenty years won’t build on top of SAP.
Another space is something like Zapier and Integromat for SMBs. And there are also Zapier alternatives, such as Tray and Workato, for larger companies. Zapier, Tray, Workato and alike have better tech because it’s APIs talking to each other and everything happens in the background instead of a click bot on someone’s PC. Zapier is quite mature already, but they still have considerable room to grow. I assumed that most people in my broader network of young, technology-minded people have known about Zapier for years, but I often find that they've never heard of it. So, there's still lots of room for them to grow and show that something like RPA might not be the best long-term solution for the problems that exist. I also think that it was a very smart move by Celonis to acquire Integromat, especially at that price. Combining it with their process mining technology has enormous potential.
As a general trend, these technologies arise because there's also an explosion of new tools and lots of APIs that are not built in a way that they can talk to each other natively. There's a need for a Zapier or a Tray to bridge that gap and it will only get worse in the future so they’re not going anywhere.
Where do AI-based tools like Levity and others fit in in this market? Do you see them as replacing existing tools or as complementing and extending the value chain?
Many companies we work with are already heavy users of tools like Zapier, but that isn't really a good fit for the types of problems that we're solving. For us, there's definitely an overlap with a tool like Zapier, but it's not like we're replacing it or vice versa.
Usually, people that approach us have done the task that we're automating, manually, before. Maybe they tried automating it but failed. So, they're not coming to us from a different tool. They’re coming to us from nothing and we're the first tool that works for them.
The easy things that work in a rule-based way are either automated or going to be automated soon, because there's no reason to not set something up that just does the task for you, especially if it's repetitive. But then, there's this vast space with things that are not as easy or quite ambiguous due to this unstructured data that is not automated yet. That's where we come in.
By the way, we also integrate with Zapier, Integromat and others because we see ourselves as complementary. With our own, native integrations, however, we can offer a higher abstraction layer and opinionated UX because of the specific “job to be done” that Levity is hired for. Integrating a Levity classifier into your workflow takes just a few clicks, doing the same with Zapier could take two or three Zaps with dozens of steps.
Do you see breakpoints where customers duct-tape together their own solutions with existing automation tools and eventually graduate to something which is automated or AI-based like Levity?
Definitely. That's also why we’d rather focus on companies with 100+ full-time employees because with the really small ones or sometimes, larger companies with "small processes”, if there's insufficient data, there are multiple issues.
First, you can't really train something that works well if you don’t have enough historic data. Second, if the volume that you're processing is low, there's no urgent need to automate it. It only really gets painful, if some person is spending 10 to 20% of their time doing this task, so, when you scale, you need to hire more such people. That's what we've seen often.
Initially, people tell us, "We're doing it with two interns or two working students right now. It's not an issue." A year later, they have 50 of those people and then, it becomes huge. That's why the amount of data that's being processed is an important factor for how much of a pain it is and how likely it is that they will succeed.
How does Levity actually work? What are the various components that come together from an AI and data model point of view?
We have a very standard React web application on the frontend that people interact with. Our backend is built on Python/Django to process the requests that they make. Apart from that, we have built dedicated services for the “heavy-lifting” components of our technology, such as data import, data processing, training and deploying machine learning models.
Currently, our ML stack consists of PyTorch as a baseline framework and we work with Valohai as an MLOps solution, which runs inside our AWS. We run the data pre-processing and model training on Valohai which makes it very easy to spin up GPU instances to train models in a timely manner. It's a great solution.
The other part concerns data upload and data processing. This can be quite challenging especially if a customer comes to us with historical data of a million rows in an Excel sheet that they want to upload using our web application.
You’ll often see SaaS applications limit CSV import to a couple thousand rows, because it’s a technical challenge and it becomes even worse when people want to upload thousands of PDF documents from the past. Then, you need to do all sorts of different things, like splitting the PDFs into pages, running OCR on them, and transforming everything into a format that makes it possible for the ML pipeline to consume.
Therefore, data upload and data processing is a major part we're building. Connected to that are data import integrations, because you may not always have the perfect dataset on your PC ready to be uploaded. Often, it's stuck in one or multiple apps and not structured the right way.
Earlier, when we didn't have these integrations, people often told us, "I need a developer to get me that export, clean it, and transform it before I can upload it to you." So, we started integrating with those data sources to make it easier to extract and ingest data into our system.
The difference here is that it's not like a normal ETL where you have databases with rows and columns that you want to import. It's unstructured data, like images, PDF documents, or raw text that needs to be processed a certain way. That also makes it more challenging because there isn't that much existing tooling out there.
Another major component is the actual workflow integration. Usually there's a trigger like “new email”, then you run that data through your Levity classifier and get a prediction. After that, you want do something with that prediction. That's where we need to integrate with lots of tools to make it possible for our users to put whatever they have trained to use and integrate it into the actual workflow.
Can you double-click on how your customers are currently importing their data? You mentioned import integrations. Is it self-serve or do they reach out to you?
In the beginning, we handled it manually for a lot of people and learned a lot from that.
Now, we have built the integration into the workflow. If, for example, you want to import data from historic emails on your Gmail but you want to import a certain type of email where you've maybe tagged yourself or that follows certain rules, then, you would get an interface where you could query Gmail as one of the services and get a list of historic emails. You could then take the body of these emails and import it into Levity. That’s one example.
But we’re building the same for things like CRMs, cloud storage providers and other systems of record. It's basically self-serve, so, you would say, "I want Levity to be able to read my files on Google Drive." You’d connect and then, select the files that you wanted to import and move it to our servers.
What is the real hard thing about what you are building right now that many people who are not trained to understand AI will not really appreciate?
There are many hard things. On the machine learning side specifically, it's not as much about the ML itself because we're just applying what is already out there, proven, and available open source. Text and image classification is not a hard machine learning problem.
What’s hard is everything around it. Putting everything into production and covering every step, from having some raw data somewhere to having a solution that does the task that you want to automate end-to-end.
From a cloud infrastructure point of view, it's also quite challenging. Let's say, if I'm a data scientist in a small team of a mid-sized company or even a large company, perhaps I run a few dozen models. But we have to build something that can support tens of thousands of concurrently deployed models that need to be available at all times because requests and prediction requests can come anytime. These models, especially in the text space, have become very large recently which makes it a challenge to deploy them in a cost-efficient way.
That's one of the machine learning-related things that we need to solve because we also want to make it possible for small- and medium-sized companies to use this technology. We cannot charge these companies 10,000 Euros a month for that. If we could, then it wouldn't be a problem, we would just throw money at the problem. But we have to build the infrastructure in a way that it works at a lower price point for larger numbers of companies in the long run.
How do you optimize infrastructure and manage operational costs? As you scale, how different will your infrastructure look—will this grow by 10X or 100X?
We do a lot of things on top of Valohai, which runs inside AWS but it also runs in any other cloud. That’s good if we want to switch or need a lot more resources. There’s been quota issues recently and it was hard to actually get more GPU resources from the cloud providers, probably because of chip shortages and crypto mining. That made it very hard to say, "I want 50 more powerful GPUs now."
The challenge there is we don't have a constant load on these GPUs. It's not like I know I need one GPU and then, I add a hundred more customers and suddenly, I need two GPUs. It's hard to predict when people make training or inference requests so we have to build things in a way that allows them to scale up and down very quickly.
Recently, we have adopted a new technology from AWS called Sagemaker Serverless. It's not running the model all the time, but it’s responding to requests whenever they come in. It's similar to Lambda and I think built on top of that, so we don't have these big costs of a model sitting and waiting for requests all the time. We drove the costs down to just a couple dollars per month to host a single model but that was still not economically viable for the ones that only got sparse requests or not too many requests in a month.
Now, we’ve switched to something that's actually serverless. For the training, it just spins up the instances as they're needed, and then shuts them down a few minutes later. It can scale really well and it doesn't explode in cost just because we have more customers. We're trying to keep it variable where it's important, so that we don't have fixed costs that just grow all the time.
With regards to the modeling and the data needed to train it, what happens if the data is not sanitized? What are the steps you share with your customers or take on their behalf to make it more consumable by the AI engine? Have there been issues with the quality of data used?
The biggest challenge in machine learning is always the data.
You can only improve your models by a few percentage points of performance by tweaking them but when it comes to data it follows the garbage-in-garbage-out paradigm. For us, there are definitely many cases where customers approach us who don’t have the data that they would need to be successful. Then, we have to be very transparent and tell them, "It might work. It might not work. You have to try it out, but we make it very easy for you to quickly conclude whether it works for you or not."
It’s a lot about expectation management, but it also really benefits if you get quick results, rather than start a machine learning project and then find out three months later that your data is insufficient and you can’t really do anything about it.
We don't do specific or manual steps with our customers. If they book a call with us, we give them a few tips to help but usually, we just have a bunch of heuristics in place to improve what's there and pre-process the data a certain way. Other than that, we deliberately don't adapt models per problem or customer.
Are there any trade-offs between using a no-code tool versus developing something like this in-house? Are there situations where one fares better than the other on cost or performance parameters?
There are cases for both.
First, many companies are actually not in a privileged position to make that decision because they don't have the resources at all. They might have developers, but they are usually already overworked and don't have machine learning expertise. Even if they had machine learning expertise, the in-house ML teams don’t work with us because they're busy with two or three high leverage things like for eCommerce it would be pricing optimization. They don’t work much on internal process optimization to make some teams 10 to 20% more efficient. Since their time is limited, there's just a long tail of things that have not been automated yet and would stay that way indefinitely.
Some customers told us they’ve tried doing things internally but oftentimes it’s a very slow back and forth between the person that has the problem and the person that can solve the problem. Then, you may have something deployed and running, and if you want to make a change, you have to reach out to the machine learning person again, and then, they have to retrain. It becomes very cumbersome to maintain. It's like Webflow where you're not reaching out to the developer anymore to make changes to your website, but you're just doing it yourself and then, hit “publish.” It's the same with us, you just hit “train”.
We're placing the tools to solve the problem in the hands of the same person that has the problem. There are cases where this is the best solution, and there are cases where you need to tweak for those last few percentage points of performance because it has such a big impact on your bottom line. Then it does make sense to have your own people to dive deep into it but if it's just a very basic thing where you need to classify some texts and you have the data at hand, then we’re often the better option.
From a customer retention point of view, what are some of the things which make Levity more sticky?
Customers usually come to us after having tried to do this manually and then, usually scaling linearly. Let's say, the company doubles and they need twice the people to do the task. When they automate the task with us to enjoy time savings, it’s great. Now, if they switched this off and went back to the original way of handling it, that probably wouldn’t work. They cannot just process it manually again, that’s not an option anymore.
With regard to competition, in general, when we looked at the space or started the company, we were in the same position as our customers and that's why we started building it. We saw a lot of players go very specific and very deep into one part of the problem space, like data labeling, for example, or model training, model deployment, or the whole integration or workflow automation. They only solved one part of the problem.
In order to replace us with other software, many customers would have to combine many different tools that all of them have and go very deep into their problem space. We’d rather go wide (end-to-end) and only focus on some problems.
The no-code market is full of solutions that deep dive into specific vertical use cases—revenue operations, fraud detection, revenue forecasting, and so on. What went into that decision to build Levity as a horizontal solution?
We were in the same position as our customers or prospects in the sense we also had a specific machine learning problem and we looked at whether there was a quick and easy and pre-built solution out there for this. There wasn't.
We realized that there was so much white space between those vertical solutions. While there are these big things like fraud detection or customer support applications where people or companies really go very deep and vertical, and it makes total sense, it's like they're probably a lot better at that than we could be.
Sometimes, we compete with them. Sometimes, people build similar things with us. It’s similar to Airtable where people use it as a CRM, but it's definitely not the best CRM. Maybe that's the entry point where they get started. Then, they build other things that are very custom and specialized and where there isn't a vertical solution available. So, they have ten things running on Airtable and they're using it long-term but maybe by that time, they have already moved to a proper CRM which is what we did.
Our first CRM was built on Airtable. Now, we're using HubSpot. But we didn't stop using Airtable. In the machine learning space, there are many things where people, first of all, need to train on their own data. It's such a special problem that it wouldn't really make sense for anyone to build a vertical AI company around it.
We have many examples for this. There are some special laboratory image processing cases where they train the model on their own data. There are maybe a few dozen other companies in the world that do the same thing and need the same solution, but that's not enough to build a vertical business on.
Right now, this is only covered by ML consultants, agencies or internal dev teams where these custom solutions are needed or where it's not possible to build a vertical. That white space is where we see lots of opportunity for us.
Many SaaS or cloud-based companies offer a freemium tier or free trials. Levity starts with a paid plan as its lowest tier. What was the thinking there?
Initially, we were completely closed. So, people just signed up and shared some information. We reached out to them and manually onboarded everyone. We were very deliberate with that.
Now, we're in the position where we only have a paid plan. The reason we do this is because we want to filter people and only get those that are 100% committed, know what they want to do, and are willing to pay. However, we will also have a free trial that anyone can get started with very soon.
Why we haven’t done it yet is also because of the infrastructure challenges we faced in the beginning. How can we support thousands of models concurrently? If one person trains ten models and if we have a hundred users, that would already be a thousand models. That's a challenge we needed to solve first before opening up further.
The next step for us will be a free trial. Maybe in the future – and it's definitely a goal – we will also have a free plan of some sort to really lower the barrier for people to get started and experience the product. It wasn't easy to build a product in a way where this was actually possible without us being exposed to cost and scaling risks.
Levity 1.0 essentially is about data categorization, tagging, and labeling. What's your vision for Levity 2.0 and beyond?
Extraction of data is also a big space, but it's essentially the same dynamic. There are, on the one hand, vertical solutions like invoice data processing companies or maybe they have invoices and then, they have ten other types of documents that are just very common across many companies. So, you can build a vertical around it.
Then, there's the long tail of very specific things. For instance, we have a customer in the hearing aids space and they get PDF scans of hearing test results. You can’t really offer them something off-the-shelf and say, "You can just plug this in." Instead, they’ll have to build something custom on their own data.
Now, the problem there is that building custom data extraction on, for example, PDF documents or also text, is very hard and you need a lot of labeled data. That is very costly and time-consuming. I think we can solve that with the large language models that are available now, like GPT-3 and its open source competitors that could eliminate the need for a lot of training data. That's what we're looking into right now, making extraction possible without having to label thousands of examples in a custom way, so that it works for your very specific things that are not just invoices or CVs.
The other thing also dependent on how the research progresses there, is multimodal. Right now, we focus on text, images, and PDF documents. But by combining things, we’re going one level higher in what a human is doing on a cognitive level. Categorizing stuff is very basic. It's only one specific thing like, "Oh, there's a text. I want to know the tag." In many decisions that people are making, there's more than just that. There are many different things flowing together, like tabular and text information, and perhaps an image too. Then, you may need to make one or multiple decisions on that data. That’s not been solved yet from a machine learning perspective, but it will be eventually. That's where we see a lot of opportunity and that is dependent on ML progress.
What isn’t dependent on anything is integrating more deeply, not just with the data sources but also with workflow integration and automation parts and that’s where we want to improve Levity. We want to build everything more native and give people more ways to transform data before they upload it to us, so that the whole issue of not so clean data or data not being in a format that we can use is minimized. We are working on that.
In terms of your tech, vision, and product, what will Levity look like five years from now?
Since we focus on the smaller underserved companies that weren't able yet to benefit from this technology, our goal is to make them more powerful than the big players.
If you’re a small company in the logistics space, for instance, you can’t leverage as much data as your larger competitors. If you just build ML for yourself, they might outperform you all the time because they have bigger datasets.
However, if there are many small logistics companies doing similar things on Levity, they could collaborate. Without needing actual access to other companies’ data they can pool their data to create better outcomes, because even if the dataset is the same size or smaller than their larger competitor, it will be heterogeneous and that will make those models more robust.
That's what we want to go after. We want to show people, "There are so many other companies doing something similar to you so if you work together, you will all have a way better outcome." For us it's great because it’s self-reinforcing and people stay with us long-term.
Disclaimers
This transcript is for information purposes only and does not constitute advice of any type or trade recommendation and should not form the basis of any investment decision. Sacra accepts no liability for the transcript or for any errors, omissions or inaccuracies in respect of it. The views of the experts expressed in the transcript are those of the experts and they are not endorsed by, nor do they represent the opinion of Sacra. Sacra reserves all copyright, intellectual property rights in the transcript. Any modification, copying, displaying, distributing, transmitting, publishing, licensing, creating derivative works from, or selling any transcript is strictly prohibited.