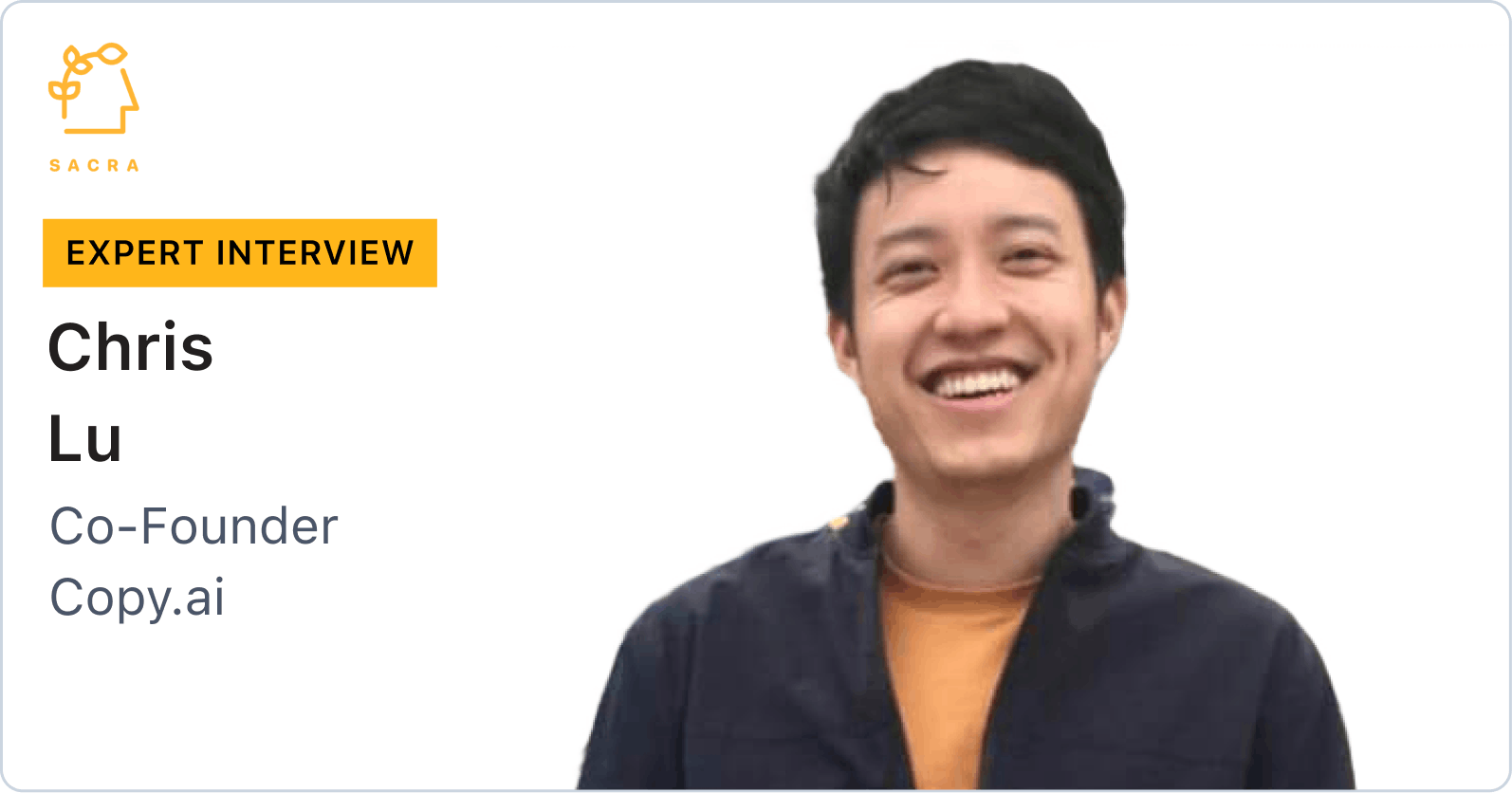
Background
Chris is co-founder of Copy.ai, a generative AI application for GTM teams. We talked to Chris to learn more about the effect of ChatGPT on prosumer use cases for AI and Copy.ai's strategy and positioning in selling into the enterprise.
Questions
- How has the generative AI space evolved since we last talked in late 2022 and especially since the launch of ChatGPT?
- Can you talk a bit about the transition that happened at Copy.ai from this more prosumer-type product to enterprise?
- Where do you see the potential remaining for consumer products alongside ChatGPT?
- We're seeing this pattern where people are using their own models more and more, but multiplexing with e.g. GPT-4 or Claude for higher-value use cases like generating long text. Do you divide up your use of models like this at Copy.ai?
- Can you talk a bit about what you're seeing in the enterprise around adoption of AI? In general, are you seeing big companies (that you wouldn't have expected in 2022) interested in adopting new go-to-market platforms?
- It seems like Writer is going hard on this idea of being the Grammarly for the enterprise, while Jasper is drilling down more into the marketing use case and Copy.ai is a little horizontal across the teams involved in go-to-market. How do you see these different niches evolving and how do you see them developing?
- One thing I wanted to circle back on is you talked about the PLG motion that you still have with some of the enterprise customers, and you mentioned the CMO-CRO region is where you're really selling to. What does that look like? What's the initial adoption look like at the low level? Are there specific features of Copy.ai where someone's picking it up to write emails, another to do sales? Is there a typical way people enter into Copy.ai, or Copy.ai enters the organization?
Interview
How has the generative AI space evolved since we last talked in late 2022 and especially since the launch of ChatGPT?
A lot of the things that we predicted have come true. When we first started Copy.ai we took the Amazon/Jeff Bezos approach—what's going to be true 10 years from now? The AI models are going to get cheaper, faster, and better, all of the same time, and it's going to happen in stepwise function.
Meaning a new model gets dropped, and boom, suddenly, completely new things are unlocked. It’s kind of similar to previous internet waves, where it's like ‘oh, a piece of hardware came out suddenly, completely new things were unlocked,’ but here it's models, and it's from multiple companies. We knew that there was going to be more than one model company that would catch up to OpenAI and compete with them. That came true, that was Anthropic—as well as Google, to a certain degree—and then there are some open source ones that are starting to really catch up.
Facebook taking a big open source stance was a little less expected than I would've imagined, but knowing Zuckerberg and understanding his thinking, it makes a ton of sense. He wants to bring that cost down. He sees that as a really great opportunity to change the game there. In terms of generative AI, what was a little unexpected was the pivots into AI chatbots. In our eyes, it never really made sense long term that it would be the best interface. It makes sense that it was the one that captured everyone's imagination, just because humans are just so used to conversation. But it paints an implicit feature that you're going to be talking to AI bots a lot in the future, and that's just not a very compelling feature. I don't want to be spending 90% of my time working by talking to an AI. So we're still focusing on: What is the future that we're excited about?
Funny story—where we are today is a lot closer to the original founding vision of Copy.ai than the previous app. Before Paul and I started Copy.ai, we were imagining what we call the “business business.” What's the business that will create businesses? That would help people create businesses, and that would help people create multiple businesses? One thing that we were passionate about is entrepreneurship, and we're passionate about it because we want to see technological progress move forward. So going back to the first principle, it's like, ‘Okay, well why aren't there as many of these companies? Why isn't technological progress being pushed? Is it a lack of creativity?’ To a certain degree, but not really. I bet you if you talked to 10 of your friends—they're all really creative people, they all have ideas—but it's just way too much work. And even people who have spent the time investing and putting time into building a product that they really love, and that they think is really good, they get hit with a wall and say, ‘how do I sell this? How do I market this?’ That's been a huge issue.
If you look at the public companies, you're spending 40% up to 60% of your costs on sales and marketing. It's like, that just feels really inefficient. Instead, you should be spending a lot more money on R&D and constantly improving the products. When GPT first came out, we started focusing on the initial use cases and where the market pull, and the market pull was just a simple wrapper, the market pull was just literally getting words on the paper. Today with ChatGPT, with Google, with Facebook, and all these other large players entering the space, that's going to be commoditized, and we recognize that even the last time we chatted. We already started investing heavily into what we're calling “the workflows platform” or “platform” in general.
Our learning on it was, okay, so there's two ways that work gets done. One is really creative work, which is divergent. You can argue that the current chatbot interface is kind of like that with ChatGPT. It's not creative in a traditional sense, but it's divergent. Every time you talk to it, you'll get a different response for the most part. Then the other type of work is really repeatable manual work. It's like, ‘okay, now that I've figured out this is my process, when I have this transcript, I need to extract these things. I take these things and then I write a blog in this specific structure.’ Or for sales use cases, it's like, ‘hey, I have an email for a prospect. Now I need to go look at their website, analyze who they are, what they do, how they work, what their deal size is, and then we create a custom pitch.’
Those are very repeatable things, and that's what AI is actually really good at. It's able to take that process and then where our platform allows you to formalize that, it becomes a pipeline of work that just happens. Over time, it can be optimized, over time, it can change, but that becomes what is a true workflow inside of a business. That's extremely scalable. Then we realized, ‘oh, well, if we can build these workflows and if we can get the best ones in the world for these workflows, then we can deploy them to every other company with a click of a button.’
Traditionally, in order to get one of these workflows working you have to deeply understand it. The way to deeply understand it would be to read all the blog posts that one of these companies might put out about it, it might be to hire the person themselves. Silicon Valley does have a similar—it's not viable, but it's a similar model—where a lot of experiences are passed down only by working together. So much of it just doesn't even actually get written about. I'd say the last 10 years, content marketing and other stuff has improved that, so a lot more has been written about it, but there's still so much of the deep understanding of how something works that doesn't get written about. Which is why all the startups try to hire the person who did this at the other startups.
We realized, ‘oh, well the next phase in that is, hey, you're able to build this workflow now. You can make this and copy it into every other business who needs it.’ That's where we're starting. Hopefully that gives a high level view of how we've seen the space change from ChatGPT and purely chat interfaces, to the agent interfaces today where it's still similar to ChatGPT, but it's still a little divergent. You're still chatting with an AI, to what we're calling more repeatable platform workflow use cases, which are deeply integrated into your business.
Can you talk a bit about the transition that happened at Copy.ai from this more prosumer-type product to enterprise?
We've always known at some point we would need a zag and we told an investor this. It's like we're zigging along and we know that this industry is going to be really different and it's going to change rapidly, and there's going to be a pivotal moment that enables what we're really passionate about, which is the business of being able to orchestrate much larger tasks. That moment came about probably two months before we last chatted in November 2022. The realization was that, ‘oh, this initial product is going to become a commodity and it's not where we want to play. It’s going to just be an uphill battle.’
I believe one of the things that came out was Notion AI. It was their marketing materials that they started launching the beta and we're like, ‘oh, okay, all the applications are going to integrate this in one way or another. It's not super-defendable. Those larger platforms are going to be able to add this in and we would be in trouble.’ So we started investing at that point in time into our new platform. And we still kept the prosumer-focused stuff going because it was generating revenue. It was growing until probably a little after ChatGPT came out. ChatGPT was free for the first six months, and that wrecked havoc on our business for a bit. I think it wrecked havoc on a lot of our competitors’ businesses as well.
That being said, because we’d already started making that investment, and we kept the team small, we were able to iterate really rapidly and change our focus quite quickly. It doesn't mean that we're not doing marketing and writing tasks anymore. We're just doing larger ones. Instead of writing one single email, say, ‘hey, let's help you do the research for this person and help you write the entire sequence. And then while we're at it, we'll send it to your CRM, HubSpot, Salesforce, whatever you want to call it.’
That stuff really excited us, it was the only thing that really made sense to us in terms of unlocking real scaled volume. You’re just straight up limited by the number of times you can click the generate button as a human. But when you're integrated into Salesforce, every single new lead that comes in has a personalized email that's well-researched immediately ready for that. That made a lot of sense to us. That made it where it's like, ‘oh, wow. In the future, if somebody wants to start a business, they can just install that, and that's one of the best workflows that normally you just fill by hiring a lot of people.’ It doesn't mean you don't hire the people. Now those people can be spending their time instead of doing the same manual task over and over again, they can explore and find all different types of ideas to resonate better with their customers, to message them better, and to optimize these workflows.
So instead of working at the manual clock on the wheel level, you actually become more of the conductor level with a bunch of these AIs working for you. Our perspective on the market is the models finally got good enough to be able to do this. So even in November 2022, GPT-4 wasn't out yet, that came out in around March of '23, and we hit that timing perfectly. Our platform was just built, and then GPT-4 came out. It was like, ‘oh, great, this is amazing.’ But the one thing we ran into was it was really slow and really expensive. A month later, Anthropic launched their first cloud model, which was cheaper, faster, and still really high quality. We're like, ‘great, we'll use that one.’ And then we used that one for a bit, and then GPT-4 suddenly came out with GPT-4 Turbo and it's suddenly a lot cheaper, a lot faster, and even smarter. It's like, ‘oh, great, well use that one.’
So we've been riding that wave since, and it's like… this is the worst, slowest, and most expensive it's ever going to be. These prices will go down and the speed is going to increase. Building this platform that basically can utilize these models in a really deeply integrated way is going to benefit from all of those tailwinds. We ended up going a level above trying to build a platform and we’re now multi-model, so we don't really care who the model provider is. If a really good open source model comes out, we can implement it on our platform immediately.
We also give it access to a lot of external tools, so scraping the web, internet, and searching the internet, you're able to find open job descriptions. There's all kinds of really, really valuable sources of information on the internet. We can take those, synthesize them and then do something with them. Whether that's actually generating an email, saving it to Salesforce, or brainstorming how to even approach a sale. The same thing goes for content marketing. It's like content marketing comes from the source of the truth. We have our own internal workflow where we take one of our sales transcripts and extract the interesting topics from that as well as the unique insights from it. Then you use that information to generate an entire blog. We get about 20 new blogs a day with a content team of one person. Which is absolutely insane.
They just pick the one that they like the most that day, or they have a list of keywords that they're targeting, and they find the one that hits the keyword that they're going for, and they take that, polish it to the final little bit, and then they’re able to publish a blog post a day. That's a complete game changer. Historically, at least three years ago, four of those types of blogs would cost you $25,000 each—so call it $10,000 to get four of those blogs. Now we get 20 of them every single day, and we're able to publish one a day with a single person who really just is able to grab the best of the best.
Where do you see the potential remaining for consumer products alongside ChatGPT?
We're seeing the search engine moment in the chat app world, where basically everyone's trying to compete for eyeballs, and every single chatbot kind of offers a slightly different value proposition. Depending on the value proposition, do you prefer one chatbot to another? It's kind of like with search engines, there was something similar. In this case, ChatGPT was definitely first to market. However, they're pretty large, and they have their own foundational model, which is really, really powerful. But they’re also limited by what they can do because they're a larger, well-established market leader that everyone knows has billions of dollars.
I do see some companies, specifically Perplexity, that can come up with a better interface and offer a value proposition that’s completely different. Which is like, ‘hey, we give you really accurate data along with the sources.’ That’s a game-changer, versus OpenAI tended to be pushing more and more towards, like ‘hey, let's make this chatbot do all kinds of crazy stuff.’ It's like, ‘now it can run code, I can do all these things,’ which is also valuable, but the vast majority of people don't really need that. What everyone does want, though, is accurate answers and that's not going to change. So the way Perplexity has entered that market is absolutely fascinating. I've heard from multiple people that they actually preferred Perplexity results.
The funny thing is, you can use Perplexity with OpenAI on the backend, so it's like this friend/enemy frenemy relationship that's really, really fascinating. I anticipate this is going to continue. If a company wants to compete in that space, they're going to have to create a chatbot that's pretty specific for a very given use case. However, from the consumer-prosumer side, I think Perplexity will be able to really hold their own against the ChatGPTs of the world and the Claudes of the world. But they're all offering slightly different value propositions. Hopefully that makes sense.
We're seeing this pattern where people are using their own models more and more, but multiplexing with e.g. GPT-4 or Claude for higher-value use cases like generating long text. Do you divide up your use of models like this at Copy.ai?
We haven't focused too much on the fine-tuning yet in terms of the platform], however, what we have done is fine-tuned models for the customers, and that's a really, really interesting value proposition. So we have this workflow platform that helps you orchestrate all kinds of workflows. Now, what makes that even better? Oh, if we can create a model for your business that's specifically tuned to your parameters, and that one has worked really, really well. It's literally customized. I believe that workflow is one where there's a bunch of categories, and then given a website, it tries to find the best category to put it in. But those categories have to be exact and it has to match the ID of the category inside of that company's CMS.
So we're able to do those kinds of workflows that are super-personalized and customized to that company, really rapidly actually. That was a very fast project for us. It's even faster to deploy it as a new action, that they can use as a building block, and now they can use that same model anywhere they want throughout their entire business. In terms of the larger models, and more general things, we have a perspective that the models are improving so quickly, it's not really worth the time to fine-tune at the moment. Because today, if you fine-tune something, suddenly tomorrow, something better and cheaper might come out, that you might as well just switch back. So, we’re going to ride that wave for a bit.
However, the way we built our platform, with modular building blocks, means we have access to data that is modular and segmented. Meaning that it's really easy to grab really, really high quality data for a given task and fine-tune it into its own model. Before, it was a little harder for us, when somebody was using this data to generate blog titles, and somebody else is using this to generate emails, it's a little harder to really pull out exactly what you need. But when it's modularizing it, each given building block has a specific purpose. You can suddenly do it. For example, on our platform we have a workflow that goes and fetches a company's earnings call, a public company's earning call, and then extracts three growth areas and three struggle areas from that earnings call. Today, we use a pretty expensive model for that. It's a very long context window and it's a lot of context.
However, every single time that thing gets run, we're building up a training data set that now we can use to train a new model that does that job specifically. ‘Hey, this is the earnings call extraction model.’ And we've seen the prices, they can literally drop 10x from where they are today, and those are benefits that'll be valuable for our entire platform. For every single customer, they would immediately get that benefit. Those are the really exciting improvements that we see coming in the future, where when we start focusing on enhancing our margins, or improving the value to our customers, we'll be able to do insane price drops like that as well and pass them on to our customers.
Can you talk a bit about what you're seeing in the enterprise around adoption of AI? In general, are you seeing big companies (that you wouldn't have expected in 2022) interested in adopting new go-to-market platforms?
Great question. All the logos on our site, they're real enterprise customers now where we've talked at pretty high levels, if not the C level, at those companies. And they're real deals. They did start small, we usually land with a single use case and usually it's something that's a layup. Really, it's like, ‘Hey, we have this problem, can you help us solve it?’ When we were starting to build this entire enterprise platform, we hired a solutions guy, his name's Rob, he's incredible, and he started selling it before we even had a product, before the platform was ready. Basically, he used it to manually solve some of these large company issues. Once they get the platform vision, they get it. They're like, ‘oh, wow, I can customize this to anything.’
The extremely large enterprises are especially interested because they have very, very unique needs.They have various old systems, sometimes. They have very unique data sets sometimes. They have very unique problems. So for example, like Siemens and Thermo Fisher, they have thousands if not millions of products, and each product has a different vendor sometimes. And for each vendor, they have a different data sheet. And it's like, ‘okay, well how do you format this data sheet into our standardized format?’ That's a perfect job for AI. ‘Hey, given this data sheet, format it to this specific spec and then send it into our CMS or our PIMS, our product inventory management system.’
Those are the use cases that are extremely valuable. They hire entire teams to try and just do that manually. But now with generative AI, they can do that so much faster and so much better. Now, it doesn't mean that they're letting it run willy-nilly, they still have a human loop process to make sure that the data is accurate and valuable, but man, as these models get better, it's faster and faster. They have to make less and less changes. We've already seen that happen with the difference between the initial GPT-4, or to Claude, or to the GPT-4 Turbo.
Selling to them is a little different. Some of these still came in very much PLG, where they came in as an enterprise, they started exploring our platform, we get them on a demo, we show them, oh, within the demo itself we show them we can solve a problem. That gets them really interested, and we share the platform vision and suddenly they're like, ‘wow, how do we get started?’ That's been working really, really well. And now our thought is, ‘okay, well how can we help standardize this a little bit more?’ What are the use cases that everyone would need and really want? How do we start with those instead of custom-building it for an enterprise? How do we really go at scale? How do we win the mid-market as well as the enterprise at the same time?
For us, we were early, we invested a lot into SOC-2 compliance and into GDPR compliance. So we are enterprise ready with real enterprise customers and enterprise contracts, and it's given us a huge advantage. A lot of these companies really love the platform vision because they find themselves bloated with a lot of different tools. If you look at the entire AI tool landscape there, there are going to be thousands if not hundreds of thousands of these tools. It's going to be procurement hell if you're going to buy point solutions for every single need.
That's why we sell the platform vision, to get rid of that. Now, on the go-to-market side in particular, a lot of companies are facing go-to-market bloat, which is like every single tool has its own data silo that does a very specific thing, and that was a leftover from the zero interest rate era. Everyone could buy a tool just to solve this single problem. Now, they're looking to consolidate these, and a lot of them are starting to realize, ‘wow, a lot of these tools end up being just a workflow on Copy.ai.’ And not only that, it's better because now they can customize it to their specific needs on our go-to-market platform as well.
Let's see, the question is to what degree is the AI boom opening up big companies to adopting novel software from startups? Yes, they're the big push, especially from a top down approach. A lot of these companies are getting a mandate from the top, ‘hey, how do we use this and how much ROI can it actually create?’ A lot of people have no idea where to even start. When we get on the demo, we show them what's possible, suddenly they're like, ‘wait a second, I can actually take this workflow and turn it into an ROI in numbers.’
Versus, ChatGPT—or any of these chat apps—great. Every single person at the company has it, and they all work 20% faster. What's the ROI on that? It's really hard to calculate what they’re actually using that other 20% of the time for. Here, it's like, ‘hey, we have this backlog of product descriptions that need to be written. If we use Copy.ai, that thing is accelerated by three months.’ It's a very, very hard core ROI number that they can adopt. What are the core challenges? Well, the magical part about generative AI is that it’s so customizable. With a single small prompt tweak it can change the entire output, and you customize it to exactly what you need.
But that's also one of the bigger challenges. Historically, software forced companies to fit into their workflow. So it's like, ‘hey, this is how our software works. If you want to use it, you have to put your information in here. You'll get this data back, and then you have to figure out how to put it into your own system.’ With generative AI, suddenly it's like, ‘wait a second, what do you need? We can modify our workflow to your needs so it hits exactly what you need.’ But that modification process is still a little bit harder. Some other ways it's been talked about is that enterprise software is typically the same across the board, but people like to have tweaks around the edges, and that's where a lot of the complexity comes up. With generative AI, it helps handle that complexity. But the complexity of setting it up and actually setting up those customizations is still a little hard.
And the fact that these models are not non-deterministic, they have a probability of going a little bit off the rails and whatnot. It's a challenge. We have to help them build those guardrails, whether they feel really, really highly confident that, ‘hey, this workflow, it's going to be consistent whether you throw in one product description or a million product descriptions.’
It seems like Writer is going hard on this idea of being the Grammarly for the enterprise, while Jasper is drilling down more into the marketing use case and Copy.ai is a little horizontal across the teams involved in go-to-market. How do you see these different niches evolving and how do you see them developing?
Great question. Our perspective on this is that adopting AI should really increase your company's revenues. If you adopt it and revenues don't improve, or if cashflow or your margins don't improve, you're not doing it right. That's why we call it a go-to-market AI platform instead of just sales or just marketing or just content creation. Those tend to be a little bit upstream. Our job as a go-to-market AI platform is to help you increase your revenues. Hit that go-to-market velocity. Go faster, better, improve outcomes.
What that leads to, though, is a unique set of challenges. It's like, ‘okay, well how do you do it for a company?’ There's some standardized use cases, and then there's some really personalized and customized use cases to a specific company. Jasper, from what I understand, is going after the AI marketing copilot type of stuff, where it's like, ‘hey, you are the different blog posts you can be creating.’ Versus, Writer has been very much also geared toward the marketers, but to be on brand and on tone, that's what the CMO really cares about.
We are targeting more of the CRO-CMO area, I would say. The CROs care about, ‘okay, well how do I go end to end for the customer experience journey to be as optimized as possible?’ Generative AI allows that type of personalization. Sales, in general, is when your product is able to solve one of the top three pain points that some other company is facing, right? If it's not a top three pain point, you're probably not going to get a sale. So it's like, ‘okay, well, what are the top three pain points of a company?’ You open up every single CRM at the average company today, and it's probably most empty. You open up ours, and hey, for any given company, we've done all the research, we've done the heavy lifting.
If it's a public company, yeah, we've read the latest earnings call, we've read the latest 10K, we've found any CEO interviews recently that talks about their focus, their target area, where they're investing, and what their goals are. From that, you're able to actually take that, and position your product for that specific company. So a great example of this would be Mongo, they're trying to increase their international presence. The brainstorming that came out of that was like, ‘hey, we can help you translate your sales enablement material into different languages. We can help you translate all the support docs into these other languages. And we can help you really implement a targeted focus of finding lookalike customers for international companies.’
It's extremely on-point things that suddenly make a lot more sense. If this company wants to go international, these are the pain points, these are the things and the solutions that you should be talking about. That happens for every single opportunity, every single account and every single contact that signs up. We're able to get all that context. It exists somewhere on the internet and we bring it into your CRM and personalize it for your business. That makes it so every single SDR, every single AE at your company is set up for success. If you're given a blank CRM, how are you even set up for success? You're just not even able to start. But from that data foundation, you're actually able to do some very, very interesting things down the line. Which is that, suddenly, after a sales cycle with all this filled up information, you can start finding patterns. Hey, what were the big problems that actually led to conversion rates that led to actual sales? What did this thing achieve?
Which case studies would actually make sense for this new customer that's coming in? Can we speak to them about their problems in such a deep way that they think that we fully understand that problem? And that's the customer experience that would really transform an entire go-to-market organization. If every customer feels like, ‘oh, this is the perfect solution for me and my needs today. And that's what we're helping our customers replicate, and we're doing it ourselves internally, and we're still very early in this journey. There's just so much more opportunity here, and literally, whatever you can imagine you can do now. It's all around the creativity of who can create the most creative workflows to improve that customer experience in such a deep way.
One thing I wanted to circle back on is you talked about the PLG motion that you still have with some of the enterprise customers, and you mentioned the CMO-CRO region is where you're really selling to. What does that look like? What's the initial adoption look like at the low level? Are there specific features of Copy.ai where someone's picking it up to write emails, another to do sales? Is there a typical way people enter into Copy.ai, or Copy.ai enters the organization?
Typically what happens is someone a little lower on the totem pole signed up because they have a problem. It's usually around account research or writing cold emails or something along those lines. And then they find that we have these pre-built categories, our pre-built workflows. And these workflows do everything from finding phone numbers, analyzing earnings calls, to finding prospects at a company, all kinds of enrichment workflows and whatnot. So they use those manually, and it's like, ‘hey, I have a CSV list of 100 accounts. Great, I'm just going to upload a CSV of these 100 accounts to research them.’
And now, they suddenly did the work that would normally take weeks to do. It's like, ‘hey, for every single account, go and find out what they care about.’ Then, the best part is you can actually even add a lead scoring component to it, which now it's like, not only did you do the research, but you also had AI brainstorm out of these 100 accounts, which are the best ones to start with, and then you focus your energies on those. That's a really valuable use case just right off the bat. People are constantly getting new territories and people constantly get new accounts and have to prioritize. So being able to figure out how to focus your time on the most valuable ones is just such great value out of the box.
The second part of that, though, is they'll actually introduce us to higher up leadership. So it's like, ‘hey, by the way, you should talk to this person. They're our head of sales or VP of revenue or VP of RevOps’ or something along those lines. Suddenly, we're able to get them on a pitch. We show them what this person is doing and what the best processes are, and they're blown away and they're like, ‘wait, I want that. I want that.’ That really gets us a foot in the door, and then we're able to land a deal from the higher up. And now every single rep has access to that workflow directly needed inside of their CRM. They don't have to come into Copy.ai. Now, it's just work. It's just like, you open up your CRM and it's filled. It's filled with really valuable information.
You don't have to go out and Google, and search, and look up all this information, and analyze it and brainstorm around it. The best SDRs spend hours upon hours doing research before writing that email, and it's all to optimize that first two sentences. We bring all that research together into your CRM, with potentially even a draft of that first email, and the SDRs are just a lot more effective and a lot more competent. The customers love it because suddenly the outbound emails are just a lot more personalized, and a lot more relevant, and usually a lot more valuable, because they usually start with a huge value.
Disclaimers
This transcript is for information purposes only and does not constitute advice of any type or trade recommendation and should not form the basis of any investment decision. Sacra accepts no liability for the transcript or for any errors, omissions or inaccuracies in respect of it. The views of the experts expressed in the transcript are those of the experts and they are not endorsed by, nor do they represent the opinion of Sacra. Sacra reserves all copyright, intellectual property rights in the transcript. Any modification, copying, displaying, distributing, transmitting, publishing, licensing, creating derivative works from, or selling any transcript is strictly prohibited.