Andrew Yates, CEO of Promoted.ai, on when marketplaces should layer on ads
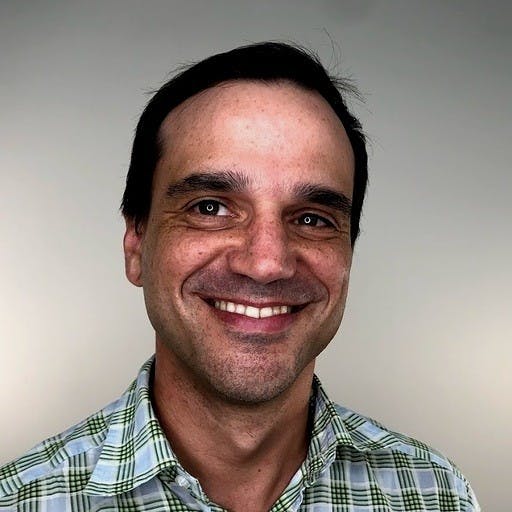
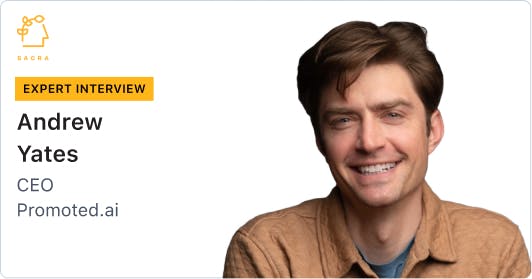
Background
Andrew Yates is the CEO and co-founder of Promoted.ai. We talked with Andrew about the role of AI in optimizing marketplace efficiency, the challenges of layering on ads as a monetization strategy, and what marketplace companies should do before they start thinking about adding ads.
Questions
- Can you start by telling me a bit about your background and Promoted.ai's background?
- Promoted.ai is not about enabling ads per se, although you support ads rollouts. It’s about marketplace search and discovery, and the underlying optimization and measurement systems. The question of ads versus increasing ARPU in other ways is tactical. Increasing ARPU is the goal.
- Why the focus on marketplaces specifically?
- When you start working with a new client, how do you frame the optimization system you're providing and the concrete benefits it will drive for them?
- There seems to be an implicit sequencing to what you're saying, which might not apply to every marketplace, but in general you really need to maximize your core take rate before layering on ads. Is that a fair characterization?
- That seems to also imply that ads are an option that comes later in a marketplace's lifecycle, once it has reached a certain level of maturity and scale. What signals do you look for that a marketplace is at the point where ads could be a viable option to layer on top of the core transaction model?
- Shifting gears a bit—you mentioned earlier that it takes a while for sellers to really see the ROI they're getting from ads on a marketplace?
- Obviously AI is a huge topic right now. Can you share a bit about how you see ML and AI shaping this space, beyond what you mentioned earlier about it enabling incremental gains?
- You mentioned your platform has a unique approach in terms of letting customers bring their own models and combine them with yours. Can you explain why you think that's the right approach vs. a one-size-fits-all model?
- Switching gears, what other key trends are you tracking in the marketplace and e-commerce platform world as it relates to the topics we've been discussing?
- That's a good segue to another topic, which is build vs. buy. I'm sure you get asked about this a lot. Why should marketplaces work with a company like Promoted vs. building these capabilities in house?
- Anything else you want to share with our audience before we wrap up?
Interview
Can you start by telling me a bit about your background and Promoted.ai's background?
I'm Andrew Yates, the CEO and co-founder of Promoted. I started it with my co-founder, Dan Hill. We were engineering leaders together at Pinterest, working on Pinterest ads. Prior to that, I worked on machine learning for ads at Facebook in engineering and data science roles, and Dan worked at Google, also in ads engineering.
When we originally started Promoted, there were two aspects to our thinking. One part was from a market perspective, and one was from a passion for the technology. The passion for technology part is easy—we really enjoy building these types of optimization and measurement systems at scale.
We thought we could do a better job ourselves, so we assembled some of our best colleagues and former reports, and we did it. We really enjoyed this—we just like building these types of systems and thought we could innovate further.
From a market perspective, people who have that interest, skill, talent and experience like we do are very valuable. We thought, well, we know the systems are also very valuable, so would there be a market for this at the top level? There are already many vendors and opportunities if you're just getting started or can't afford an engineering team or that's not your focus. But at the top, meaning you're at least a public company or almost public and doing billions in GMV, you can afford whoever you want. It's not a matter of budget for engineering R&D, it's actually accomplishing this and making it increase revenue per user sustainably, which is very difficult. If we did that and had a system to do that, what kind of company would that be? So that was our thinking.
Promoted.ai is not about enabling ads per se, although you support ads rollouts. It’s about marketplace search and discovery, and the underlying optimization and measurement systems. The question of ads versus increasing ARPU in other ways is tactical. Increasing ARPU is the goal.
Yes, exactly. Reframing it a bit—everyone sees Promoted.ai as being about ads. A lot of times people even get confused and think we help them buy ads on Google and such. But our customers' actual goal usually isn't ads. They may say "we want to build an ad network" or "we want sponsored search." But that's not really what they're trying to do most of the time. What they want is for average revenue per user to go up. They want to be more profitable.
If you can accomplish that without creating a whole new line of business that complicates your app and org structure, that's success. I mean, that's ultimately what we've learned and what we've delivered on. We took technology and concepts from performance ad systems built by first-party platforms like Pinterest, Facebook, and Google.
Performance ads are all about optimizing for incremental value. Did the user engage? Was it real engagement or some low-quality interaction? Did it lead to a valuable action? Was that action adding to cart, purchasing, booking something?
It can get super complex. For example, with a freelancer marketplace, you have to track things like: did they send an offer, did the offer get accepted, did the job get completed successfully, was it a big or small contract, did the user come back and engage again? So it's about measuring the complete path of value and correctly attributing it to the engagement on your platform.
This is what Google, Facebook, Amazon have invested in heavily. They call it "performance advertising" but really it's just measuring value correctly and optimizing for it. We're applying those concepts to search and discovery for marketplaces and e-commerce. You can layer ads on top if you want to get into the competitive take rate aspect. But it's not required to drive a lot of value.
For bigger marketplaces like Wayfair, they were doing a very large amount in ads already. We started out working on just the ads piece with them. But you don't have to do that. Ultimately, the goal for marketplaces and e-commerce platforms is increasing ARPU. And usually the fastest way to do that is by taking your existing product and making it better, not by introducing a whole separate line of business that theoretically will make more money.
Why the focus on marketplaces specifically?
Because they're complicated. We have a technology advantage, and there's not much to optimize if a business is very simple. If you're just a straightforward commerce website with limited inventory that's not changing much, you don't need the greatest tech and there are many reasonable solutions out there. But two-sided marketplaces get complicated really fast. Even at a medium scale, it becomes a very difficult search and discovery problem, with challenges around trust and safety. The take rate and adversarial dynamics come into play too.
Our grand vision is to connect every buyer and seller across every app. But with marketplaces there's such a power law distribution where a few winners dominate and the tail drops off quickly. So we have to build technology that services the biggest players, or else we're missing almost all the market for this product. Marketplaces allow us to refine our technology on a challenging problem while still being able to start somewhere as just two people.
For online two-sided marketplaces in particular, that's where the lines crossed for us to get started. As we grow, we're able to service huge customers like Wayfair. Wayfair isn't usually thought of as a marketplace, but there's a lot of overlap between two-sided marketplace dynamics and performance advertising. The technology, measurement, and concepts are similar. So that's why we focused on marketplaces.
When you start working with a new client, how do you frame the optimization system you're providing and the concrete benefits it will drive for them?
The proof is in the numbers—run an A/B test and see if revenue goes up in the treatment vs. control group. It's that simple. You can also look at proxies for revenue like number of users who purchase or metrics specific to the marketplace. That's how you know it works.
The overall business impact then depends on how much of their activity flows through the surfaces you're optimizing. If it's a large percentage, like if most of their transactions come through search and discovery, then improving that by “X”% flows through to overall ARPU.
Not only do you see the immediate improvement in unit economics, you get secondary benefits in terms of how the product feels and works. When you match buyers and sellers more effectively, sellers invest more in the platform over time because they're seeing results. Buyers have a better experience finding what they need and completing successful transactions. Those compounding growth effects are harder to measure in an A/B test but it's an intuitive flywheel—of course it's going to help the platform grow if you're making both sides more successful.
I bring this up because this is where the advertising piece can start to break that virtuous dynamic. There are good types of ARPU growth, where people find what they're looking for and buy more, or sellers connect with more buyers and make more sales. But there are less healthy types of ARPU growth that don't really make the core platform better.
We've all seen examples of apps getting overloaded with ads in a way that clearly degrades the experience. “Enshittification,” or whatever you want to call it. Sure, maybe revenue per user goes up temporarily, but no one really believes it's the kind of sustainable growth that will make the platform better long-term. It's more like taxing people's existing habits until they get fed up and leave. When we work with customers, we try to help them focus on the healthy, compounding types of ARPU growth first.
Then there's the question of whether you're actually charging a fair price for the value you create as a platform. In the beginning, a lot of marketplaces have to sell at a loss, or at least a very slim margin, to get up and running. But eventually you need to be able to capture more of the value you're creating. Easier said than done.
What we try to help customers think through is—you need to know exactly where each incremental dollar you're trying to capture is coming from, and it should be done intentionally. Ideally it's coming from a clear theory of value generated. On the seller side, that could be—we've driven X amount more volume for you, so we're going to charge Y amount more in take rate, but you're still net positive. Or we've provided new tools and capabilities that let you be more competitive. The platform needs to be able to articulate what it's providing in exchange for a higher take rate.
I think where marketplaces often get in trouble is when they skip straight to "therefore you owe us more money" without being able to back it up. That's when you get into dangerous territory, especially if sellers are still ramping up and don't have enough history on the platform to really evaluate the ROI they're getting. If you've overpromised, eventually that comes back to bite you. Not necessarily this quarter, but within a year you're going to have to answer for it.
There seems to be an implicit sequencing to what you're saying, which might not apply to every marketplace, but in general you really need to maximize your core take rate before layering on ads. Is that a fair characterization?
Yes, I think so. And why not just raise the take rate? You can literally just go into your system and increase it by a few percentage points. Either people will accept it and you're done, or they'll say "we can't do that, our sellers will leave the platform." That's when it's time to start thinking about ads.
If you've hit the ceiling on take rate and literally cannot increase it further without sellers leaving en masse, then you either need to revisit your business model or explore other monetization options. There's usually a good reason why you weren't doing ads when times were good. So if the finances take a turn and suddenly it's "we need ads ASAP," without really revisiting how it fits into the overall ecosystem ... good luck. Those original concerns are probably still valid.
That seems to also imply that ads are an option that comes later in a marketplace's lifecycle, once it has reached a certain level of maturity and scale. What signals do you look for that a marketplace is at the point where ads could be a viable option to layer on top of the core transaction model?
The main one is—if a seller stops selling on your platform, are you truly okay with that? That's the litmus test. If not, people ultimately won't pay your ad prices. They'll just refuse to sponsor listings and you'll have to keep displaying their products anyway. You need enough real competition, where the platform is comfortable showing alternatives, for ads to work. If you're not willing to let some sellers walk away, why should anyone pay to sponsor? There has to be that credible threat for the ads flywheel to spin up.
Shifting gears a bit—you mentioned earlier that it takes a while for sellers to really see the ROI they're getting from ads on a marketplace?
Let me clarify that. Improving match rates and relevance will immediately lift your platform's unit economics. That's provable in an A/B test and it continues delivering value over time. There are harder-to-measure secondary effects, like sellers being more bought in because they're seeing more success, or buyers engaging more because they learn that search works reliably. That type of incremental growth compounding is abstract and plays out over a longer period. But the core matching efficiency gains are evident right away.
What I was talking about with ads taking time is—look at how most sponsored search is sold today. The story is spend money on our platform and you'll get 5x ROAS [Return on Ad Spend], or 20x ROAS, or some other huge number. Here are all these case studies and so on. But for the individual seller, it takes a while to really see that play out, especially for considered purchases or offline sales. They have to run campaigns, wait to see results, hope it matches what was promised. And on the platform side, you usually have whole sales teams dedicated to nothing but convincing advertisers to keep spending more.
Anyone who has experience running digital ad campaigns knows this is challenging. Even the most sophisticated advertisers in the world, spending on the most mature platforms like Google and Facebook, often have dedicated teams and resources to validate that they're actually seeing the promised returns. It's a constant process.
Now imagine you're a marketplace doing a few billion in GMV, which sounds big but is small compared to the giants, and you're trying to build an ads business from scratch. Attributing value and ROI is going to be at least as hard as it is for Google and Facebook, if not much harder. You have less data, less mature tools, your own platform quirks to figure out. Of course it's going to take a long time for sellers to really buy in.
And this is what we emphasize to our customers - convincing people of the value of your ad products long-term is already a very heavy lift. If you're not completely certain you're delivering that value, down to your core, it's going to be nearly impossible. If you harbor any internal doubts about the ROI you're driving, you're probably not generating as much value as you're selling to advertisers. All roads lead back to focusing on actually creating value as priority one.
Obviously AI is a huge topic right now. Can you share a bit about how you see ML and AI shaping this space, beyond what you mentioned earlier about it enabling incremental gains?
Machine learning has been driving compounding improvements in this domain for a long time. I don't think of the current AI buzz as a single step change so much as a continuation of that progression, albeit an exciting one. We absolutely use ML and even large language models to better predict user intent—taking behavioral data and external context to match queries to relevant products in smarter ways. This absolutely enables better, more "magical" search experiences.
But it's not a silver bullet that will transform everything overnight. There are good reasons why the Amazons of the world aren't suddenly shifting to a completely GPT-powered search experience. The models are still expensive and slow to run, and their existing search UX is honestly good enough for most needs. Over time the efficiency and costs will improve to make more ambitious applications viable. We're already pulling some of this into alpha tests and pilots. But it's an incremental boost to the core job of modeling user behavior and predicting the next action.
Some of the AI hype right now, while exciting, misses the real unlock in my opinion. We went from a world where high quality, open domain, zero-shot learning was considered impossible to one where it's very doable, just computationally expensive. Expensive and slow are barriers that tend to fall over time with focused engineering. So it's a huge deal, but more in a "we can now do powerful new things" way vs. an imminent singularity. It practically matters a lot for teams like ours who obsess over this stuff. Over time it will enable all sorts of product innovations. But it's not an overnight revolution, it's a significant increment on top of the core machine learning work that has been quietly driving a lot of the gains in this industry for years.
You mentioned your platform has a unique approach in terms of letting customers bring their own models and combine them with yours. Can you explain why you think that's the right approach vs. a one-size-fits-all model?
I think there's often a misconception, even among pretty technical folks who aren't deep ML engineers, that there is "the model." The reality is that any sophisticated search and discovery system involves dozens of models developed by different teams, constantly being updated, working together in various ways.
Sometimes "the model" refers to a specific point-in-time set of weights. Sometimes it's more of a general architecture that keeps evolving. Sometimes it's the overall ensemble, the whole shebang. At big tech companies, having this zoo of models created by different teams, snapped together in different ways, is just the norm. Of course there's a ton of engineering and process work that goes into making that manageable. But conceptually, model composition is a given.
A simple example: is this image high quality or not? On its own, that's not a business, but for an e-commerce marketplace it's super valuable to automatically flag low quality listings. You could even have another model that tries to fix the image if it's blurry. That's an example of composing different purpose-built models together—maybe an image quality model, a blur detection model, a photo enhancement model, and so on. Then you have other models for query understanding, retrieval, ranking, ads targeting, and so on. They're all doing different things but working together to power the overall experience.
So this concept of letting customers snap together models like Lego bricks felt very natural to us coming from big tech. We didn't originally position it as a key differentiator, it was just obvious that this is how you'd want to do it. Turns out not everyone had that context.
We believe the best search and discovery systems over time will be built this way—combining the best-in-class models for each particular job, and letting the customer control how they fit together. For some companies that see this capability as a key differentiator, they should absolutely invest in it as a core competence. But for most, it makes sense to focus on their core value prop, and snap together great models from providers who specialize in them.
Switching gears, what other key trends are you tracking in the marketplace and e-commerce platform world as it relates to the topics we've been discussing?
One big theme is this concept of "zero take rate" and how it relates to connecting 3P [third-party] seller dynamics and advertising. On some level, zero take rate is the Google model—it's free to list your products, they'll even do it automatically, but if you want better placement you pay for ads. Which is really just paying a higher take rate for incremental distribution.
For an e-commerce marketplace, true zero take rate is more of an accounting fiction because fulfillment and transactions have real costs. You could choose to subsidize things to make it feel like zero take rate. But unless you're literally printing money, that means you're just shifting the take rate to other parts of the system.
So I think it's better to reason about it in terms of—who is paying for what, how are you dividing up the economics between the different participants. If sellers want more volume, you can generate that, but it costs money, so the take rate on those incremental sales will be higher. You could choose to call that "ads" or not, but fundamentally it's the same thing—paying a higher percentage for more distribution.
The same goes for 3P dynamics and drop shipping. Part of why marketplaces like 3P is that it lets them improve selection without taking on as much inventory risk. But someone still has to do the work of figuring out the positioning, handling the actual fulfillment, providing customer support, and so on. The platform can transfer those costs and responsibilities to 3P sellers, but it's still an important part of the overall system.
With both ads and 3P, the key questions are—what costs are you transferring, who bears them, and are you still creating enough value to justify the effective take rates? Is the end customer experience actually better or are you just squeezing other participants? And do you have a bulletproof theory for where the incremental value is coming from to make the numbers balance? If you don't, you can get away with handwaving for a little while, but eventually it tends to unravel.
That's a good segue to another topic, which is build vs. buy. I'm sure you get asked about this a lot. Why should marketplaces work with a company like Promoted vs. building these capabilities in house?
It's a great question. There are really two separate issues that often get conflated. One is, should you invest in these capabilities at all or just focus on other priorities? The second is, if you're going to invest in search and discovery, should you build it yourself or buy it?
On the first question - it's surprising how often we get the feedback "sounds great, but if we work with you then we won't be building it ourselves!" To which I say—if you can build a system in 6 months that drives 10% ARPU gains, what are you waiting for? Go do that immediately! No one is stopping you. If it's important, why wouldn't you invest right now instead of in some hypothetical future?
I think what often happens is companies fall in love with the idea of having these capabilities, without being realistic about what it takes to actually manifest them. It's not just hiring a bunch of smart people and buying some AI tools. The hard part is building an end-to-end system that probably drives real results. A lot of companies seem to focus more on the narrative and implied valuation multiples than the brass tacks of shipping a reliable solution.
Now, to be clear, for a small number of companies, investing deeply in search and recommendations is absolutely the right call. It's a core part of their product and their primary differentiator. Think of the Netflixes of the world. They've made a strategic bet that world-class recommendations are essential to their business. So of course they're going to go all-in on building that muscle in-house. And they should!
But for most marketplaces and e-commerce companies, search and discovery is an important piece of the puzzle, but not the main thing. You still need best-in-class capabilities to be competitive. But your secret sauce is likely something else, maybe it's inventory, or merchandising, or logistics, or whatever. In those cases, trying to also be the world leader in information retrieval is probably a distraction.
That's where our pitch comes in. We're not a black box. Our whole approach is to be transparent about how these systems work, to help our customers build their own intuition for what drives performance. We'll even tell you exactly what to measure and how the economics pencil out. The point isn't to obfuscate what's happening, it's to short-circuit the need to reinvent the wheel.
We sometimes get asked—aren't you worried customers will just learn from you and then build it themselves? I'm really not. The handful that are truly committed to building these capabilities internally and see it as an existential priority—they weren't going to work with us anyway. And that's fine! They absolutely should invest in it directly. But the reality is it's really hard to build a great search system. Much harder than most people assume. There's a ton of embedded knowledge and infrastructure required. It doesn't happen by accident and it doesn't happen overnight.
The problem is you actually have to build it. Not just try to build it, not just hire all the people to go build it, not have a mandate that “we're going to own this.” You have to actually do it, and have it finished, and working. The last part is a lot harder than the first part. The first part is like, "It's our mission to be an AI-first company. We're done. Success." And even hiring, like, "Okay, I have $100 million, let's go hire all the people, and spend it all on Snowflake." You did it. Now what have you got? Is it done?
So for the vast majority of companies, it ends up being a better investment to focus on the things that are truly unique to their business, and partner with experts for capabilities that are more infrastructural. Obviously I'm biased, but I think search and recommendations often fall into that bucket, in the same way that most companies prefer to use AWS or GCP instead of managing their own data centers.
At the end of the day, you have to create real value for your business. Everything else is just financial engineering. The companies that actually succeed long-term are the ones that relentlessly focus on delivering great experiences and outcomes for their customers and partners. That's what we're all about—helping marketplaces and e-commerce companies measurably drive more value from their platforms. Sometimes that means ads, sometimes it means other approaches. But it always comes back to creating real value. If we can help our customers do that faster by sharing our battle-tested tools and expertise, that's a win for everyone.
Anything else you want to share with our audience before we wrap up?
I think we hit all the main themes! The key takeaway is that a lot of the conversation around ads and AI in e-commerce is missing the forest for the trees. It's not about chasing shiny objects, it's about using data and technology in a disciplined way to create more value for your ecosystem. New capabilities like large language models are exciting and will unlock a lot of opportunities over time. But the fundamentals are still the fundamentals.
Focus on really understanding your customers, your suppliers, and your business model. Invest in the capabilities that are essential to your unique strategy. For everything else, find great partners who can help you accelerate your progress. And always, always come back to the north star of creating concrete value. If you get that right, the rest tends to fall into place.
Disclaimers
This transcript is for information purposes only and does not constitute advice of any type or trade recommendation and should not form the basis of any investment decision. Sacra accepts no liability for the transcript or for any errors, omissions or inaccuracies in respect of it. The views of the experts expressed in the transcript are those of the experts and they are not endorsed by, nor do they represent the opinion of Sacra. Sacra reserves all copyright, intellectual property rights in the transcript. Any modification, copying, displaying, distributing, transmitting, publishing, licensing, creating derivative works from, or selling any transcript is strictly prohibited.