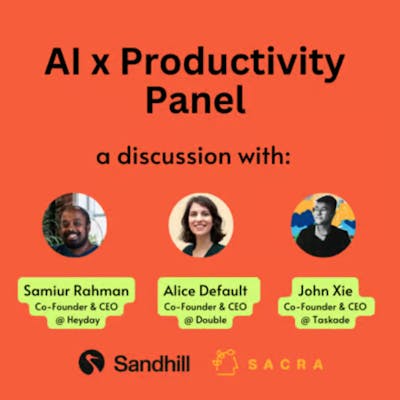
Every productivity app you use is embedding generative AI features, from Notion, Grammarly, and ClickUp to Microsoft Word and Google Docs.
To better understand how productivity app founders are thinking about and building with LLMs, we teamed up with Sandhill Markets to host a panel conversation with three founders/CEOs who have made "bet the company" moves into AI:
- Heyday co-founder and CEO Samiur Rahman
- Double co-founder and CEO Alice Default
- Taskade co-founder and CEO John Xie
Transcript below.
Adam:
As you know, if you've been here before, I'll kill a few minutes here as people stream in. If you haven't met before, Sandhill Markets does a lot of these live events. We have an alumni page, a daily-ish newsletter that's more like two times a week, and we have a big syndicate. We do early stage stuff, we do really late stage stuff the way that a normal syndicate does where we share exciting things and you get to interact with it.
Much more importantly, some of the people that helped this happen—if you don't know Walter, if you don't know Sacra, it's worth checking out. They do an awesome job introducing us to exciting founders and investors and they think really hard about private markets generally. If you haven't checked them out before, you should. They're a big part of how we stay smart about this stuff. So check 'em out.
Finally, our guests. Samiur from Heyday, an AI copilot that transforms your documents, notes and conversations into quotes. I'll let them explain better so I won't go too long. But one of the cool parts about this panel is that everyone on it has been working in the productivity space for at least a few years. Samiur, I think it's three years. I'll have them do it, but then I think six and seven for Double and Taskade, who are the other companies I'll talk about in a second. So super excited about that.
Samiur, it's great to have him. They're focused on exec coaches. I'll let him kind of explain that a little bit more.
Next we've got Alice from Double. Double gives you remote assistance powered by AI tools. I've looked at Double, I've known about Double for a long time. Super excited to hear not only how Alice has been doing over the course of the last six years of building this company, but then how AI has supercharged it. And again, she's been in productivity for a while, having been at Front before.
And then finally last, but definitely not least, John from Taskade—AI powered team collaboration including tasks and projects. And I'm interested to hear a little bit about how that's similar or not similar from Heyday. Obviously there's a lot of different companies in this space. Taskade though has been in this space again for a while. I think I'm forgetting what year they were in YC, but as far as LinkedIn's concerned, they're about seven years in.
So really excited to—I'll leave the intros there. I think I've killed enough time. We'll start to pull up our esteemed guests and get into it. Hey, nice new background, looking good.
I gave really horrible intros of each of you. I think the best way to start is to have you all go around the room, if you're up for it, to give a little bit of background on yourself, which I think is awesome. And then a better explanation than I gave of what your business does and is focused on. Samiur, if you're game to kick us off, we can get into it.
Samiur:
Sure. Thanks Adam. Thanks for putting this together and thanks for inviting me. Yeah, I'm Samiur. I went to school for machine learning and AI and electrical engineering and signal processing a long time before it was cool.
I was drawn to it because I would say I'm one of those engineers who likes engineering because it helps me automate things that I don't want to do for myself. And AI is the ultimate tool for that. So it's always been something that I've been excited about.
I've worked at big companies like Amazon, realized it really wasn't for me even early on when I worked there. And then have worked at pretty much startups since then. Most recently I've been working on Heyday, which thank you, Adam, for introducing that. I can cover that a little bit more. But I also worked on a productivity startup before that too, also using machine learning, but less so forwardly marketed with AI.
So we have a lot of learnings about productivity and how to use AI—mostly how not to do things. So I can talk about that, but thanks again for having me.
Adam:
Yeah, awesome. Alice, you want to keep it moving?
Alice:
Sure. So I've been in the productivity space for almost 10 years now. Kind of got into it by chance, and I think once I was in it, I was like, this works well. And I am very interested by just helping people get to the things that they really want to do on a daily basis, which doesn't usually include project management and scheduling and all those things.
I was the first non-tech employee at both Front, which is an email app, and Sunrise, which was a calendar app that got acquired by Microsoft. So I built email and calendar, and then went on to rebuild Outlook for a couple of years at Microsoft Mobile apps. Then left Microsoft because I was frustrated—one, from being in the corporate world, that's a different story, but two, also because I loved building productivity apps, but they weren't really making people more productive. There was still a lot of busy work. People were still not doing what they were supposed to be doing.
And so kind of took it one step further by bringing in human-in-the-loop to start Double. We're a remote executive assistant service where our assistants and our clients have access to AI tools. It used to be just productivity tools and now it's AI and productivity tools to make this delegation more seamless.
Adam:
Awesome. And I'm excited to explore that specifically—the difference between, and in my notes I said "cyborg", which I think maybe makes sense, but whether you're pure product or mix and how that goes, decisions around that. John, you want to keep us moving?
John:
Yeah. Hi everyone. I'm John Xie from Taskade. Quick background on my side as well—started building websites early days for Pokemon websites and gaming websites, just out of school thinking it was fun. Throughout that journey, startups—originally started helping out folks, even setting up servers for Counter-Strike and so on and so forth.
But I think we really kicked off Taskade—we did YC summer '19. It was the last batch that actually had an in-person Demo Day. It was really the idea of helping people collaborate across the world because the three co-founders were in different time zones. It was about creating a unified workspace where you can chat, organize and collaborate in real time together and really get things organized from start to finish.
I think what really changed the spectrum with AI is that it unlocked a lot of superpowers. Instead of working and collaborating and making sure you need your whole team with you inside of our platform, the entire cold start problem just changed because we always had video chat and collaboration and the ability to talk to your team, but now you can really just actually collaborate with AI and the whole experience changed overnight.
And I think that's where we doubled down on AI agents—the ability to not just use AI as an assistant, but how can I create a mini version of myself or even build or train an agent with my own knowledge in it. So that really changed the entire spectrum of our workspace and the way that you use Taskade. But yeah, it's been a pretty crazy past year or so and every day there's something new.
Adam:
Yeah. Well I think that maybe is a good segue into one of the first questions of this kind of paradigm shift that we're living in, or at least the "ChatGPT moment" I think is how it gets referred to. Some of you were, I guess, before that.
I would like each of you to talk about how, let's call it the "ChatGPT moment", has impacted or maybe not impacted what you were doing. What parts of it were a big shift both internally and the way you were personally thinking about it, and what parts of it were more hypey and less relevant to you?
So Samiur at Heyday, again, you've been in this world since before whatever the morning news was talking about ChatGPT, but now they are, right. So how did you see that? Did you feel validated? Were you like, "I've been telling you guys!" Or I don't know. Tell me a little bit about that.
Samiur:
No, I would say the first reaction, and I'm just going to be very honest, it was more of fear than it was of excitement. And then thankfully the lizard brain backed off a little bit. It was mostly like, "Oh, I've built my career on the skill set of being able to create automated AI machine learning products." Most of the time people don't even know that that's what is AI, it doesn't matter. It's built products that help people in a way that they can do more with less, but suddenly a lot of people can just hit an API and get the same level of functionality.
Adam:
All the things that you've been building a lot of—the magic you had is a little bit less magic maybe.
Samiur:
Right, right, exactly. So selfishly, that initial reaction made me a little bit fearful. Then obviously lizard brain backing off, I was more excited about how there were so many new ideas coming in because the amount of people thinking about automation and AI 100 extra thousand Xed basically within six months. And so that was amazing—just the level of new ideas, the capacity of people increasing hardware, investments in hardware. So things became cheaper, everything just started becoming easier and easier. So that's awesome.
Personally, I've also seen that I've been building ML products for a long time and getting it right is really, really, really hard. And it's not just the engineering piece of it, it's actually understanding what you need to do for people. You could just hire an assistant, but if you didn't direct that assistant to do the right things, they wouldn't actually be effective. So it's sort of similarly.
And so I think a lot of people are figuring that out. If they do, let me know because it's hard to get that right. And so I think a lot of people are figuring out that with AI it's really easy to build some cool magical demos and toys, but how do you actually make it really truly useful for people is not so easy.
Adam:
Yeah. It's like the management, and I'm interested to hear what Alice has to say about this. I feel like you have dealt with this a lot really specifically, but it's like AI—you still have to manage it, even if you still have to tell them what to do then specifically. And that's really hard in a lot of environments.
So Alice, you have, again, you were—and correct me if I'm wrong—but seven years in Double?
Alice:
Six.
Adam:
Okay. Was AI at all a part of the early vision or was it something that you became aware of after launching, and then how did that change in the course of the last couple years?
Alice:
I mean, I think it was always part of the vision, but in a very long-term way. And I think like Samiur was saying, all of a sudden some people just came in and started using tools that weren't available. We were those people. We always said, and kind of when you go raise money and you're pitching like, "Well, right now we're doing a first version, but soon we'll do AI." But it was kind of always a far away dream because we were a small team, super resource constrained, and so we didn't have the resources to train our own model and to build it.
And so for us, I think it was kind of magical in a way because what we had been selling, the super long-term vision that we had been selling to investors, to the team, to our assistants, to our clients forever suddenly became not easy—and like Samiur said, it really well, it's one thing to know what you want to do and then actually do it and actually provide value—but it became achievable and we could actually put our hands into it, just connect to a few APIs and start playing and start pushing stuff to clients.
So for us, it was really an amazing moment to live that. It was also for us, given the business model that we have, it involved a lot of change management, especially not on the client side, because I think on the client side, most clients are excited to use AI. It's something that is great. But we also, our business is that we also have human assistance. They're all based here in the US, all super qualified assistance. And for them, AI is something that they can be afraid of. They're like, "Well, the way people talk about AI, it's going to replace my job."
Especially, everyone talks about AI assistance and so far in their mind, it's a lot about, "I'm done, AI is going to replace." And so we had a lot of pushback on the AI piece of, "I don't want to use AI. I'm a human, I want to." And so I think for us, it's also been super interesting to do that change management with the assistant and say, "We're not building AI to replace you. We're building AI to elevate you for you to be able to do a better job and for you to focus on the things where you have a lot of value. And this is actually an assistant for you." And that has, it's been super fascinating for us to see our assistants get trained on the AI, understand what it can do, and ramp up to using it now on a daily basis and really liking what it's adding to their day-to-day.
Adam:
Yeah, and correct me if I'm wrong, but so Heyday, pure product. Correct product. And then Double mix, right? Augmented. And Taskade back to pure product. Correct. And a more agent focus. Right. And maybe you can explain that, John.
John:
Yeah, sure. I mean, I think going back a little bit on the question and that ChatGPT moment as well, when we thought about that initial launch, the wow moment or the aha moment—I think that that effect it had on people, it really was just communicating to everyone, the power of UI, the power of product for mass adoption to happen. And the innovation I think was around the chat interface and the ability to see the streaming text as if though someone is typing to you.
And this moment was kind of magical in many ways when you thought about how LLMs were actually powerful. And connecting a little bit on productivity, we always thought about Taskade and Task to remember the name for Taskade AI, and trying to help you break things down into smaller pieces by taking a chunk of information, try to organize it. And then this whole concept of building a second brain was around for a few years as well.
But now you can dump all of that information into LLMs and help you organize. And the crazy part is you can actually dump it into a chatting interface. It can do whatever you want it to, and it can output everything from a flow chart, a mind map, a task list agenda. And that was kind of magical. This chatting interface can actually do all of that for you and take all of the information and organize it and present it in different form factors in text.
But yeah, I think from our side, that is kind of like the aha moment of connecting the LLMs into our chatting interface where you can chat with team, but you can chat with AI. And then of course, we also had the editing component of being able to create your task list, your to-dos, your structured notes, docs, and mind maps. So it kind of all worked out, and that was a bit of aha moment for us, but we felt like we really just piggyback off of the innovation that came about.
Adam:
One quick kind of follow up on that side of it before I have a question about what AI is maybe not good at—but before I do that, that came up from what you guys were saying is, from a team organizational perspective, if Double, I would imagine maybe you didn't have an AI team before and now the APIs act as that, but Heyday and Taskade where you were involved beforehand—are there parts where you're just not working as much in a certain area of the core tech and you're on top of it more? What organizational changes within the team have taken place on the back of the innovation is maybe the more concise question?
Samiur:
Mine's probably the easiest because no change. I mean, we were an AI company from the get-go. So basically for the most part it's like, if we can get away with not training our own models, I guess that's less work. But we still have a lot of people thinking about machine learning and AI.
Adam:
On top and been accelerating what's available. So you're already, yeah.
Samiur:
Or even what's the right way to use the available APIs? What's the right data to collect? There's a lot of things that—essentially having machine learning people is still pretty necessary in my head to do it well, and so our team composition hasn't really changed.
Adam:
But I guess have workflows of that team changed?
Samiur:
To a degree. I think we can experiment a lot faster just because you can get a sense of the capabilities of something like LLMs. We primarily do most of our work in text, so we don't do much around the vision stuff, but in text, there's so many either open source LLM providers that we can just open up Grok or whatever to test out—how would Mythril do on this? How would Llama do on this? How does GPT do on this? How does Claude do on this? And then we can figure out, okay, this approach to solving this problem can work. We're getting decent responses, and so then we can double down on optimizing as opposed to these things being so available. It would be a lot of work for us to do that first pass MVP test.
Adam:
Yeah, very cool. It's just less ability to move faster off of the—I feel like the story of software more generally also, it's just a step up now from where we were.
Samiur:
You can kind of think of it as like before AWS or things like that.
Adam:
Exactly. Right.
Samiur:
You had to get your own servers and to put up a basic website. So those kinds of, they're kind of step function changes along the way.
Adam:
Yeah. Double I would imagine very, very different because did you have internal—you didn't have any maybe before. And then,
Alice:
So we've always had an engineering and product team, but mostly working on building productivity tools. And we kept that and basically they were working on just pure productivity tools like task management and stuff like that. And they all moved to AI. Right now, I think probably 80 to 90% of our resources are spent on AI features and the rest just maintenance.
The thing that I found interesting, I'm curious if it happened at Taskade or Heyday, is in the role of engineers because it's so accessible now to use. I think that was fine. The transition went away well. We noticed a real change in the workload between PMs and engineers.
And for us actually, because a lot of what we're doing in prompt engineering, the role of PMs actually has evolved quite a bit for us in the last year where they're not just about scoping out the feature and then doing QA and figuring stuff out. They're actually building the feature because they're the ones who are owning the prompt piece of the feature. And so our engineers are basically just giving them a place to do that and iterate really quickly.
And so the dynamic has changed quite a lot, where PMs are taking a lot more than what they used to or taking different things. And for us, that's been super interesting as well, especially with constrained engineering resources to be able to offload a lot of that stuff as well to product managers.
Adam:
Yeah, interesting. Similar experience. John, Samiur, similar shift on the PM-engineering role?
John:
Yeah, I mean, I think of myself as a glorified PM in some senses, but the crazy thing is—you mentioned about that—the ability to start building prototypes faster and even sending PRs to the team myself and getting rejected by the CTOs, the co-founders, I was able to actually do a lot more than I was able to in the past.
But also at the same time, the code quality might not be there, but you can really get prototypes out much, much faster with assistance. And that's going to get crazier and crazier over time for sure.
But for our team day one, it was mostly very technical on the product side. And even when we pitched to Paul and Jared at YC Summer 19, we pitched real time collaboration and organization because our backend and product was always around a structured document that was collaborative.
So we had our own algorithm for OT, not CRDT. We did a lot of backend indexing in real time. So when LLMs came about, I think it was kind of lucky because we just about wrapped up a lot of the pieces for the foundation and for the platform for Taskade. So we were able to really just transition and go all in and get everyone on board to dive deep into it, AI and LLMs and RAG and so on and so forth. But it's also super exciting. Again, there's something new coming out every single day and it keeps just building momentum for the team without you even having to do much because it's just about trying to get the team to focus on the right stuff.
Adam:
A question that I've been excited to ask, and I think you all have a really great insight on it, is—what has AI—what have you seen AI been really good at and what have you seen it not really be good at? Right? There's lots of promises. It's really fun to think about the future where it does everything really well or whatever. It's not where we are today. What have been some surprising moments of like, "Wow, AI does this better than maybe we even expected!" And then, "This just isn't useful for this."
Samiur, I think you've probably been living that reality for a long time.
Samiur:
My answer's probably not going to be as interesting. I'm more curious to hear from John and Alice. Just sort of like, when you see your kid growing by an inch every day, you don't notice them growing up. It's like the grandparents are like, "Oh wow, you've grown a foot last year!" This has been my world, so I'm sort of incrementally along the way.
Adam:
Really, it's been a long time since you were surprised?
Samiur:
Yeah, sort of like this is the thing that I'm used to. Even when ChatGPT came out, I was like, "Oh, this is an awesome interface for GPT-3, which we've been playing around with, but was too expensive for us to use consistently." And so I'm curious to hear from Alice and John actually.
Adam:
Alice, what was your experience?
Alice:
So we use AI in our tools that are user-facing both on the client side, so to help with delegations, refine a task. And then on the assistance side, obviously for task execution. And I definitely think that we had super high hopes on the assistant side of like, "Oh, AI is going to be able to execute on a lot of things," which it's not.
And there's actually a lot of what our assistants do on a daily basis that AI can't help with right now, probably won't be able to help short term and maybe very long term. It's going to be able to help—obviously all the calling people or reaching out to multiple people at once. All the more complex tasks.
The tasks that it's been absolutely amazing for and that our assistants love using it for are everything that's related to writing. Obviously those are language models, so drafting content, reviewing, producing content, writing emails—really great at classification. Our data became so much more accurate because now whenever a client puts in a task, we're able to—we automatically tag it based on AI and we can do way better reporting than what we used to do.
Really not great for us at everything that's numerical values. We realized that it was really, really bad at estimating how long a task is going to take. So if a client is like, "Oh, can you book me a flight to so-and-so?" it will give very different numbers multiple times.
And for us, the key learning here is—it's actually at the end of the day, at least for us and from our experience, the output of the AI is only going to be as good as what you input into it. And right now, both our clients and our assistants are not great at those inputs. And really, even if you are familiar with AI, even if you've used it before, knowing what to share and what not to share and what's going to be relevant is still super hard.
So obviously there's a lot that we can do on our side with automatically giving it content, but I think that's the thing for us that is the biggest thing to focus on right now is how do we make sure that that input is facilitated in the best possible way?
Adam:
And that input being the prompt being used or the data it's pulling on, or...?
Alice:
Both, it's the prompt, it's the personal context of the person so that the response can be linked to them. It's kind of the expectations. I think people usually have super high expectations of the AI, but if you give it a really bad input, then it's not going to figure it out for you.
And so I think that kind of expectation setting as well, what are you actually going to look for? I think for us is the things that we're working on right now the most.
Samiur:
Yeah. Just to inject for a second, one of the things that I think is to be figured out is for all the folks who are new and using APIs—sorry Alice, not to be, but more just like, you're getting a lot of value from the initial use case, but then there's the classic things that people probably associate with AI or machine learning even subconsciously—is if I give it feedback, it will get better.
The problem is, if you're using public APIs, you really probably aren't actually making anything better, which was a default thing. If you're building your own models, you're training your own models—by default, that just becomes data to make for the new retrain of the model, it will get better.
And so one of those, it's like this kind of figuring out what the inputs are, and then it's like, well, having an approach to, "Well, how do we make this better? When do we make this better? Can we have user interaction? How do we incorporate user interaction into making it better?" Not quite—the APIs are not there to make that quite easily usable yet.
Adam:
John, how about you? I guess you mentioned the ChatGPT team being a big kind of step up and the team kind of rallying around it as well, but have there been—I don't know, parts that have been less than—what have been a down moment of it? Or is it all just all great? Is it just all the new—have you been disappointed I guess by any of the releases? Have there been exuberance and then like, "Ah, it's good, but it's not that good." What's been that?
John:
Yeah, I think it is funny. Every single time there's a new model coming out, people have different kind of opinions about it. You try to benchmark it, but ultimately it's very hard to say exactly what is a step up when you're interfacing with a lot of these from a human angle.
But I guess starting on a positive note, obviously LLMs, AI has been great for everything from research to creative writing to all the way down around productivity and turning a lot of context data and organizing it for you and turning it into things that's more actionable.
But on a more human level, in terms of AI, I think it's kind of surprisingly good at knowing about yourself. So if you curate the right amount of context knowledge, you pass it to LLMs, you ask questions—and even not just from a productivity standpoint, but from a mental health therapy, trying to understand yourself better, it actually gives you very good answers in terms of understanding who you are and the problems you're going through and suggesting the next steps.
So there's kind of scary moments where you're just talking to the AI and you have a really good threat going on and you pass it the right context. You're like, "Wait, this thing knows who I am." It's scary. But of course, and this goes back to the point, I think it's only going to get better, but of course the limitation with the context window, of course the memories, the long-term buildup - there's still a lot of limitations there today, but that's only going to get better.
There's definitely a lot of letdowns when you think about agents and tool calling and the ability to do task completion, right? Because there's so many different tools and if you want to limit it to just a set of really strong use cases, there's not that many today, but I do believe that's going to change. And today, not there yet for sure when it comes to completing actual tasks for you outside of just text-to-text.
Adam:
So within that, you've mentioned the slope of—let's assume that this momentum will continue, the LLMs continue to get better a variety of ways. Maybe they get better at the task completion, maybe they get even better at the things they're already doing. How do you all think about value capture on top of that?
You all sit in these kind of—and we'll talk about pricing and how people pay you and how people use you—but I feel like the common thing there has been proprietary data or context. How do you think about—let's say that the LLMs keep getting better and better and are more generalized. When do they eat into the value that you're providing? Do they not? Are you always on top of them in a way that you think allows you to capture the value, whatever defensively?
Maybe this is kind of an investor question, but if AGI gets here, why do people still use Heyday or Double or Taskade? And maybe that's a hard question or maybe that's a dumb question.
Samiur:
I think I have really strong opinions about this.
Adam:
Yeah, I would assume anyone who's built—I feel like if you're building in this, you have to—you can't say, "Oh, I don't know, we're gone."
Samiur:
Yeah. I mean, I am continuously getting questions around like, "Oh wait, is ChatGPT just going to do this or whatever." It's very possible there's going to be features built in, but that's more like taking the route of a super app that does everything. I just don't think that—I mean, they could try to do it. I think it's going to be really, really, really, really hard.
So as in you probably need to get close to an AGI to actually—and I don't think we're actually anywhere near AGI, by the way, people talk about that. We're just nowhere near it. We've built phenomenal memory pattern matching engines that can do a lot of things because we fed it a lot of information and it can see multiple levels of patterns. But once you get into zones where you're trying to get it to do something that it's never seen a pattern of, it's just not going to do well.
And that is, I don't know how human brains do it, but we're pretty good at it. And so that's probably the big thing. So one of the things that I think when you're trying to build an AI—if you're trying to build both the super app and the API that allows people to build meaningful, specialized things, you can't really be both at the same time just as a comparatively small company like OpenAI.
And secondly, I think that that's where antitrust will—if they actually pull it off. I don't know how that goes, but Microsoft's saying "Internet Explorer is the only browser on Windows" kind of level, or Apple getting sued now for—I don't know, if you're trying to be both the platform and the only app on top of it, you'll probably get in trouble. I don't know if you'll pull it off.
And just the type of specialized use cases that most people actually have to deal with—it's similar to saying you have the smartest 12-year-old on the planet, IQ 300, whatever, and then you say, "Do my VC job for me." It doesn't matter how smart that kid is, they're not going to just immediately jump in and be able to do your job. So you have to train it to do that. Is OpenAI going to figure out how to train every single job on the planet? I don't think so.
So it being a platform just makes so much sense. It extracts a tax on the ability or whatever, all these providers—similar to would you have said AWS can build every app on the planet because they have the ability to scale all the servers on the planet, whatever.
Yeah, I think it's probably on us to figure out how to actually solve people's problems and how to use AI—and it's different problems for different things. So yeah, anyway.
Adam:
No, that makes a ton of sense to me. I hadn't heard the monopoly take before, but I think that's an interesting one, even if they could—that's so far away though. Again, I just don't...
Alice, I feel like similar in terms of how you think about it in terms of the LLMs continuing to get better, it just allows your assistants and ultimately your clients to be served better within your platform.
Alice:
Yeah, exactly. And for us, the idea is too—there probably, well, I mean "always" is a really intense term when you're talking about startups, but at least the short to medium term we'll have humans in the loop. That's the big value prop. And we really don't think right now that just AI can do the type of work that our assistants are doing.
Because again, there's a big element of reviewing stuff, of taking the phone from time to time, of coordinating a lot of different things, actually executing on tasks that ChatGPT right now can't execute on. It can give you recommendations, it can share next steps, but it can't actually log into your bank account and make payments, which an assistant can do with the right accesses.
So I think for us, that's what we're thinking about right now is just—how do we leverage AI to complement our assistant and make sure that the quality is more consistent, that they have all the superpowers of AI that know everything at all times, never be super consistent on the quality, but not really about how do we just make ChatGPT be the ultimate assistant for our clients, but more like how do we find the right balance between the two?
Adam:
John, similar?
John:
Yeah, I think the scary part, again, touching on the LLMs too—a lot of...
Adam:
Scary stuff from John.
John:
I know, when you take out your laptop too, when you start thinking about MacBook Pros and then even pulling up your MacBook Air, I think a lot of people don't realize that you can run Mistral AB on your machine locally and you can actually interact with AI and LLMs, and these are all open source and available today, and that's only going to get better.
And the closed source ones like GPT-4, moving to GPT-5—I mean the capabilities are only going to improve. So the way I think we see it is to really position Taskade in a way that whatever we're setting up today, whether it's the multi-agent, letting the customers actually personalize, customize the workspace or setting up the automation and orchestration layer to connect with our platform, all of those are only going to improve when the next leap happens with the LLM.
So in a sense, we want to really partner with them the long term, but of course, in terms of training your own LLMs or fine tuning, a lot of those things are possibilities. And it's not necessarily something you have to utilize third party as well, especially if you have a lot of customers already today, a large user base, and you can actually just observe the actions people are taking and try to make that into even your own LLMs. I'm sure you've seen the Rabbit AI one's large action model, but if you actually have a customer base, user base, a lot of these things will become more and more possible.
Samiur:
Yeah, I mean, our product Heyday basically does this. We integrate with everything from your browsing history, your emails, your conversations, everything and help you achieve certain tasks. The thing is, I still don't think if you just take that information and then put in anything more complicated—if you just want to chat with our system, it can do basic tasks very well.
And then for our workflows that are very optimized for the use cases that we're working on, we've done a lot of work to train based on what we know are the actual things that people in our persona are actually looking for. And it takes a lot of work to get that to the point where people just trust the AI, because again, it turns out it's cool a lot of times, but it's not the type of thing that you just let run by itself. It's not like the same thing as me having a chief of staff or an executive assistant, and I can just say, "Oh, cool, I know you can take care of this. I don't even have to think about that."
Adam:
Yeah. I think a good follow up to this—the value capture and the owning ultimately the customer relationship. And I personally agree with that—the platform and then building on top of it. You use example of the 12-year-old, even if the 12-year-old becomes a 15-year-old or an 18-year-old or 22-year-old, they're all still general. It's like the liberal arts education—and can you do this really specific thing though still? And that takes training even in the human context. You're an awesome history major, clearly smart, whatever—you still have to show up to your job and get trained to do your job, just figure it out on the fly or whatever.
Samiur:
And one of the issues with LLMs, and I'll keep harping on this—the way that these work right now, they're basically either they can find multiple patterns and just squish them together to do something that looks really cool, but innovative new things like new music, new literature, new styles—rap isn't going to be written in the 80s by an AI. That stuff just won't happen from an AI. So if you're working on even at the 20th percentile of novel stuff for your industry, like an AI by itself is not going to be able to replace you.
Adam:
But yeah, back to the value capture aspect of it—so I think that pricing has been, and we've talked about it before a little bit, and it was in the kind of pre-show notes, big kind of hot button thing recently. I feel like it was happening before AI, there was definitely this per seat versus usage conversation happening before the ChatGPT moment. And then it's been accelerated in I think a lot of ways because of—I don't know, maybe it's just because software is more accelerated, more generally.
How are you all thinking about pricing? And I know that you probably have some questions for each other as well, and obviously different human versus pure software or whatever. But in terms of the product—has this shifted? Is it an ongoing thought that everyone's having? Samiur, I'll keep the loop going.
Samiur:
So we're probably earlier in our lifecycle as a business than Taskade and Double. So I'm very curious to hear about it from y'all. And we've been—the Heyday product up until recently has been an individual AI assistant for knowledge workers focused on executive coaches and consultants.
But we started working with consulting firms and once you start think going from a, "Hey, I'm powering the productivity of an individual and they're paying me for it" too, "We're trying to help an entire firm be more productive and efficient"—the per seat license becomes weird in my mind, in that we may actually be helping the consulting firm do more with fewer people. And so therefore are we like...
Adam:
Yeah, there's a weird incentive there.
Samiur:
Exactly.
Adam:
You need more seats, but it's a productivity app, so you should need less seats and then...
Samiur:
Yeah, right. Exactly. And so that's one of those tensions where we're still figuring out, and we're not sure exactly how that should be or if there are any ideas that we should be considering.
Adam:
Alice and John, any ideas for Samiur?
Alice:
I mean, we're in a similar situation of notpricing model is broken.
And so what we're having incentives that align. And I think that's a lot of companies are going to be in this situation. For us specifically actually building AI is shooting ourselves in the foot because right now our pricing is not even perceived—it's already per usage, but it's like the hours of our assistants. So they pay packages of hours.
And so actually the more AI our assistant use, the less hours they work. And so the less our clients pay and our assistants are not incentivized to use AI because then they're paid less. So the entire model, our working on right now is to figure out pricing based on the output. So based on—you're paying actually for the result of the task. Where it gets complicated is again on those expectation setting of what is exactly success for a specific task and maybe what success is at the end of the task is not what it was at the beginning because a lot has happened.
So that's where we're spending most of our time. We don't have the solution yet, but we are—what we're trying to do is be as descriptive as possible about what does the client want. So really, really working on those expectations and then giving a price for that—would you be willing to pay 30 or 40 or $50 for this task to be done? And then the client can say yes or no, and then we pay on the same side Double a fixed price to the assistant for the task. And so they're incentivized to use AI because the faster—the price doesn't change if they work 10 minutes or 30 minutes on it. So they're incentivized to use AI.
So our goal right now is to realign everyone's incentives. But it is, it's tricky, right? Because it is not something that we're used to and it adds a lot more work upfront. And so adding a lot more friction upfront.
Adam:
To defining the task correctly, defining the...
Alice:
The task, asking the client, are they ready to pay without even seeing the quality of the work. And I think it opens a can of worms, but it is necessary for us to figure it out.
Adam:
Interesting. So it's less—it's like seat, usage, outcome is the full lifecycle, and we're almost accelerating through usage to outcome because in the world you don't want actually lots of usage and that applies. Productivity is a very clear example. You don't want—whatever, Twitter, LinkedIn tracking, how much time are people? I just saw some notification about LinkedIn's launching games to get their usage up on the app more. And it's like the opposite spectrum of productivity type. But yeah, so usage—so almost accelerating through that to what do you want the outcomes to be.
But in any hiring, and I've worked with contractors and I've worked with assistants and stuff, defining the thing that's happening ends up being such a huge task if you do it really well and then it can change and all that stuff. But...
Samiur:
Side note related story from a long time ago, which might be—it's still important to us, is when Google was first starting out, Larry and Sergey didn't really want to build a company, and so they were looking to sell the Google technology to a company. And at the time, one of the biggest search engines was Excite and the Excite team had the Google team on, and there was some CPO type person who looked at the Google data and they were like, "People spend so little time on the Google search pages, we're not going to be able to..." They were focusing in on the fact that people would come to Google and then leave right away.
Adam:
You type it in, you press the link, you're gone, you're off the website. How are we making money here?
Samiur:
Yeah. And compared to Excite, people would be going through multiple pages of Excite results and not understanding that engagement there was actually a very bad thing.
Adam:
Yeah. Engagement's not always—especially in productivity feels like the extreme example of this, right? Where John, yeah, how are you all thinking about it?
John:
Yeah, and I think we've just covered on some good points around reducing friction because that was always the hardest thing to think about. We've experimented with three different plans, four different plans, even five, and then you cut it down to two, and we noticed the less friction, it was always the better kind of choice, especially when Taskade is a freemium product and you go after many SMBs and business owners.
And of course going back to productivity, the best way probably you can charge folks is by saying how many hours you saved them or usage based around task completion. But when you ask the customers, they say that's what they want, but then at the same time, they don't want that because you're making them do math. You're making them think how much you're going to pay. It's like they want that, but they don't want that. So it's very tricky.
Adam:
Building pricing page with a calculator in it.
John:
Exactly, the slider—we tried that slide things up down, but the way we ended up doing that is kind of simpler where your usage goes up as you add more seats. So it's a shared pool of a lot of the resources, and as the shared pool increases, the more seats you buy. So it scales nicely with your team within your workspaces together in unified kind of subscription.
But to touch on the usage of knowledge as a piece for agents, and tying back to the fact that I do think AI assistants, copilots are not that great today, but the point being LLMs are always going to be very generalists. They know everything, but they don't know anything super well.
And the way that we've spoken to our customers is that in order to build the best expert for a specific area, they are the ones that know it best because they already know what knowledge to feed it and to curate it and then actually come back and say, "This is crazy. This is even better than myself."
But you look at the stuff they uploaded into the knowledge or into the agents that they created, it's insane. They know exactly what to put in, whether it's the docs, the stuff they've written, their project contacts within Taskade.
So in a way, even if LLMs do get better, it's still up to the human to train it and to curate the knowledge. And then you can create a set of experts to work with you. And then how do you monetize on that? Maybe the usage should be around knowledge, not about the output or not about the task completion, but how much knowledge you can store. And you see that a lot with company specifically targeting building out the rack systems and so on and so forth today. But anyways, just kind of jumping around a bit.
Adam:
I think, John, you mentioned maybe a Pokemon path of building some Pokemon—whenever someone brings up, the word "training" gets thrown around a lot, and as I grew up with Pokemon, I just go back to walking around my Game Boy or whatever.
And I've always—because you don't know what it doesn't know, there's this aspect of building on the generalist and training, and I want to be able to feed something to my LLM, right? "Hey, you know this now." It's like The Matrix is maybe the more grown-up visualization of it where they download kung fu and stuff like that because an aspect of knowing what your LLM does know and doesn't know, and all of the demos and stuff make it feel like it knows everything. You watch a demo of a ChatGPT app, it's like, "Oh, it knows everything. It's perfect." And the reality that we're all kind of dealing with is that it doesn't really—it knows a little bit about everything, but then if you're going to really get deeper, it...
Samiur:
Actually knows nothing. All it knows is...
Adam:
Samiur—it's always the guy who's been deep in it for the longest, who's like, "They don't know shit. I don't know what everyone's about."
Samiur:
It's repeating patterns in a way that makes sense.
Adam:
Yes. To move on from my Pokemon reference, I think a cool question for all of you who have both been in the AI space for a long time and also have been building companies for a long time—there's been a surge in and there's so much of it that's hype, and it's one of those classic moments where as an investor or as a founder, and I have friends on both sides of the table, thinking about the space—what's the right thing to do? What's the thing that you can raise money for? What's the thing that is actually the longstanding or something that actually has real future potential?
Is the ground shifting so quickly? How do you decide you don't want to miss it? When you all look out from your—you have established things that you're doing and you were doing it before the ChatGPT, you made those decisions before, and this is happening outside.
When you look into the AI hype bubble or whatever it might be, and you think about maybe building something else or someone asking you, what do you think is really interesting to—if you weren't working on this, what would you be working on? Are there things that come to mind for you all there? Are there things that you see? And again, putting aside the fact that you're all working on what you're most excited about by virtue of how you spend your time on a day-to-day basis, but maybe in second place, is there anything that is interesting to you all or that you see other people doing that you're like, "That maybe wasn't possible when you first started your company as well?"
Samiur:
That's a great question.
Adam:
Give me your startup idea. Give everyone your best startup idea.
John:
I mean, the point of being just text-to-text and then of course the evolution of text-to-voice. Now, text-to-music, text-to-video, that's only going to get better. But the ability to go from audio to video or going from different mediums, compat, that is going to become real as well. But we're not quite there yet. But if you were to build today, you want to build for the future and you would want to build knowing that that's going to get better. The video is very exciting in some senses. And of course with the recent kind of wave with the audio and the music startups, imagine having that as a kid growing up, that would've been just insane.
Adam:
Yeah, that's cool. So moving away from text-to-text, and I think there's a lot of actual product experience, there's a lot of innovation that'll have to happen in terms of how you interact with software more generally. I feel like I make fun of it on Twitter sometimes, but the Vial's new thing where it's a social media, it's kind of like Clubhouse seed, but it's pure dictation. It's meant to be—I feel like there's lots of experiments happening in that space of how we interact with software or social networks, whatever. Samiur and Alice, any thoughts come to mind?
Samiur:
Yeah, I think for me, it's all in this area of how do we free up time for humans to spend on, just can't...
Adam:
Get off of the productivity.
Samiur:
But it's not about helping humans be more productive all the time. It's about, so how can we help humans spend more time on literature, music, fun, sports, whatever. And so what are high value things that we do as humans that can be automated or what will significantly improve quality of life?
So an area where I think is very underserved, but it's super valuable from an AI perspective—it's only recently people are thinking about it—is drug development therapeutics. What can we do to have AI accelerate research into treatments of any kind, longevity, all these kinds of things. That'd be super interesting to me. Agricultural development. So how can we make good food that's environmentally sustainable? So I want my beef, but I also don't want to destroy the planet while everyone gets beef. So there's got to be a way we can figure this out. How can we leverage AI to accelerate those kinds of things. Those are the things that I would probably spend more time on, but this is more fun.
Alice:
I'm the type of person that can only think about one thing with that intensity. But I think one thing I do hear sometimes, and I sometimes feel from the VC world, is this thing of like, "Oh, now that we have AI, we have to think about all the usage of AI." And to me, at the end of the day, AI isn't an endpoint. It's like a tool. And maybe Samiur's going to be like, "No."
But for me it's like it's a good tool. It's a hammer, right? And it's like when we're thinking about what are the startups we can do with AI, it's like you have a hammer and you're going around your house trying to find if you have a nail or not. And maybe you don't, you don't know.
I think for me, at the end of the day, it's more about finding the problems that interest you and then what's going to sell to the clients. But clients don't really care about AI. They don't use something because it has AI or it doesn't have AI—they use something or an app or a product or a service because it fits a need that they have. And if AI makes it better, then great. But if it doesn't need AI, then maybe it's fine to use apps and services and tools that don't have AI if it still fits your need.
And so I think it's—for me, it's obviously AI opens so many new possibilities, but I think launching, as I'm thinking about or anyone thinking about launching a new company, it's always about what's the problem? What's the need that you're trying to solve? And then again, if AI can be a tool for you to do it faster, better, more efficiently, let's go. But it's not an end in itself.
Samiur:
Although I will now defend the VC for a second—it's probably less like a hammer and more like the invention of fire. It's like the equivalent to the internet. People are just more aware of this monumental change that is now available for us technologically, but it's still more like a hammer than it is the all-encompassing solution to everything. And so I think it's like, "Oh cool, what are new things we can do with fire?" We can cook food. That's great, that's awesome. But not everything has to be about fire. There are other things you need to do that didn't involve fire.
Adam:
And I think that it's not that the world's problem set has changed. You mentioned some of the big ones of disease and food and those things are still the same thing. Now we have new tools that make it—or productivity, I'll put that in there as well. Well, from what you get done at work and how that frees you up to enjoy your life more—haven't changed. And yeah, it's interesting, but the "why now" is super compelling. Like, "Oh, we're now in this new paradigm" and it almost overshadows other slides. The problem-solution slides and then the "why now—AI" slide's like double bold face, whatever.
John:
But I think even the reference to prototypes before—for PMs to be able to use AI to build that, that's very similar to creating a sound clip or creating a song today, or even video generation. It's very good to get to that prototype level, but not so much to the end product. However, if you are building a startup around building a community of folks to actually come together and learn and create, it could spark a ton of new ideas and creativity from the product type angle. But applying to multiple categories everywhere—and there's so much you can do and just follow your passion and the areas that interest you, whether it's Pokemon or gaming or music, entertainment. I think it's way beyond just text-to-text. I think that's the point I was trying to make, but yes, still quite exciting.
Adam:
Yeah. Well, we're at the hour here. I know that you all have startups to run, so I won't push you too far. Or maybe you have AI do it. No, I think we've determined you can't. Actually.
Samiur:
I can. It'd be great.
Adam:
Yeah. Thank you so much. I really enjoyed it. I definitely—I know that the audience, I'm sure the audience did—we'll be cutting up the recording and getting clips out and all that stuff. So thank you again. And I'll put up this slide to thank Sacra and Walter, our friend Walter, and I hope to see you all soon.
Samiur:
Looks like there was one big question that came in. I don't know if...
Adam:
Oh, yeah, I can read it off. I think we covered it mostly, if not all the—I'll just read it out maybe. How about this, the difference between the adoption of—I scanned it for bad words already. I appreciate that, Samiur. Usually we don't do it, but it's helpful.
Samiur:
No, no, I was just pointing.
Adam:
Yeah. The difference—can you guys see it? Or maybe—and I can put it up on the screen. It's too long really to be helpful, you guys—anyone who's reading this, you can read it on the side and if you have an answer to it, go for it. I'm just going to start asking...
John:
"AI, summarize TLDR." Let's go. What is it? Restream needs that feature.
Adam:
Yeah. The general question is can it do everything? What happens if it—I'm trying to condense it. Bigger or smaller—launching generators, innovators, quickly create enough jobs—a human labor question. I personally—I think that, and maybe I'll channel some Samiur here—I don't see that happening really anytime soon at all. And I feel like that's been—we had this ChatGPT moment, and then everyone went like, "Well, what happens when we don't have anything to do?" And it's like, I don't know when that happens. Really, Samiur. And I don't think...
Samiur:
There's a lot of industries that are at risk at being automated away, and it's going to be great—but great for the world, but not great for the people, unfortunately because there's no retraining or how do we affect—let's say the trucking industry is going to be essentially...
Adam:
Bringing stuff from point—if you're a truck driver and now we have...
Samiur:
That's going to get automated in the next 10 years, 100%. So that's 5% of the US job market.
Adam:
Is it really?
Samiur:
Yeah, it's a huge—logistics and transportation is a huge market. And so I don't know, no one's thinking about—I get really annoyed with the fact that people think about existential AI risk before the things staring us in the face where there's going to be disruption, not the kind where it's just replacing high knowledge workers and things like that. But it's replacing a lot of things that humans needed to do. But maybe we don't anymore. It's actually good for us because those people could probably work on things that are more interesting than driving a truck or going into the mines. Those are jobs that—why should we have humans do them? But it's also not something that our government is really doing a great job supporting the transition from.
John:
But I mean, thinking about just autonomous driving, when you think about Tesla, FSD—it's gotten so good, but obviously still problems. If you're in SF, you see Waymo going around, you ride it a few times. The first time you're really scared, then by the third time you're like, "Wait, this is actually pretty good." And it's a lot faster than even waiting for Uber to pick up sometimes. So it is kind of scary in that sense. When you think about knowledge workers, task completion, autonomous agents—the crazy part is it will inevitably replace jobs, like you said, but maybe not so much like the expert level, more like the internship level, not some full-time employee that you're replacing. But when you're talking to customers that are running businesses or small business owners, if we do help 'em save time or save resources because they don't have the budget to hire 10 people, they can hire three and then the rest can delegate to AI. That's a win, I think, for our side. But the trucking point is also valid. That is kind of crazy. And it will free them up to do other things, but some people will be affected inevitably.
Adam:
Alice, I feel like you kind of touched on this really specifically. I feel like the AI assistant—and I think that what you said is where I end up thinking about it, and I think it's kind of what Samiur touched on as well—is augmented, right? There's still—you do different tasks and I think that the general—truckers now, yes, new jobs, but the whole technology stack has shifted. So now there's new things available. There's also new industries and new things to do. And I feel like Alice, I would assume that you feel like that more broadly as well.
Alice:
For me, it's not really about jobs, and I think we've heard all this everywhere, but it's not about jobs being—at least in our industry, obviously truck drivers definitely. But I think in our industry, it's not about assistants not existing anymore, it's just assistants who don't use AI being replaced by assistants who do use AI.
And I think it's—for me, when I talk about assistants, usually people have this image of the secretaries in Mad Men typing away, and I'm like, well, they were on typewriters and the day where we invented computers and they could type faster. And they didn't have to post things because emails was there. There wasn't no more secretaries. It's just that their job evolved quite drastically and they started doing a lot more things because there's always things to do.
And I think for us, that's the way we see it, of the role of the assistants still being needed, but the tasks and the scope that they do being radically different because they're focusing on things that have that human value and the low level tasks being automated, which at the end is good for everyone, right? It's good for a client who's paying less. It's good for the assistant who's doing more high value tasks. It's a win-win, but it has to be managed well, for sure. It's a fine line.
Samiur:
Or more people can afford assistants.
Alice:
And then as John said, then you're powering more businesses to run and not completely die. And it's just a very short—it's also, yeah, but it's not the case for all industries, obviously. I think there are industries where jobs are just going to be automated away.
Adam:
Well, I think that's what we're ending—I think that's what we're ending on. Hey, well truck drivers that use AI though—secretaries that use AI—get replaced. Maybe that's what needs to be happening. Anyways, thank you for staying over. Thank you for everyone for asking the questions and for engaging—for the over a hundred people that got here. So that's fun. And we will talk to you all soon. Thank you so much.