George Xing, co-founder and CEO of Supergrain, on the future of business intelligence
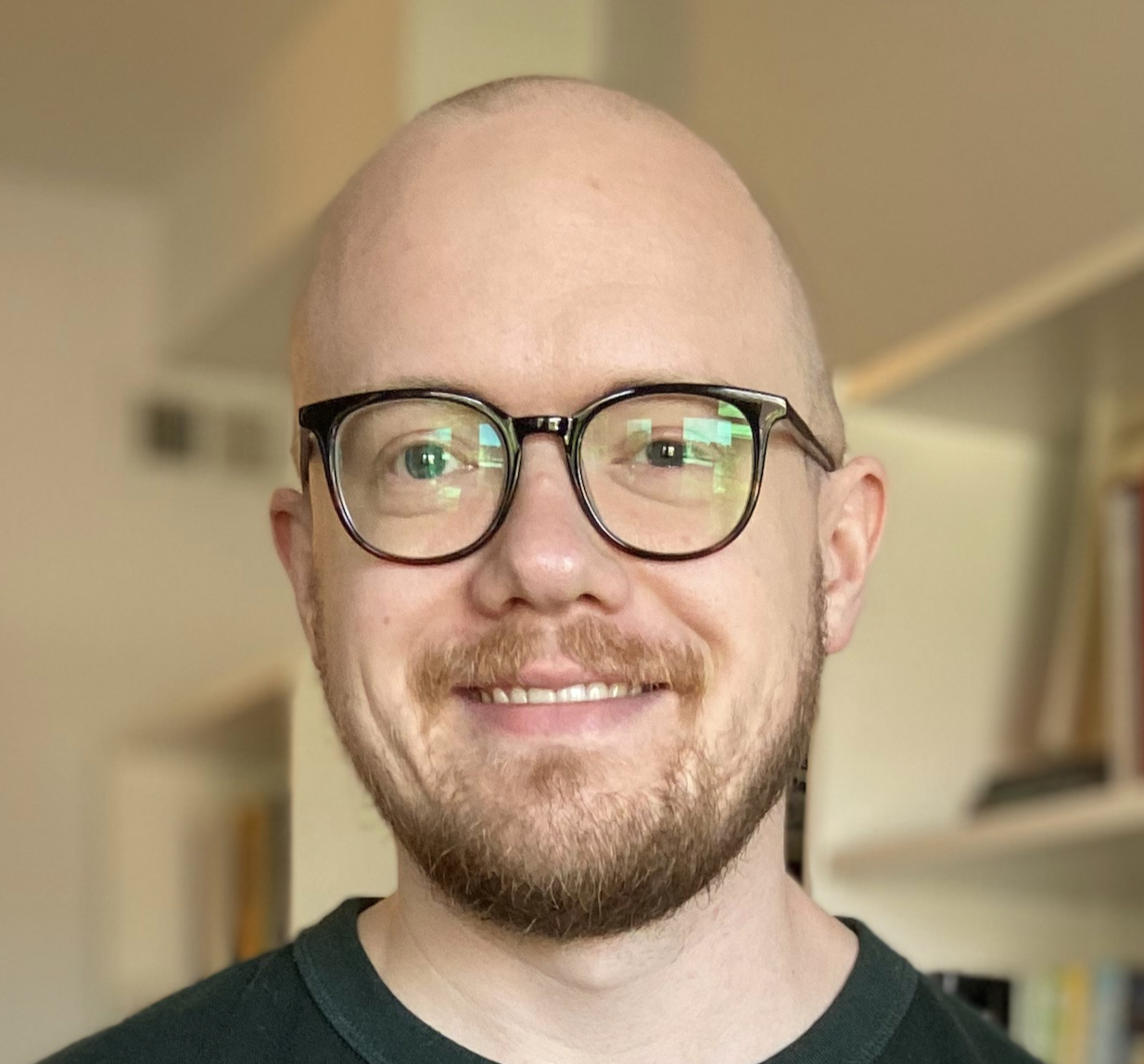
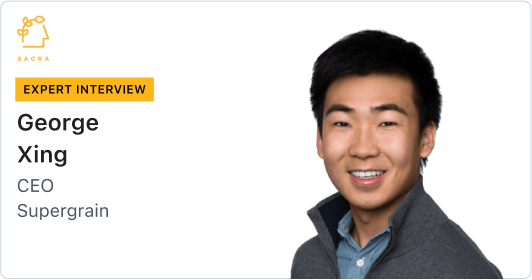
Background
George Xing is the co-founder and CEO of Supergrain.
Questions
- Could you talk a little bit about the evolution of the BI (business intelligence) market? How have teams defined and used their metrics, and what issues have they had in doing so?
- What exactly happens that leads to these multiple different definitions of metrics being created? Why can’t this problem be solved in the data warehouse?
- One thing we’ve heard in talking to folks is that the two valuable places in the data warehouse value chain are the data warehouse where the data is stored and then the place where definitions are maintained. What’s your take on this view?
- How do you think about where interoperability and integration in BI are going into the future?
- Do you see a compelling reason for Snowflake or another data warehouse to build a product like this, or are there structural reasons that that wouldn’t make sense? Maybe they’re making so much money with the data warehouse that they don’t care.
- Assuming we're moving towards a world where the data warehouse is the center of gravity, do you have thoughts on whether that’s incompatible with the CDP model? Or is it more that everyone will have a data warehouse, but some teams will still use tools like Segment, Zapier, Workato, or Tray to move information around?
- What’s your take on the bigger BI tools and how they might be moving towards more interoperability or unbundling? For example, Looker’s partnership with Tableau.
Interview
Could you talk a little bit about the evolution of the BI (business intelligence) market? How have teams defined and used their metrics, and what issues have they had in doing so?
Business intelligence (BI) has been around for a long time. There were products like IBM Cognos and SAP Business Objects, which were this big, monolithic platforms that did everything from storing the data to transformations to metric calculations to visualization.
Over the years, what we’ve seen is that these monolithic stacks have become unbundled and nowadays, modern BI tools like Looker and Tableau are built on top of cloud data warehouses like Snowflake and BigQuery.
What we’re seeing now is that people want to consume data in the interfaces of their choice. However, metric definitions for many businesses are still defined within a single BI tool. The challenge for these companies is creating a source of truth for business definitions across the company for metrics like revenue or conversion.
I saw this problem firsthand while running the analytics team at Lyft. We didn't have the right semantics on top of the data, and different people on different teams were running different SQL queries that they thought were the right thing, but were actually wrong. The finance team was using spreadsheets, product teams were using BI tools, and there were a number of other surfaces in which people were consuming data.
What exactly happens that leads to these multiple different definitions of metrics being created? Why can’t this problem be solved in the data warehouse?
The way that BI tools traditionally talk to data and get data is they run SQL queries against your data warehouse, something like Redshift or Snowflake. There may be a code-based SQL editor, or it may be a drag-and-drop tool that generates a SQL query that they execute against the warehouse. Either way, you have a lot of flexibility there. All the aggregation logic for what “revenue” means and how revenue is defined is done in the BI tool itself. The challenge of that is you might define that SQL query differently in two different tools. You might even define it differently in two different dashboards in the same tool. We've seen that happen a lot at Lyft.
Why isn’t that just done in the warehouse? Why not create a definition of revenue, put it into some table that lives inside your data warehouse, and query that table directly? Well, the challenge with that is flexibility. Any time you materialize a metric into a table in a database, you are defining the ways you can slice that data. You are defining the grain of that table.
Maybe you have that metric cut by day or by week, and you want that metric by month, or you want it by product line, or you want it by city. Then you need to create a new table, or you need to run a separate SQL query that queries another raw data set that gives you the flexibility that you need. You run into the same problem over and over again.
One thing we’ve heard in talking to folks is that the two valuable places in the data warehouse value chain are the data warehouse where the data is stored and then the place where definitions are maintained. What’s your take on this view?
I don't think anyone would disagree that the data warehouse is valuable, so no argument there.
Metrics are the atomic unit for pretty much any type of analysis or data-driven process in a company. Whether you're doing reporting and trying to visualize a chart on a dashboard, whether you're trying to do anomaly detection on why a metric moved a certain way, whether you're trying to experiment on a new product feature, or whether you're doing financial planning and you're trying to figure out where your business should be in a year. All those boil down to metrics and the relationships between metrics, and in my experience, sitting at the intersection of data and business stakeholders, the majority of our conversations on how to move the business revolve around metrics as well for the same reason.
So metrics are just this very key lever to unlocking better decisions within the modern organization. In a world in which there are more and more applications that are talking to the data warehouse, more and more surfaces in which business stakeholders and decision makers of all different types and sizes are trying to consume data, it becomes even more necessary to have a single place where those definitions are managed and maintained consistently.
How do you think about where interoperability and integration in BI are going into the future?
BI, and analytics more generally, is moving into multiple applications. It's decentralizing from one single BI tool -- ten years ago, or even five years ago, people went to your single enterprise BI tool and you got a core set of dashboards that one team maintains and that's it -- to all these different data-driven applications: reverse ETL tools, anomaly detection tools, financial planning software, traditional BI, notebooks.
Almost every application is going to be a data-driven application in the future and is going to have to talk to the data warehouse in some way, because that's where the data lives. In that world, you have to have something that manages a common set of definitions and semantics across all those different applications for metrics.
There will not be a single BI tool that is one-size-fits-all for all the analytical needs of the organization.
Do you see a compelling reason for Snowflake or another data warehouse to build a product like this, or are there structural reasons that that wouldn’t make sense? Maybe they’re making so much money with the data warehouse that they don’t care.
I'm not an expert on data warehouses or their internals, and I obviously don't know what's going on at Snowflake, but what I perceive from the outside is that there's a lot of room to expand into supporting additional use cases on top of Snowflake. Meaning Snowflake started as an analytical database, great for exactly the types of use cases that we're talking about now, but it's not great for machine learning, and it doesn't support real time or streaming analytics as a first-class citizen yet. Some of the things that they're doing are certainly moving in that direction, so I think there's a lot of opportunity to move into that world that is for now owned by other products, in Databricks land or otherwise. To the extent that they can support those use cases, then they can enable many more applications to be built on top of Snowflake. The way they make money is through compute, so that would just help them grow their business more.
Assuming we're moving towards a world where the data warehouse is the center of gravity, do you have thoughts on whether that’s incompatible with the CDP model? Or is it more that everyone will have a data warehouse, but some teams will still use tools like Segment, Zapier, Workato, or Tray to move information around?
To be candid, I don't have too strong opinions on Zapier, Tray, and Workato. I haven't thought too much about that world.
With regard to Segment, if you're talking about their CDP product, I think the reverse ETL tools are already in some ways moving into that space. In some ways they are becoming the new CDPs. There are also other product-led growth products that sit on top of the warehouse that do a lot of the targeting that traditional CDPs have done. I think you're already seeing some of those workflows move to be built directly on top of the warehouse.
Having said that, a lot of other companies still use Segment for event tracking and sending those analytics events into a number of different destinations, including some of the tools I just mentioned. So I think they have a pretty strong hold. I still think that they're best in breed for that use case.
What’s your take on the bigger BI tools and how they might be moving towards more interoperability or unbundling? For example, Looker’s partnership with Tableau.
Looker in some ways pioneered a lot of this code-based metrics modeling when they first launched.
I don't have visibility into the internals of the Tableau-Looker partnership or what's going on there. Both of those companies were acquired in the last few years, and I imagine their parent companies have other motivations at play as well. So I wouldn't try to read too much into the tea leaves there until, or unless, they talk more about it.
Disclaimers
This transcript is for information purposes only and does not constitute advice of any type or trade recommendation and should not form the basis of any investment decision. Sacra accepts no liability for the transcript or for any errors, omissions or inaccuracies in respect of it. The views of the experts expressed in the transcript are those of the experts and they are not endorsed by, nor do they represent the opinion of Sacra. Sacra reserves all copyright, intellectual property rights in the transcript. Any modification, copying, displaying, distributing, transmitting, publishing, licensing, creating derivative works from, or selling any transcript is strictly prohibited.