- Valuation Model
- Expert Interviews
- Founders, funding
What is the history behind the development of the modern data stack?
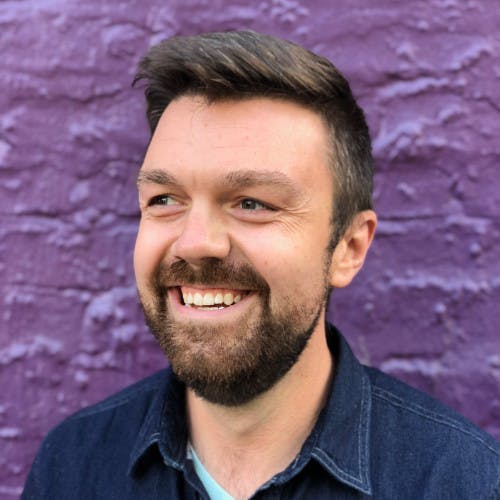
Sean Lynch
Co-founder & CPO at Census
The high-level story of Census is that we are a data integration platform. We talk about it in terms of operational analytics; other people in the industry call it "reverse ETL" -- that seems to be the accepted standard term. Both are fancy ways of saying "doing things with data," as opposed to just reading and understanding data. It's taking action on data. Our twist relative to past generations of integration platforms is that we sit as a layer on top of a data warehouse. So to understand where Census fits, it does make sense to understand the modern data stack as a concept, what that is, and then reverse ETL, or data operationalization, on top of that.
A lot of the modern data stack started with the creation of cloud data warehouses. Amazon Redshift was the big early leader. They launched in 2013; I think they did a private preview in 2012. Snowflake originally launched in 2014, so they're in a sense an overnight success ten years in the making, or a little less than that. Databricks was founded in 2013. Google claims Google BigQuery got started earlier than all of those.
Ultimately this trend around modern data stack started with the shift towards cloud data warehouses. Then you started seeing some of the other pieces that fit into that. Fivetran, for example, had been going for a couple years but pivoted into data connectors in 2014. Fishtown -- which is now Dbt Labs, the creators of DBT -- launched their first version in 2016.
Those are the component pieces when we talk about the modern data stack. They are all, frankly, relatively new. We're talking about an eight year-ish timeframe at this point. And it is an ecosystem all building around the warehouse. The warehouse is the focal point.
Over those eight years, the warehouse has gotten a lot faster. They've had competition with Databricks and Snowflake competing against Amazon and Google. They've become a lot cheaper. They've been designed to support more and more of the data use cases that, when they started, were out of their scope. When they started, they were really, "Hey, this is a cloud version of the data warehouse infrastructure that you might be installing in your data center." That's the Teradata and Netezza type of product. This was the cloud version of it, and it happened to be scalable and cheaper, but it was not necessarily changing the story in terms of use cases.
What changed is that, in addition to becoming super scalable and cheaper, they also started to shift the story of the types of data you could throw at it, what speed, over what timeframe. I like to think about the original term “Data Warehouse” as the Indiana Jones dusty old warehouse where artifacts go to die. Today, the warehouse has evolved into the Tesla manufacturing plant version, where it's shiny and new, things are moving through it at incredible speed. That's the way that the warehouse has evolved. We -- and Fivetran, dbt, and now a big ecosystem of other products around quality and discoverability, etc -- are all building on top of this very modern manufacturing pipeline, as opposed to the old storage way of thinking about warehouses. That's the core of the modern data stack.
At Census, we think about what we’re building as creating a feedback loop using that stack. As a business, you have your applications. You have something like a Fivetran that's ingesting all that data into your data warehouse -- what previously would've been called ETL and is now ELT. You have dbt doing the "T," the transform in your data warehouse. And Census comes along in the last step to provide that return path back to all those apps, to help companies actually do things with those data.