- Valuation Model
- Expert Interviews
- Founders, funding
How does Levity address data quality issues in AI modeling?
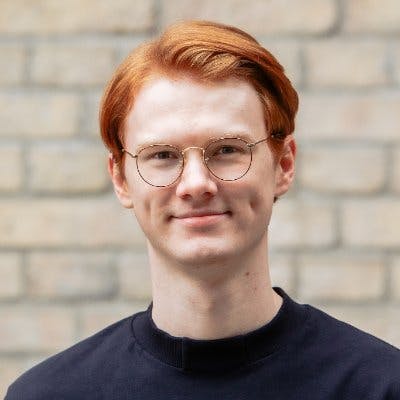
Thilo Huellmann
Co-founder & CTO at Levity
The biggest challenge in machine learning is always the data.
You can only improve your models by a few percentage points of performance by tweaking them but when it comes to data it follows the garbage-in-garbage-out paradigm. For us, there are definitely many cases where customers approach us who don’t have the data that they would need to be successful. Then, we have to be very transparent and tell them, "It might work. It might not work. You have to try it out, but we make it very easy for you to quickly conclude whether it works for you or not."
It’s a lot about expectation management, but it also really benefits if you get quick results, rather than start a machine learning project and then find out three months later that your data is insufficient and you can’t really do anything about it.
We don't do specific or manual steps with our customers. If they book a call with us, we give them a few tips to help but usually, we just have a bunch of heuristics in place to improve what's there and pre-process the data a certain way. Other than that, we deliberately don't adapt models per problem or customer.