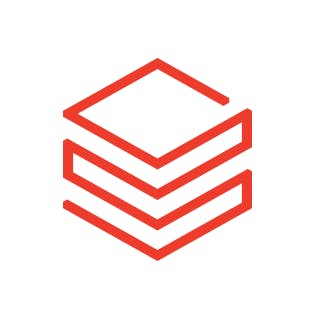
Revenue
$2.40B
2024
Valuation
$43.00B
2023
Growth Rate (y/y)
60%
2024
Funding
$4.00B
2023
Revenue
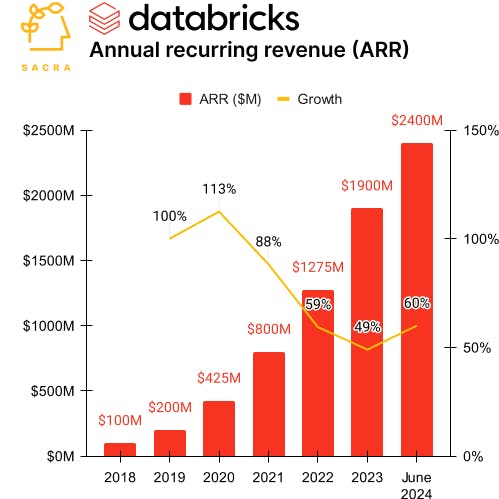
Sacra estimates that Databricks hit about $2.4B in annual recurring revenue (ARR) in June 2024, up 60% year-over-year.
Databricks reported crossing the $1B in annual recurring revenue (ARR) mark in August 2022 and bringing in $1B in total revenue for the year of 2022.
As of June 2024, Databricks has about 80% gross margins, down from 85% a year ago, while net dollar retention is at 140%.
In April 2023, their data warehousing product—Databricks SQL—hit $100M in ARR one year after launching. A year later, Databricks SQL has grown to $400M ARR.
Databricks has a pay-as-you-go model and bills customers depending on their tier, how much processing power of the software they use, and for how long. The more expensive premium and enterprise tiers offer more security, governance, higher speeds, and data processing features. Databricks works on top of Microsoft Azure, Google Cloud, and AWS, each having slightly different charges across tiers and computing power.
Databricks primarily sells to large enterprises, with some contracts reaching millions of dollars annually. As of June 2024, the company has over 11,500 customers globally. Its average contract value (ACV) has grown steadily, reaching $208,696 in June 2024.
Valuation
Databricks reached a valuation of $43 billion as of September 2023, following its latest funding round. The company has raised a total of $4.1 billion across 9 funding rounds to date. Key investors include T. Rowe Price, Andreessen Horowitz, and NVIDIA, with additional strategic investments from Microsoft Corporation and BlackRock. The company's September 2023 Series I round included participation from multiple institutional investors including Capital One Ventures and the Ontario Teachers' Pension Plan.
Product
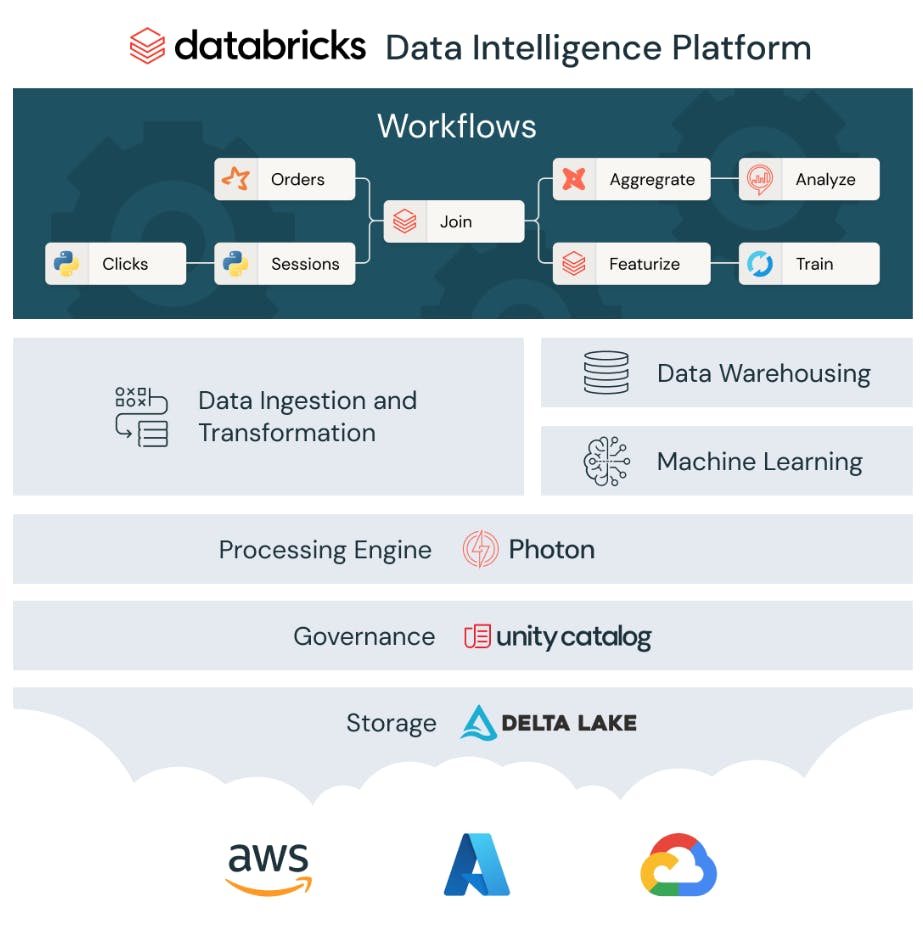
Databricks was created by the same team that made Apache Spark, open-source software for running queries on data lakes used to store large amounts of raw data cheaply. When Spark was launched in 2009, most data lakes were hosted on-premise on Hadoop, the first OS for data centers. However, running large queries on Hadoop was cumbersome and took a lot of time. Spark found its initial product market fit by making it easier and faster to run queries on top of data lakes. While it was originally designed to run on top of Hadoop, Databricks now runs on any cloud storage like AWS, Google Cloud, or Microsoft Azure.
Databricks found product-market fit by simplifying the complex data infrastructure typically required for large-scale analytics and machine learning. By providing a unified platform, Databricks reduces the need for multiple specialized tools and the associated integration challenges.
Second, the platform's collaborative features enable different roles (data engineers, data scientists, analysts) to work together more effectively.
Third, Databricks' emphasis on open standards and interoperability with popular data science tools (like Python, R, and SQL) has made it attractive to organizations with existing investments in these technologies.
Delta Lake
Delta Lake is an open-source storage layer that provides ACID transactions, scalable metadata handling, and unifies streaming and batch data processing for data lakes. Delta Lake enables organizations to build reliable data pipelines and maintain data quality at scale.
Delta Lake is the key component of Databricks’s push to move into the BI and data analytics category to compete with data warehouse companies such as SnowFlake, Amazon, and others.
Spark
Databricks’s core offering is managed Spark clusters, groups of machines used for running data analysis. It gives a web-based portal to data scientists to create these Spark clusters for running their data analysis workloads. The portal also consists of a notebook-like workspace for data scientists to collaboratively write queries in SQL, Python, etc., and a scheduler for running data pipelines on a regular schedule that data engineers can use as a replacement for Airflow or Prefect.
MosaicML
In June 2023, Databricks acquired MosaicML for $1.3B, a move aimed at bolstering its capabilities in training large language models (LLMs) and image generation models. MosaicML has developed tools and infrastructure to simplify and reduce the cost of running LLMs from data preparation to training and managing infrastructure.
The training of LLMs like GPT-3, involves significant costs, but MosaicML claims to be able to train GPT-3 quality models for its customers for as little as $325K (compare to $368K for Google LaMDA, $1M for Bloom, and $841K for GPT-3).
These prices from MosaicML also include a full suite of ML Ops tools, thus further reducing the additional personnel required to train a model reliably.
MLFlow
MLFlow is an open-source platform for managing the machine learning lifecycle. It includes capabilities for experiment tracking, model versioning, and model deployment. MLflow helps data scientists and engineers streamline the process of developing and productionizing machine learning models.
Databricks SQL
Databricks SQL is a data warehouse that lets users run SQL on top of Delta Lake, create visualizations, and build/share dashboards aimed toward data analysts in organizations that are used to running queries in SQL.
Business Model
Databricks builds open source software for data processing and AI applications and then offers a paid version with additional proprietary features, which companies cannot replicate easily on their own. While open source software gives companies flexibility of not getting locked into a proprietary architecture, most companies don’t usually have the engineering talent to manage its complexity.
This is where Databricks comes in and sells enterprises a fully managed version of its open source software, with additional utilities like SaaS tools to write queries and connectors to connect data sources. This aspect of Databricks is similar to AWS, which also provides managed services for open source software, but Databricks makes all the open source software it manages, giving it an edge over others.
Databricks started with Apache Spark for running queries on large raw datasets in data lakes. It then expanded its revenue by launching products that tapped into adjacent markets such as AI lifecycle management/MLOps (MLFlow), data warehouse (Delta Lake), data visualization (Redash), and BI and analytics (Databricks SQL).
It has both bottom-up and enterprise sales GTM. For its bottom-up sales motion, Databricks offers a free forever community edition, a small slice of the large software. Half of its leads are from community edition customers who want to use the full software or when SDRs notice heavy usage patterns and pass leads to sales teams. Databricks also offers a Twilio-like self-service model where anyone can just swipe their card and start a free trial without talking to a sales rep. Databricks also provides free training and workshops to get such users started.
When Databricks pitches to CIOs through traditional enterprise sales motion, on many occasions it is endorsed by the data scientists/engineers already using it, shortening the sales cycle. Among the bottom-up GTM software companies, Databricks has one of the highest sales headcounts to support its enterprise sales motion.
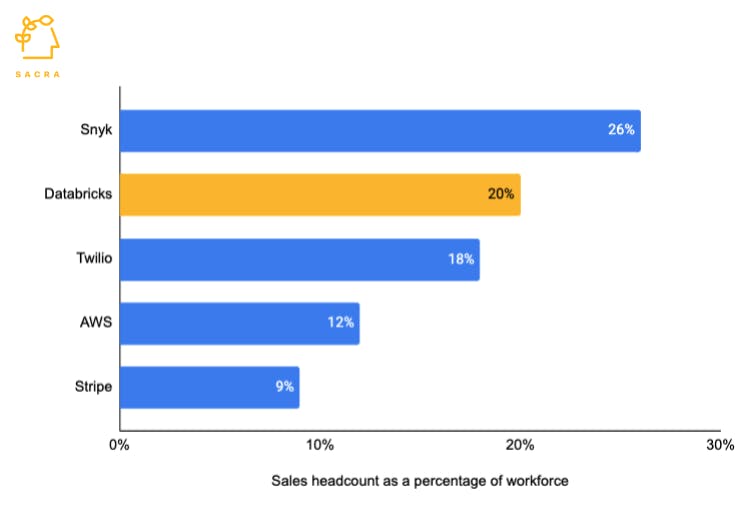
Competition
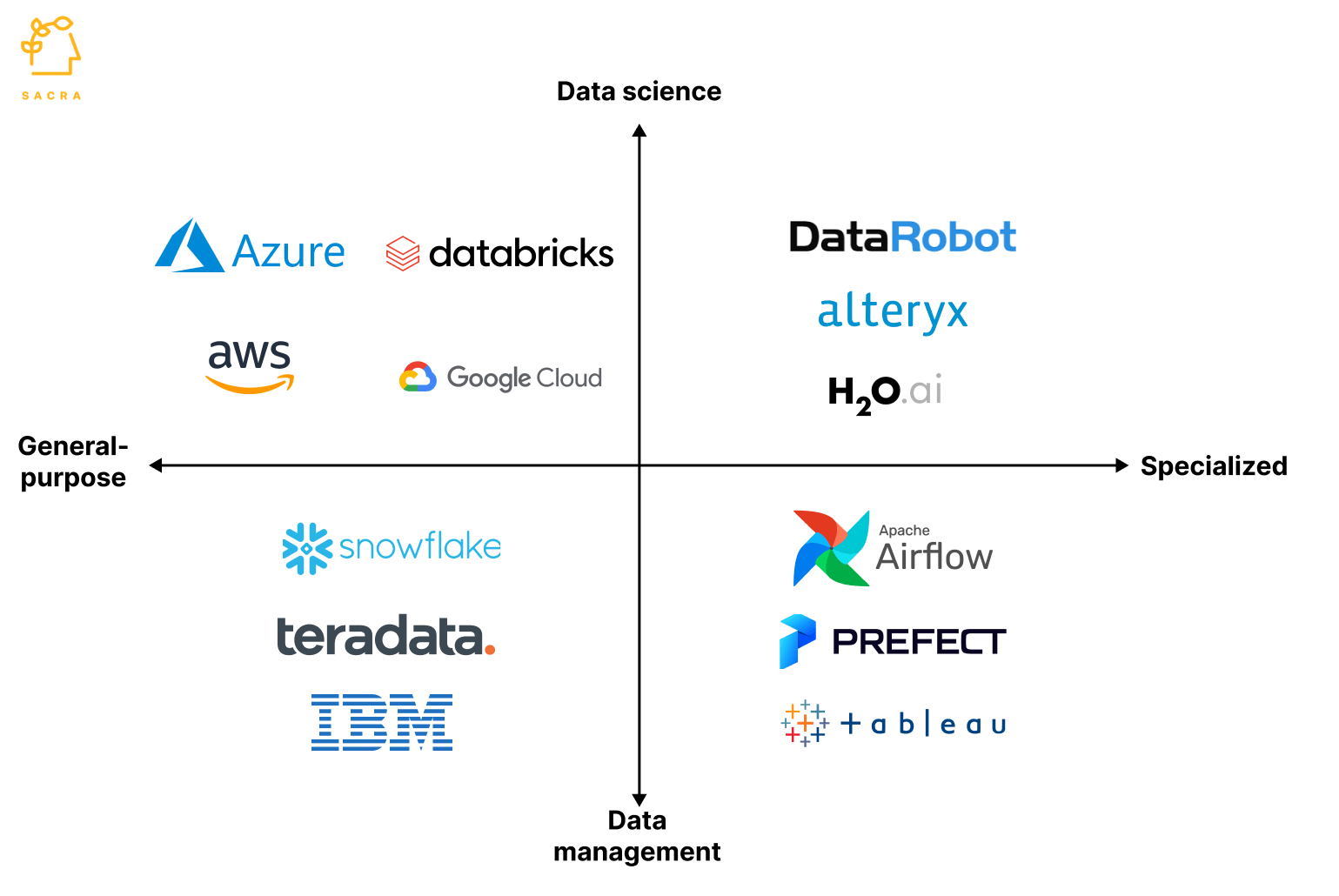
Databricks operates in the data analytics and artificial intelligence infrastructure market, competing across several key segments. The company's primary focus is on providing a unified platform for data engineering, machine learning, and business intelligence, positioning itself at the intersection of traditional data warehousing and modern AI/ML workloads.
Data Warehousing and Analytics
In the data warehousing space, Databricks' main competitor is Snowflake. Both companies aim to provide a comprehensive solution for storing, processing, and analyzing large volumes of data.
Snowflake has gained significant traction with its cloud-native data warehouse, offering seamless scalability and separation of storage and compute. Databricks, however, differentiates itself with its "lakehouse" architecture, which combines elements of data lakes and data warehouses.
The lakehouse approach allows Databricks to handle both structured and unstructured data more efficiently than traditional data warehouses. This is particularly advantageous for companies dealing with diverse data types and looking to implement machine learning models. Databricks' foundation in Apache Spark also gives it an edge in processing large-scale data and running complex analytics workloads.
Other players in this category include cloud providers like Amazon Web Services (Redshift), Google Cloud Platform (BigQuery), and Microsoft Azure (Synapse Analytics).
These companies offer integrated solutions within their respective cloud ecosystems, which can be attractive for organizations already heavily invested in a particular cloud platform. Databricks counters this by offering multi-cloud support, allowing customers to avoid vendor lock-in and leverage best-of-breed services across different cloud providers.
Machine Learning and AI Infrastructure
In the machine learning and AI infrastructure space, Databricks competes with a range of specialized platforms and cloud-based services. Key competitors include DataRobot, H2O.ai, and cloud-native offerings like AWS SageMaker and Azure Machine Learning.
Databricks distinguishes itself in this category through its integrated approach. While many competitors focus solely on model development and deployment, Databricks provides a full-stack solution that encompasses data preparation, feature engineering, model training, and deployment.
This end-to-end capability is particularly valuable for organizations looking to streamline their ML workflows and reduce the complexity of managing multiple tools and platforms.
The company's acquisition of MosaicML in 2023 for $1.3 billion further strengthened its position in the AI infrastructure market. This move expanded Databricks' capabilities in training and deploying large language models (LLMs), positioning it to compete more directly with specialized AI infrastructure providers like OpenAI and Anthropic.
Databricks' MLflow project, an open-source platform for managing the ML lifecycle, has gained significant adoption in the data science community. This has helped Databricks establish itself as a thought leader in the ML space and created a funnel for potential customers of its commercial offerings.
Data Governance and Collaboration
As organizations grapple with increasing data volumes and regulatory requirements, data governance and collaboration tools have become crucial. In this segment, Databricks competes with companies like Collibra, Alation, and Informatica.
Databricks' Unity Catalog, introduced in 2022, aims to provide a unified governance layer across all data and AI assets within an organization.
This offering integrates tightly with Databricks' core platform, providing a seamless experience for data discovery, access control, and lineage tracking. While specialized governance tools may offer more depth in certain areas, Databricks' advantage lies in its ability to provide governance capabilities natively within the data processing and analytics environment.
The company's collaborative notebooks and workspace features also compete with tools like Jupyter and Google Colab. Databricks differentiates itself by offering enterprise-grade security and scalability, making it more suitable for large-scale, production environments.
TAM Expansion
Databricks has a few clear avenues for TAM expansion both through ongoing trends and through new products and services.
AI
Databricks, through its acquisition of MosaicML, steps into a competitive arena against the likes of OpenAI by offering tools and infrastructure to companies for creating their own AI applications from scratch. Unlike OpenAI, which offers proprietary models, Databricks's approach empowers companies to harness their own data for AI model training, thus appealing to organizations keen on retaining control over their data and AI assets.
The revenue model of Databricks relies on subscriptions to its toolset and charges based on usage. The addition of MosaicML's cost-effective model training infrastructure could attract a wider customer base looking to reduce the financial barriers in training large models.
This potentially increases the subscription and usage revenue for Databricks. Moreover, the competitive pricing for training large models could become a distinctive selling point that drives additional revenue.
New partnerships with Nvidia (to optimize its software for Nvidia-powered servers) and Microsoft (to offer a version of Databricks's software through Azure) show promising movement in this direction, as well as suggest a growing ecosystem outside the walled garden of OpenAI and its proprietary models.
Databricks's positioning contra OpenAI positions Databricks favorably in a market where companies like SAP are exploring both closed-source and open-source models to avoid dependency on a single entity.
Digital transformation
COVID forced legacy companies and startups to start selling digitally. They are racing to make their personalized consumer experience as good as Amazon or TikTok by doubling down on AI.
This means pulling in data from all sorts of customer touch points like front-end clickstreams from Segment, payments from Stripe, and conversion data from Google Ads, running them through ML models, and feeding back the data streams into your website and app.
Data centralization
With cheap cloud storage and fast networks, most companies are shifting from analyzing organization data in ERP and customer data in Salesforce to putting all data in a central data store. This helps them better understand what happened (business intelligence) and what will happen (predictive analytics). Data centralization is a tailwind for Databricks as it is built on data lake technology, where you can throw in all data without worrying about the type/source of data.
Taken together, these tailwinds point towards a future where all data processing happens on a single platform. However, currently, most companies use data warehouses for running real-time business intelligence operations and data lakes for ML/data science projects. Databricks is betting that as more things go digital, the explosion of data will make it impossible for companies to run two parallel large-scale data stores, which will converge into one.
The bull case for Databricks is that it will capture the lion’s share of this future, with its Data Lakehouse offering becoming the industry standard for data centralization as Salesforce became the industry standard for customer data.
Risks
Stickiness of data warehouse: Databricks is betting on a future where companies will stop using separate software for data warehouses and instead shift to Databricks for all data processing/storage requirements. However, data warehouses are highly sticky products, like ERPs, and not easy for a large enterprise to rip out. This can make its sales cycles very long and put a cap on its serviceable market.
Funding Rounds
|
||||||
|
||||||
|
||||||
|
||||||
|
||||||
|
||||||
|
||||||
|
||||||
|
||||||
|
||||||
|
||||||
|
||||||
|
||||||
|
||||||
|
||||||
|
||||||
|
||||||
|
||||||
View the source Certificate of Incorporation copy. |
News
DISCLAIMERS
This report is for information purposes only and is not to be used or considered as an offer or the solicitation of an offer to sell or to buy or subscribe for securities or other financial instruments. Nothing in this report constitutes investment, legal, accounting or tax advice or a representation that any investment or strategy is suitable or appropriate to your individual circumstances or otherwise constitutes a personal trade recommendation to you.
This research report has been prepared solely by Sacra and should not be considered a product of any person or entity that makes such report available, if any.
Information and opinions presented in the sections of the report were obtained or derived from sources Sacra believes are reliable, but Sacra makes no representation as to their accuracy or completeness. Past performance should not be taken as an indication or guarantee of future performance, and no representation or warranty, express or implied, is made regarding future performance. Information, opinions and estimates contained in this report reflect a determination at its original date of publication by Sacra and are subject to change without notice.
Sacra accepts no liability for loss arising from the use of the material presented in this report, except that this exclusion of liability does not apply to the extent that liability arises under specific statutes or regulations applicable to Sacra. Sacra may have issued, and may in the future issue, other reports that are inconsistent with, and reach different conclusions from, the information presented in this report. Those reports reflect different assumptions, views and analytical methods of the analysts who prepared them and Sacra is under no obligation to ensure that such other reports are brought to the attention of any recipient of this report.
All rights reserved. All material presented in this report, unless specifically indicated otherwise is under copyright to Sacra. Sacra reserves any and all intellectual property rights in the report. All trademarks, service marks and logos used in this report are trademarks or service marks or registered trademarks or service marks of Sacra. Any modification, copying, displaying, distributing, transmitting, publishing, licensing, creating derivative works from, or selling any report is strictly prohibited. None of the material, nor its content, nor any copy of it, may be altered in any way, transmitted to, copied or distributed to any other party, without the prior express written permission of Sacra. Any unauthorized duplication, redistribution or disclosure of this report will result in prosecution.